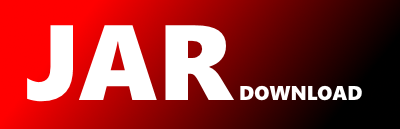
moa.clusterers.outliers.utils.mtree.PartitionFunctions Maven / Gradle / Ivy
Go to download
Show more of this group Show more artifacts with this name
Show all versions of moa Show documentation
Show all versions of moa Show documentation
Massive On-line Analysis is an environment for massive data mining. MOA
provides a framework for data stream mining and includes tools for evaluation
and a collection of machine learning algorithms. Related to the WEKA project,
also written in Java, while scaling to more demanding problems.
/*
* This program is free software; you can redistribute it and/or modify
* it under the terms of the GNU General Public License as published by
* the Free Software Foundation; either version 3 of the License, or
* (at your option) any later version.
*
* This program is distributed in the hope that it will be useful,
* but WITHOUT ANY WARRANTY; without even the implied warranty of
* MERCHANTABILITY or FITNESS FOR A PARTICULAR PURPOSE. See the
* GNU General Public License for more details.
*
* You should have received a copy of the GNU General Public License
* along with this program. If not, see .
*
*/
package moa.clusterers.outliers.utils.mtree;
import java.util.ArrayList;
import java.util.Collections;
import java.util.Comparator;
import java.util.HashSet;
import java.util.List;
import java.util.Set;
import moa.clusterers.outliers.utils.mtree.utils.Pair;
/**
* Some pre-defined implementations of {@linkplain PartitionFunction partition
* functions}.
*/
public final class PartitionFunctions {
/**
* Don't let anyone instantiate this class.
*/
private PartitionFunctions() {}
/**
* A {@linkplain PartitionFunction partition function} that tries to
* distribute the data objects equally between the promoted data objects,
* associating to each promoted data objects the nearest data objects.
*
* @param The type of the data objects.
*/
public static class BalancedPartition implements PartitionFunction {
/**
* Processes the balanced partition.
*
* The algorithm is roughly equivalent to this:
*
* While dataSet is not Empty:
* X := The object in dataSet which is nearest to promoted.first
* Remove X from dataSet
* Add X to result.first
*
* Y := The object in dataSet which is nearest to promoted.second
* Remove Y from dataSet
* Add Y to result.second
*
* Return result
*
*
* @see mtree.PartitionFunction#process(mtree.utils.Pair, java.util.Set, mtree.DistanceFunction)
*/
@Override
public Pair> process(
final Pair promoted,
Set dataSet,
final DistanceFunction super DATA> distanceFunction
)
{
List queue1 = new ArrayList(dataSet);
// Sort by distance to the first promoted data
Collections.sort(queue1, new Comparator() {
@Override
public int compare(DATA data1, DATA data2) {
double distance1 = distanceFunction.calculate(data1, promoted.first);
double distance2 = distanceFunction.calculate(data2, promoted.first);
return Double.compare(distance1, distance2);
}
});
List queue2 = new ArrayList(dataSet);
// Sort by distance to the second promoted data
Collections.sort(queue2, new Comparator() {
@Override
public int compare(DATA data1, DATA data2) {
double distance1 = distanceFunction.calculate(data1, promoted.second);
double distance2 = distanceFunction.calculate(data2, promoted.second);
return Double.compare(distance1, distance2);
}
});
Pair> partitions = new Pair>(new HashSet(), new HashSet());
int index1 = 0;
int index2 = 0;
while(index1 < queue1.size() || index2 != queue2.size()) {
while(index1 < queue1.size()) {
DATA data = queue1.get(index1++);
if(!partitions.second.contains(data)) {
partitions.first.add(data);
break;
}
}
while(index2 < queue2.size()) {
DATA data = queue2.get(index2++);
if(!partitions.first.contains(data)) {
partitions.second.add(data);
break;
}
}
}
return partitions;
}
}
}
© 2015 - 2025 Weber Informatics LLC | Privacy Policy