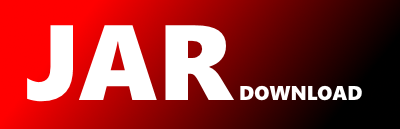
moa.evaluation.LearningPerformanceEvaluator Maven / Gradle / Ivy
Go to download
Show more of this group Show more artifacts with this name
Show all versions of moa Show documentation
Show all versions of moa Show documentation
Massive On-line Analysis is an environment for massive data mining. MOA
provides a framework for data stream mining and includes tools for evaluation
and a collection of machine learning algorithms. Related to the WEKA project,
also written in Java, while scaling to more demanding problems.
/*
* LearningPerformanceEvaluator.java
* Copyright (C) 2009 University of Waikato, Hamilton, New Zealand
* @author Albert Bifet ([email protected])
*
* This program is free software; you can redistribute it and/or modify
* it under the terms of the GNU General Public License as published by
* the Free Software Foundation; either version 3 of the License, or
* (at your option) any later version.
*
* This program is distributed in the hope that it will be useful,
* but WITHOUT ANY WARRANTY; without even the implied warranty of
* MERCHANTABILITY or FITNESS FOR A PARTICULAR PURPOSE. See the
* GNU General Public License for more details.
*
* You should have received a copy of the GNU General Public License
* along with this program. If not, see .
*
*/
package moa.evaluation;
import com.yahoo.labs.samoa.instances.InstanceData;
import com.yahoo.labs.samoa.instances.Prediction;
import moa.MOAObject;
import moa.core.Example;
import moa.core.Measurement;
/**
* Interface implemented by learner evaluators to monitor
* the results of the learning process.
*
* @author Richard Kirkby ([email protected])
* @version $Revision: 7 $
*/
public interface LearningPerformanceEvaluator extends MOAObject {
/**
* Resets this evaluator. It must be similar to
* starting a new evaluator from scratch.
*
*/
public void reset();
/**
* Adds a learning result to this evaluator.
*
* @param example the example to be classified
* @param classVotes an array containing the estimated membership
* probabilities of the test instance in each class
* @return an array of measurements monitored in this evaluator
*/
public void addResult(E testInst, Prediction prediction);
public void addResult(E example, double[] classVotes);
/**
* Gets the current measurements monitored by this evaluator.
*
* @return an array of measurements monitored by this evaluator
*/
public Measurement[] getPerformanceMeasurements();
}
© 2015 - 2025 Weber Informatics LLC | Privacy Policy