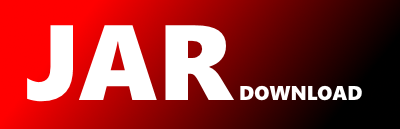
moa.classifiers.meta.RCD Maven / Gradle / Ivy
Go to download
Show more of this group Show more artifacts with this name
Show all versions of moa Show documentation
Show all versions of moa Show documentation
Massive On-line Analysis is an environment for massive data mining. MOA
provides a framework for data stream mining and includes tools for evaluation
and a collection of machine learning algorithms. Related to the WEKA project,
also written in Java, while scaling to more demanding problems.
/*
* RCD.java
* Copyright (C) 2017 Instituto Federal de Pernambuco
* @author Paulo Gonçalves ([email protected])
*
* This program is free software; you can redistribute it and/or modify
* it under the terms of the GNU General Public License as published by
* the Free Software Foundation; either version 3 of the License, or
* (at your option) any later version.
*
* This program is distributed in the hope that it will be useful,
* but WITHOUT ANY WARRANTY; without even the implied warranty of
* MERCHANTABILITY or FITNESS FOR A PARTICULAR PURPOSE. See the
* GNU General Public License for more details.
*
* You should have received a copy of the GNU General Public License
* along with this program. If not, see .
*
*/
package moa.classifiers.meta;
import com.github.javacliparser.FloatOption;
import com.github.javacliparser.IntOption;
import com.yahoo.labs.samoa.instances.Instance;
import java.io.Serializable;
import java.util.ArrayList;
import java.util.HashMap;
import java.util.List;
import java.util.Map;
import java.util.concurrent.ExecutionException;
import java.util.concurrent.ExecutorService;
import java.util.concurrent.Executors;
import java.util.concurrent.Future;
import moa.classifiers.Classifier;
import moa.classifiers.core.statisticaltests.StatisticalTest;
import moa.classifiers.drift.SingleClassifierDrift;
import moa.core.MiscUtils;
import moa.options.ClassOption;
/**
* Creates a set of classifiers, each one representing a different context.
* Reuses classifier associating to each one a sample of data and compares new
* data to old ones using a multivariate non-parametric statistical test. Tests
* are performed in parallel and classifiers are stored based on their accuracy
* and stored time.
*
* 1) Parameterized number of classifiers to store. 2) Classifiers are stored
* removing the older ones if the set is full. 3) Classifier with higher
* significance value is selected.
*
* Based on: Gonçalves Jr, Paulo Mauricio, and Roberto Souto Maior De Barros.
* "RCD: A recurring concept drift framework." Pattern Recognition Letters 34.9
* (2013): 1018-1025.
*
* @author Paulo Goncalves (paulogoncalves at recife dot ifpe dot edu dot br)
*
*/
public class RCD extends SingleClassifierDrift {
private static final long serialVersionUID = 1L;
private class ClassifierKS implements Serializable {
private final Classifier classifier;
private final List instances;
public ClassifierKS(Classifier classifier, List instances) {
this.classifier = classifier;
this.instances = instances;
}
public Classifier getClassifier() {
return classifier;
}
public List getInstances() {
return instances;
}
}
public ClassOption statisticalTestOption = new ClassOption("statisticalTest",
'a', "Non-parametric multivariate statistical test to use.", StatisticalTest.class,
"KNN");
public FloatOption similarityBetweenDistributionsOption = new FloatOption(
"similarityBetweenDistributions",
's',
"The minimum percentual similarity between distributions (p-value).",
0.01, 0, 1);
public IntOption bufferSizeOption = new IntOption("bufferSize", 'b',
"The size of the buffer that represents the distributions.", 400,
1, Integer.MAX_VALUE);
public IntOption testFrequencyOption = new IntOption("testFrequency",
't', "In the testing phase, test for best stored classifier after how many instances.",
400, 1, Integer.MAX_VALUE);
public IntOption classifiersSizeOption = new IntOption("classifiersSize",
'c', "The maximum amount of classifiers to store. 0 means unlimited.", 15, 0,
Integer.MAX_VALUE);
public IntOption threadSizeOption = new IntOption("threadSize",
'm', "The thread pool size, indicating how many simultaneous tests are allowed.", 4, 1,
Integer.MAX_VALUE);
public IntOption quantityClassifiersTestOption = new IntOption("quantityClassifiersTest",
'q', "Quantity of identified classifiers to check.", 1, 1,
Integer.MAX_VALUE);
private List classifiers;
protected List currentChunk;
protected List currentChunk2;
protected List testChunk;
