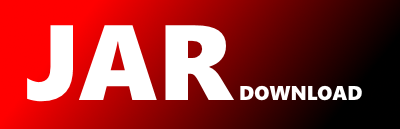
moa.classifiers.rules.multilabel.core.LearningLiteralRegression Maven / Gradle / Ivy
Go to download
Show more of this group Show more artifacts with this name
Show all versions of moa Show documentation
Show all versions of moa Show documentation
Massive On-line Analysis is an environment for massive data mining. MOA
provides a framework for data stream mining and includes tools for evaluation
and a collection of machine learning algorithms. Related to the WEKA project,
also written in Java, while scaling to more demanding problems.
package moa.classifiers.rules.multilabel.core;
import java.util.Arrays;
import java.util.LinkedList;
import java.util.List;
import moa.classifiers.MultiLabelLearner;
import moa.classifiers.rules.core.NumericRulePredicate;
import moa.classifiers.rules.core.Utils;
import moa.classifiers.rules.multilabel.attributeclassobservers.AttributeStatisticsObserver;
import moa.classifiers.rules.multilabel.attributeclassobservers.NominalStatisticsObserver;
import moa.classifiers.rules.multilabel.attributeclassobservers.NumericStatisticsObserver;
import moa.classifiers.rules.multilabel.core.splitcriteria.MultiLabelSplitCriterion;
import moa.classifiers.rules.multilabel.functions.AMRulesFunction;
import moa.classifiers.rules.multilabel.instancetransformers.InstanceOutputAttributesSelector;
import moa.classifiers.rules.multilabel.instancetransformers.InstanceTransformer;
import moa.core.AutoExpandVector;
import moa.core.DoubleVector;
import moa.core.ObjectRepository;
import moa.tasks.TaskMonitor;
import com.yahoo.labs.samoa.instances.Instance;
import com.yahoo.labs.samoa.instances.InstanceInformation;
import com.yahoo.labs.samoa.instances.InstancesHeader;
import com.yahoo.labs.samoa.instances.MultiLabelInstance;
import com.yahoo.labs.samoa.instances.Prediction;
public class LearningLiteralRegression extends LearningLiteral {
/**
*
*/
private static final long serialVersionUID = 1L;
double [] varianceShift; //for proper computation of variance
public LearningLiteralRegression() {
super();
}
public LearningLiteralRegression(int [] outputsToLearn) {
super(outputsToLearn);
}
protected double [] getNormalizedErrors(Prediction prediction, Instance instance) {
double [] errors= new double[outputsToLearn.length];
for (int i=0; i 0.0000001) {
normalizedY = (value - meanY) / (sdY);
}
return normalizedY;
}
@Override
protected void prepareForUseImpl(TaskMonitor monitor,
ObjectRepository repository) {
}
@Override
public boolean tryToExpand(double splitConfidence, double tieThreshold) {
boolean shouldSplit=false;
//find the best split per attribute and rank the results
AttributeExpansionSuggestion[] bestSplitSuggestions = this.getBestSplitSuggestions(splitCriterion);
double sumMerit=0;
meritPerInput= new double[attributesMask.length];
for (int i=0; i0){
meritPerInput[bestSplitSuggestions[i].predicate.getAttributeIndex()]=merit;
sumMerit+=merit;
}
}
//if merit==0 it means the split have not enough examples in the smallest branch
if(sumMerit==0)
meritPerInput=null; //this indicates that no merit should be considered (e.g. for feature ranking)
Arrays.sort(bestSplitSuggestions);
//disable attributes that are not relevant
int []oldInputs=inputsToLearn.clone();
inputsToLearn=inputSelector.getNextInputIndices(bestSplitSuggestions); //
Arrays.sort(this.inputsToLearn);
for (int i=0; i 0) && (bestSplitSuggestions[0].merit > 0));
bestSuggestion = bestSplitSuggestions[bestSplitSuggestions.length - 1];
shouldSplit = true;
} // Otherwise, consider which of the splits proposed may be worth trying
else {
double hoeffdingBound = computeHoeffdingBound(splitCriterion.getRangeOfMerit(this.literalStatistics), splitConfidence, weightSeen);
//debug("Hoeffding bound " + hoeffdingBound, 4);
// Determine the top two ranked splitting suggestions
bestSuggestion = bestSplitSuggestions[bestSplitSuggestions.length - 1];
AttributeExpansionSuggestion secondBestSuggestion
= bestSplitSuggestions[bestSplitSuggestions.length - 2];
if ((((bestSuggestion.merit-secondBestSuggestion.merit)) > hoeffdingBound) || (hoeffdingBound < tieThreshold)) {
//if ((((secondBestSuggestion.merit/bestSuggestion.merit) + hoeffdingBound) < 1) || (hoeffdingBound < tieThreshold)) {
//debug("Expanded ", 5);
shouldSplit = true;
//System.out.println(bestSuggestion.merit);
}
}
if(shouldSplit)
{
//check which branch is better and update bestSuggestion (in amrules the splits are binary )
DoubleVector[][] resultingStatistics=bestSuggestion.getResultingNodeStatistics();
//if not or higher is better, change predicate (negate condition)
double [] branchMerits=splitCriterion.getBranchesSplitMerits(resultingStatistics);
