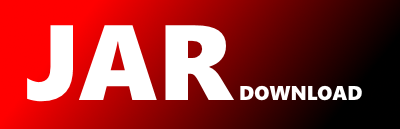
moa.classifiers.meta.AccuracyUpdatedEnsemble Maven / Gradle / Ivy
Go to download
Show more of this group Show more artifacts with this name
Show all versions of moa Show documentation
Show all versions of moa Show documentation
Massive On-line Analysis is an environment for massive data mining. MOA
provides a framework for data stream mining and includes tools for evaluation
and a collection of machine learning algorithms. Related to the WEKA project,
also written in Java, while scaling to more demanding problems.
/*
* AccuracyUpdatedEnsemble.java
* Copyright (C) 2010 Poznan University of Technology, Poznan, Poland
* @author Dariusz Brzezinski ([email protected])
*
* This program is free software; you can redistribute it and/or modify
* it under the terms of the GNU General Public License as published by
* the Free Software Foundation; either version 2 of the License, or
* (at your option) any later version.
*
* This program is distributed in the hope that it will be useful,
* but WITHOUT ANY WARRANTY; without even the implied warranty of
* MERCHANTABILITY or FITNESS FOR A PARTICULAR PURPOSE. See the
* GNU General Public License for more details.
*
* You should have received a copy of the GNU General Public License
* along with this program; if not, write to the Free Software
* Foundation, Inc., 675 Mass Ave, Cambridge, MA 02139, USA.
*/
package moa.classifiers.meta;
import moa.classifiers.AbstractClassifier;
import moa.classifiers.Classifier;
import moa.classifiers.MultiClassClassifier;
import moa.classifiers.trees.HoeffdingTree;
import moa.core.DoubleVector;
import moa.core.Measurement;
import moa.core.ObjectRepository;
import moa.options.ClassOption;
import com.github.javacliparser.IntOption;
import moa.tasks.TaskMonitor;
import com.yahoo.labs.samoa.instances.Instance;
import com.yahoo.labs.samoa.instances.Instances;
/**
* The revised version of the Accuracy Updated Ensemble as proposed by
* Brzezinski and Stefanowski in "Reacting to Different Types of Concept Drift:
* The Accuracy Updated Ensemble Algorithm", IEEE Trans. Neural Netw, 2013.
*/
public class AccuracyUpdatedEnsemble extends AbstractClassifier implements MultiClassClassifier {
private static final long serialVersionUID = 1L;
/**
* Type of classifier to use as a component classifier.
*/
public ClassOption learnerOption = new ClassOption("learner", 'l', "Classifier to train.", Classifier.class,
"trees.HoeffdingTree -e 2000000 -g 100 -c 0.01");
/**
* Number of component classifiers.
*/
public IntOption memberCountOption = new IntOption("memberCount", 'n',
"The maximum number of classifiers in an ensemble.", 10, 1, Integer.MAX_VALUE);
/**
* Chunk size.
*/
public IntOption chunkSizeOption = new IntOption("chunkSize", 'c',
"The chunk size used for classifier creation and evaluation.", 500, 1, Integer.MAX_VALUE);
/**
* Determines the maximum size of model (evaluated after every chunk).
*/
public IntOption maxByteSizeOption = new IntOption("maxByteSize", 'm', "Maximum memory consumed by ensemble.",
33554432, 0, Integer.MAX_VALUE);
/**
* The weights of stored classifiers.
* weights[x][0] = weight
* weights[x][1] = classifier number in learners
*/
protected double[][] weights;
/**
* Class distributions.
*/
protected long[] classDistributions;
/**
* Ensemble classifiers.
*/
protected Classifier[] learners;
/**
* Number of processed examples.
*/
protected int processedInstances;
/**
* Candidate classifier.
*/
protected Classifier candidate;
/**
* Current chunk of instances.
