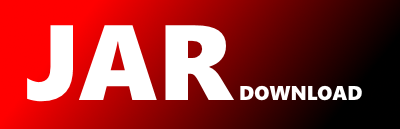
moa.cluster.Cluster Maven / Gradle / Ivy
Go to download
Massive On-line Analysis is an environment for massive data mining. MOA
provides a framework for data stream mining and includes tools for evaluation
and a collection of machine learning algorithms. Related to the WEKA project,
also written in Java, while scaling to more demanding problems.
/*
* Cluster.java
* Copyright (C) 2010 RWTH Aachen University, Germany
* @author Jansen ([email protected])
*
* Licensed under the Apache License, Version 2.0 (the "License");
* you may not use this file except in compliance with the License.
* You may obtain a copy of the License at
*
* http://www.apache.org/licenses/LICENSE-2.0
*
* Unless required by applicable law or agreed to in writing, software
* distributed under the License is distributed on an "AS IS" BASIS,
* WITHOUT WARRANTIES OR CONDITIONS OF ANY KIND, either express or implied.
* See the License for the specific language governing permissions and
* limitations under the License.
*
*
*/
package moa.cluster;
import java.util.ArrayList;
import java.util.HashMap;
import java.util.Iterator;
import java.util.Map;
import java.util.Random;
import moa.AbstractMOAObject;
import com.yahoo.labs.samoa.instances.Instance;
public abstract class Cluster extends AbstractMOAObject {
private static final long serialVersionUID = 1L;
private double id = -1;
private double gtLabel = -1;
private HashMap measure_values;
public Cluster(){
this.measure_values = new HashMap();
}
/**
* @return the center of the cluster
*/
public abstract double[] getCenter();
/**
* Returns the weight of this cluster, not neccessarily normalized.
* It could, for instance, simply return the number of points contined
* in this cluster.
* @return the weight
*/
public abstract double getWeight();
/**
* Returns the probability of the given point belonging to
* this cluster.
*
* @param point
* @return a value between 0 and 1
*/
public abstract double getInclusionProbability(Instance instance);
//TODO: for non sphere cluster sample points, find out MIN MAX neighbours within cluster
//and return the relative distance
//public abstract double getRelativeHullDistance(Instance instance);
@Override
public void getDescription(StringBuilder sb, int i) {
sb.append("Cluster Object");
}
public void setId(double id) {
this.id = id;
}
public double getId() {
return id;
}
public boolean isGroundTruth(){
return gtLabel != -1;
}
public void setGroundTruth(double truth){
gtLabel = truth;
}
public double getGroundTruth(){
return gtLabel;
}
/**
* Samples this cluster by returning a point from inside it.
* @param random a random number source
* @return an Instance that lies inside this cluster
*/
public abstract Instance sample(Random random);
public void setMeasureValue(String measureKey, String value){
measure_values.put(measureKey, value);
}
public void setMeasureValue(String measureKey, double value){
measure_values.put(measureKey, Double.toString(value));
}
public String getMeasureValue(String measureKey){
if(measure_values.containsKey(measureKey))
return measure_values.get(measureKey);
else
return "";
}
protected void getClusterSpecificInfo(ArrayList infoTitle,ArrayList infoValue){
infoTitle.add("ClusterID");
infoValue.add(Integer.toString((int)getId()));
infoTitle.add("Type");
infoValue.add(getClass().getSimpleName());
double c[] = getCenter();
if(c!=null)
for (int i = 0; i < c.length; i++) {
infoTitle.add("Dim"+i);
infoValue.add(Double.toString(c[i]));
}
infoTitle.add("Weight");
infoValue.add(Double.toString(getWeight()));
}
public String getInfo() {
ArrayList infoTitle = new ArrayList();
ArrayList infoValue = new ArrayList();
getClusterSpecificInfo(infoTitle, infoValue);
StringBuffer sb = new StringBuffer();
//Cluster properties
sb.append("");
sb.append("");
int i = 0;
while(i < infoTitle.size() && i < infoValue.size()){
sb.append(""+infoTitle.get(i)+" "+infoValue.get(i)+" ");
i++;
}
sb.append("
");
//Evaluation info
sb.append("
");
sb.append("Evaluation
");
sb.append("");
Iterator miterator = measure_values.entrySet().iterator();
while(miterator.hasNext()) {
Map.Entry e = (Map.Entry)miterator.next();
sb.append(""+e.getKey()+" "+e.getValue()+" ");
}
sb.append("
");
sb.append("");
return sb.toString();
}
}
© 2015 - 2025 Weber Informatics LLC | Privacy Policy