DoubleVector[] newLiteralStatistics;
if(branchMerits[1]>branchMerits[0]){
bestSuggestion.getPredicate().negateCondition();
newLiteralStatistics=getBranchStatistics(resultingStatistics,1);
}else{
newLiteralStatistics=getBranchStatistics(resultingStatistics,0);
}
//
int [] newOutputs=outputSelector.getNextOutputIndices(newLiteralStatistics,literalStatistics, outputsToLearn);
Arrays.sort(newOutputs); //Must be ordered for latter correspondence algorithm to work
//set other branch (only used if default rule expands)
otherBranchLearningLiteral=new LearningLiteralRegression();
otherBranchLearningLiteral.instanceHeader=instanceHeader;
otherBranchLearningLiteral.learner=(MultiLabelLearner)learner.copy();
otherBranchLearningLiteral.instanceTransformer=(InstanceTransformer)this.instanceTransformer;
//keep a rule learning to the complement set of newOutputs
//Set expanding branch
//if is AMRulesFunction and the number of output attributes changes, start learning a new predictor
//should we do the same for input attributes (attributesMask)?. It would have impact in RandomAMRules
if(learner instanceof AMRulesFunction){ //Reset learning
if(newOutputs.length != outputsToLearn.length){
//other outputs
int [] otherOutputs=Utils.complementSet(outputsToLearn,newOutputs);
int [] indices;
if(otherOutputs.length>0){
otherOutputsLearningLiteral=new LearningLiteralRegression(otherOutputs);
MultiLabelLearner otherOutputsLearner=(MultiLabelLearner)learner.copy();
indices=Utils.getIndexCorrespondence(outputsToLearn,otherOutputs);
((AMRulesFunction) otherOutputsLearner).selectOutputsToLearn(indices);
((AMRulesFunction) otherOutputsLearner).resetWithMemory();
otherOutputsLearningLiteral.learner=otherOutputsLearner;
otherOutputsLearningLiteral.instanceHeader=instanceHeader;
otherOutputsLearningLiteral.instanceTransformer=new InstanceOutputAttributesSelector(instanceHeader,otherOutputs);
}
//expanded
indices=Utils.getIndexCorrespondence(outputsToLearn,newOutputs);
((AMRulesFunction) learner).selectOutputsToLearn(indices);
}
((AMRulesFunction) learner).resetWithMemory();
}
//just reset learning
else{
//other outputs //TODO JD: Test for general learner (other than AMRules functions
if(newOutputs.length != outputsToLearn.length){
int [] otherOutputs=Utils.complementSet(outputsToLearn,newOutputs);
if(otherOutputs.length>0){
otherOutputsLearningLiteral=new LearningLiteralRegression();
MultiLabelLearner otherOutputsLearner=(MultiLabelLearner)learner.copy();
otherOutputsLearner.resetLearning();
otherOutputsLearningLiteral.learner=otherOutputsLearner;
otherOutputsLearningLiteral.instanceHeader=instanceHeader;
otherOutputsLearningLiteral.instanceTransformer=new InstanceOutputAttributesSelector(instanceHeader,otherOutputs);
}
}
//expanded
learner.resetLearning();
}
expandedLearningLiteral=new LearningLiteralRegression(newOutputs);
expandedLearningLiteral.learner=(MultiLabelLearner)this.learner.copy();
expandedLearningLiteral.instanceHeader=instanceHeader;
expandedLearningLiteral.instanceTransformer=new InstanceOutputAttributesSelector(instanceHeader,newOutputs);
}
return shouldSplit;
}
private DoubleVector[] getBranchStatistics(DoubleVector[][] resultingStatistics, int indexBranch) {
DoubleVector[] selBranchStats=new DoubleVector[resultingStatistics.length];
for(int i=0; i bestSuggestions = new LinkedList();
for (int i = 0; i < this.inputsToLearn.length; i++) {
if(attributesMask[inputsToLearn[i]]){ //Should always be true (check trainOnInstance(). Remove?
AttributeStatisticsObserver obs = this.attributeObservers.get(inputsToLearn[i]);
if (obs != null) {
AttributeExpansionSuggestion bestSuggestion = obs.getBestEvaluatedSplitSuggestion(criterion, literalStatistics, inputsToLearn[i]);
if (bestSuggestion == null) {
//ALL attributes must have a best suggestion. Adding dummy suggestion with minimal merit.
bestSuggestion=new AttributeExpansionSuggestion(new NumericRulePredicate(inputsToLearn[i],0,true),null,-Double.MAX_VALUE);
}
bestSuggestions.add(bestSuggestion);
}
}
}
return bestSuggestions.toArray(new AttributeExpansionSuggestion[bestSuggestions.size()]);
}
@Override
public void trainOnInstance(MultiLabelInstance instance) {
int numInputs=0;
if (attributesMask==null)
numInputs=initializeAttibutesMask(instance);
//learn for all output attributes if not specified at construction time
int numOutputs=instance.numberOutputTargets();
if(!hasStarted)
{
if(this.learner.isRandomizable())
this.learner.setRandomSeed(this.randomGenerator.nextInt());
if(outputsToLearn==null)
{
outputsToLearn=new int[numOutputs];
for (int i=0; i();
for(int i=0; i
© 2015 - 2025 Weber Informatics LLC | Privacy Policy