*/
protected Instances currentChunk;
@Override
public void prepareForUseImpl(TaskMonitor monitor, ObjectRepository repository) {
this.candidate = (Classifier) getPreparedClassOption(this.learnerOption);
this.candidate.resetLearning();
super.prepareForUseImpl(monitor, repository);
}
@Override
public void resetLearningImpl() {
this.currentChunk = null;
this.classDistributions = null;
this.processedInstances = 0;
this.learners = new Classifier[0];
this.candidate = (Classifier) getPreparedClassOption(this.learnerOption);
this.candidate.resetLearning();
}
@Override
public void trainOnInstanceImpl(Instance inst) {
this.initVariables();
this.classDistributions[(int) inst.classValue()]++;
this.currentChunk.add(inst);
this.processedInstances++;
if (this.processedInstances % this.chunkSizeOption.getValue() == 0) {
this.processChunk();
}
}
/**
* Determines whether the classifier is randomizable.
*/
public boolean isRandomizable() {
return false;
}
/**
* Predicts a class for an example.
*/
public double[] getVotesForInstance(Instance inst) {
DoubleVector combinedVote = new DoubleVector();
if (this.trainingWeightSeenByModel > 0.0) {
for (int i = 0; i < this.learners.length; i++) {
if (this.weights[i][0] > 0.0) {
DoubleVector vote = new DoubleVector(this.learners[(int) this.weights[i][1]].getVotesForInstance(inst));
if (vote.sumOfValues() > 0.0) {
vote.normalize();
// scale weight and prevent overflow
vote.scaleValues(this.weights[i][0] / (1.0 * this.learners.length + 1.0));
combinedVote.addValues(vote);
}
}
}
}
//combinedVote.normalize();
return combinedVote.getArrayRef();
}
@Override
public void getModelDescription(StringBuilder out, int indent) {
}
@Override
public Classifier[] getSubClassifiers() {
return this.learners.clone();
}
/**
* Processes a chunk of instances.
* This method is called after collecting a chunk of examples.
*/
protected void processChunk() {
Classifier addedClassifier = null;
double mse_r = this.computeMseR();
// Compute weights
double candidateClassifierWeight = 1.0 / (mse_r + Double.MIN_VALUE);
for (int i = 0; i < this.learners.length; i++) {
this.weights[i][0] = 1.0 / (mse_r + this.computeMse(this.learners[(int) this.weights[i][1]], this.currentChunk) + Double.MIN_VALUE);
}
if (this.learners.length < this.memberCountOption.getValue()) {
// Train and add classifier
addedClassifier = this.addToStored(this.candidate, candidateClassifierWeight);
} else {
// Substitute poorest classifier
int poorestClassifier = this.getPoorestClassifierIndex();
if (this.weights[poorestClassifier][0] < candidateClassifierWeight) {
this.weights[poorestClassifier][0] = candidateClassifierWeight;
addedClassifier = this.candidate.copy();
this.learners[(int) this.weights[poorestClassifier][1]] = addedClassifier;
}
}
// train classifiers
for (int i = 0; i < this.learners.length; i++) {
this.trainOnChunk(this.learners[(int) this.weights[i][1]]);
}
this.classDistributions = null;
this.currentChunk = null;
this.candidate = (Classifier) getPreparedClassOption(this.learnerOption);
this.candidate.resetLearning();
this.enforceMemoryLimit();
}
/**
* Checks if the memory limit is exceeded and if so prunes the classifiers in the ensemble.
*/
protected void enforceMemoryLimit() {
double memoryLimit = this.maxByteSizeOption.getValue() / (double) (this.learners.length + 1);
for (int i = 0; i < this.learners.length; i++) {
((HoeffdingTree) this.learners[(int) this.weights[i][1]]).maxByteSizeOption.setValue((int) Math
.round(memoryLimit));
((HoeffdingTree) this.learners[(int) this.weights[i][1]]).enforceTrackerLimit();
}
}
/**
* Computes the MSEr threshold.