protected int bufferSize;
protected int previousState;
protected int index;
@Override
public void resetLearningImpl() {
super.resetLearningImpl();
this.classifiers = new ArrayList();
this.bufferSize = bufferSizeOption.getValue();
this.currentChunk = null;
this.currentChunk2 = null;
this.testChunk = null;
this.previousState = Integer.MIN_VALUE;
this.index = 0;
}
@Override
public void trainOnInstanceImpl(Instance inst) {
int trueClass = (int) inst.classValue();
boolean prediction = MiscUtils.maxIndex(this.classifier
.getVotesForInstance(inst)) == trueClass;
this.driftDetectionMethod.input(prediction ? 0.0 : 1.0);
this.ddmLevel = DDM_INCONTROL_LEVEL;
if (this.driftDetectionMethod.getChange()) {
this.ddmLevel = DDM_OUTCONTROL_LEVEL;
}
if (this.driftDetectionMethod.getWarningZone()) {
this.ddmLevel = DDM_WARNING_LEVEL;
}
switch (this.ddmLevel) {
case DDM_WARNING_LEVEL:
this.warningDetected++;
switch (this.previousState) {
case DDM_INCONTROL_LEVEL:
this.newclassifier.resetLearning();
this.currentChunk2 = new ArrayList();
break;
}
this.newclassifier.trainOnInstance(inst);
this.addInstance(this.currentChunk2, inst);
this.previousState = DDM_WARNING_LEVEL;
break;
case DDM_OUTCONTROL_LEVEL:
this.changeDetected++;
switch (this.previousState) {
case DDM_WARNING_LEVEL:
ClassifierKS cs = this.getPreviousClassifier(
this.classifier, this.currentChunk2);
if (cs == null) {
this.classifier = this.newclassifier;
this.newclassifier = ((Classifier) getPreparedClassOption(this.baseLearnerOption))
.copy();
this.classifiers
.add(new ClassifierKS(
this.classifier, this.currentChunk2));
this.currentChunk = this.currentChunk2;
int maxSize = this.classifiersSizeOption.getValue();
if (this.classifiers.size() > maxSize && maxSize > 0) {
this.classifiers.remove(0);
}
} else {
this.classifier = cs.getClassifier();
this.currentChunk = cs.getInstances();
}
this.currentChunk2 = null;
this.newclassifier.resetLearning();
}
this.previousState = DDM_OUTCONTROL_LEVEL;
break;
case DDM_INCONTROL_LEVEL:
switch (this.previousState) {
case DDM_INCONTROL_LEVEL:
case DDM_OUTCONTROL_LEVEL:
break;
case DDM_WARNING_LEVEL:
this.currentChunk2 = null;
break;
default:
this.currentChunk = new ArrayList();
this.classifiers.add(new ClassifierKS(
this.classifier, this.currentChunk));
break;
}
this.addInstance(this.currentChunk, inst);
this.previousState = DDM_INCONTROL_LEVEL;
break;
}
this.classifier.trainOnInstance(inst);
}
private void addInstance(List instances, Instance instance) {
if (instances.size() >= bufferSize) {
instances.remove(0);
}
instances.add(instance);
}
@Override
public double[] getVotesForInstance(Instance inst) {
if (this.testChunk == null) {
this.testChunk = new ArrayList();
}
this.addInstance(this.testChunk, inst);
if (this.index++ == testFrequencyOption.getValue()) {
this.index = 0;
ClassifierKS cs = this.getPreviousClassifier(
this.classifier, this.testChunk);
if (cs != null) {
this.classifier = cs.getClassifier();
}
}
return this.classifier.getVotesForInstance(inst);
}
/**
* Searches for the classifier best suited for actual data. All statistical
* tests are performed in parallel.
*
* @param classifier Classifier to be added
* @param instances Instances used to build the classifier
* @return
*/
private ClassifierKS getPreviousClassifier(Classifier classifier,
List instances) {
ExecutorService threadPool = Executors.newFixedThreadPool(this.threadSizeOption.getValue());
int SIZE = this.classifiers.size();
Map> futures = new HashMap<>();
for (int i = 0; i < SIZE; i++) {
ClassifierKS cs = this.classifiers.get(i);
if (cs != null) {
if (cs.getClassifier() != classifier) {
StatisticalTest st = (StatisticalTest) getPreparedClassOption(this.statisticalTestOption);
StatisticalTest temp = (StatisticalTest) st.copy();
temp.set(instances, cs.getInstances());
futures.put(i, threadPool.submit(temp));
}
} else {
break;
}
}
ClassifierKS cks = null;
int qtd = this.quantityClassifiersTestOption.getValue();
double maxPValue = this.similarityBetweenDistributionsOption.getValue();
try {
for (int i = 0; i < SIZE && qtd > 0; i++) {
Future f = futures.get(i);
if (f != null) {
double p = f.get();
if (p < maxPValue) {
maxPValue = p;
cks = this.classifiers.get(i);
qtd--;
}
}
}
} catch (InterruptedException e) {
System.out.println("Processing interrupted.");
} catch (ExecutionException e) {
throw new RuntimeException("Error computing statistical test.", e);
}
threadPool.shutdownNow();
return cks;
}
}
© 2015 - 2025 Weber Informatics LLC | Privacy Policy