*
* @return The MSEr threshold.
*/
protected double computeMseR() {
double p_c;
double mse_r = 0;
for (int i = 0; i < this.classDistributions.length; i++) {
p_c = (double) this.classDistributions[i] / (double) this.chunkSizeOption.getValue();
mse_r += p_c * ((1 - p_c) * (1 - p_c));
}
return mse_r;
}
/**
* Computes the MSE of a learner for a given chunk of examples.
* @param learner classifier to compute error
* @param chunk chunk of examples
* @return the computed error.
*/
protected double computeMse(Classifier learner, Instances chunk) {
double mse_i = 0;
double f_ci;
double voteSum;
for (int i = 0; i < chunk.numInstances(); i++) {
try {
voteSum = 0;
for (double element : learner.getVotesForInstance(chunk.instance(i))) {
voteSum += element;
}
if (voteSum > 0) {
f_ci = learner.getVotesForInstance(chunk.instance(i))[(int) chunk.instance(i).classValue()]
/ voteSum;
mse_i += (1 - f_ci) * (1 - f_ci);
} else {
mse_i += 1;
}
} catch (Exception e) {
mse_i += 1;
}
}
mse_i /= this.chunkSizeOption.getValue();
return mse_i;
}
/**
* Adds ensemble weights to the measurements.
*/
@Override
protected Measurement[] getModelMeasurementsImpl() {
Measurement[] measurements = new Measurement[(int) this.memberCountOption.getValue()];
for (int m = 0; m < this.memberCountOption.getValue(); m++) {
measurements[m] = new Measurement("Member weight " + (m + 1), -1);
}
if (this.weights != null) {
for (int i = 0; i < this.weights.length; i++) {
measurements[i] = new Measurement("Member weight " + (i + 1), this.weights[i][0]);
}
}
return measurements;
}
/**
* Adds a classifier to the storage.
*
* @param newClassifier
* The classifier to add.
* @param newClassifiersWeight
* The new classifiers weight.
*/
protected Classifier addToStored(Classifier newClassifier, double newClassifiersWeight) {
Classifier addedClassifier = null;
Classifier[] newStored = new Classifier[this.learners.length + 1];
double[][] newStoredWeights = new double[newStored.length][2];
for (int i = 0; i < newStored.length; i++) {
if (i < this.learners.length) {
newStored[i] = this.learners[i];
newStoredWeights[i][0] = this.weights[i][0];
newStoredWeights[i][1] = this.weights[i][1];
} else {
newStored[i] = addedClassifier = newClassifier.copy();
newStoredWeights[i][0] = newClassifiersWeight;
newStoredWeights[i][1] = i;
}
}
this.learners = newStored;
this.weights = newStoredWeights;
return addedClassifier;
}
/**
* Finds the index of the classifier with the smallest weight.
* @return
*/
private int getPoorestClassifierIndex() {
int minIndex = 0;
for (int i = 1; i < this.weights.length; i++) {
if(this.weights[i][0] < this.weights[minIndex][0]){
minIndex = i;
}
}
return minIndex;
}
/**
* Initiates the current chunk and class distribution variables.
*/
private void initVariables() {
if (this.currentChunk == null) {
this.currentChunk = new Instances(this.getModelContext());
}
if (this.classDistributions == null) {
this.classDistributions = new long[this.getModelContext().classAttribute().numValues()];
for (int i = 0; i < this.classDistributions.length; i++) {
this.classDistributions[i] = 0;
}
}
}
/**
* Trains a component classifier on the most recent chunk of data.
*
* @param classifierToTrain
* Classifier being trained.
*/
private void trainOnChunk(Classifier classifierToTrain) {
for (int num = 0; num < this.chunkSizeOption.getValue(); num++) {
classifierToTrain.trainOnInstance(this.currentChunk.instance(num));
}
}
}
© 2015 - 2025 Weber Informatics LLC | Privacy Policy