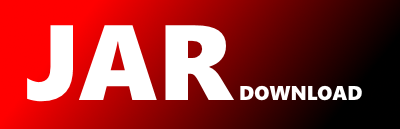
moa.classifiers.rules.multilabel.AMRulesMultiTargetRegressorSemiSuper Maven / Gradle / Ivy
Go to download
Show more of this group Show more artifacts with this name
Show all versions of moa Show documentation
Show all versions of moa Show documentation
Massive On-line Analysis is an environment for massive data mining. MOA
provides a framework for data stream mining and includes tools for evaluation
and a collection of machine learning algorithms. Related to the WEKA project,
also written in Java, while scaling to more demanding problems.
/*
* AMRulesMultiTargetRegressorSemiSuper.java
* Copyright (C) 2017 University of Porto, Portugal
* @author R. Sousa, J. Gama
*
* Licensed under the Apache License, Version 2.0 (the "License");
* you may not use this file except in compliance with the License.
* You may obtain a copy of the License at
*
* http://www.apache.org/licenses/LICENSE-2.0
*
* Unless required by applicable law or agreed to in writing, software
* distributed under the License is distributed on an "AS IS" BASIS,
* WITHOUT WARRANTIES OR CONDITIONS OF ANY KIND, either express or implied.
* See the License for the specific language governing permissions and
* limitations under the License.
*
*
*/
package moa.classifiers.rules.multilabel;
/**
* @author RSousa
* @version $Revision: 1 $
*/
import com.yahoo.labs.samoa.instances.MultiLabelInstance;
import moa.classifiers.MultiLabelLearner;
import moa.classifiers.MultiTargetLearnerSemiSupervised;
import moa.classifiers.MultiTargetRegressor;
import moa.classifiers.core.driftdetection.ChangeDetector;
import moa.classifiers.rules.multilabel.core.MultiLabelRule;
import moa.classifiers.rules.multilabel.core.MultiLabelRuleRegression;
import moa.classifiers.rules.multilabel.core.splitcriteria.MultiLabelSplitCriterion;
import moa.classifiers.rules.multilabel.core.splitcriteria.MultiTargetVarianceRatio;
import moa.classifiers.rules.multilabel.core.voting.ErrorWeightedVoteMultiLabel;
import moa.classifiers.rules.multilabel.core.voting.InverseErrorWeightedVoteMultiLabel;
import moa.classifiers.rules.multilabel.errormeasurers.MultiLabelErrorMeasurer;
import moa.classifiers.rules.multilabel.errormeasurers.RelativeMeanAbsoluteDeviationMT;
import moa.classifiers.rules.multilabel.functions.AdaptiveMultiTargetRegressor;
import moa.options.ClassOption;
public class AMRulesMultiTargetRegressorSemiSuper extends AMRulesMultiLabelLearnerSemiSuper implements MultiTargetLearnerSemiSupervised { //MultiTargetRegressor,
private static final long serialVersionUID = 1L;
@Override
public String getPurposeString() {
return "Semi-supervised AMRules method for online multi-target regression.This method measures predicts the benefit of a unlabeled example to the models(using only the input information) to the model. If this benefit is higher than then the algorithm predicts an output and artificially labels the example and use it for training.";
}
public AMRulesMultiTargetRegressorSemiSuper(){
splitCriterionOption = new ClassOption("splitCriterionOption", 'S',"Split criterion used to assess the merit of a split", MultiLabelSplitCriterion.class, MultiTargetVarianceRatio.class.getName()) ;
weightedVoteOption = new ClassOption("weightedVoteOption",'w', "Weighted vote type", ErrorWeightedVoteMultiLabel.class,InverseErrorWeightedVoteMultiLabel.class.getName());
learnerOption = new ClassOption("learnerOption",'L', "Learner", MultiTargetRegressor.class,AdaptiveMultiTargetRegressor.class.getName());
errorMeasurerOption = new ClassOption("errorMeasurer", 'e',"Measure of error for deciding which learner should predict.", MultiLabelErrorMeasurer.class, RelativeMeanAbsoluteDeviationMT.class.getName()) ;
changeDetector = new ClassOption("changeDetector",'H', "Change Detector.", ChangeDetector.class,"PageHinkleyDM -d 0.05 -l 35.0");
}
@Override
protected MultiLabelRule newDefaultRule() {
return new MultiLabelRuleRegression(1);
}
public AMRulesMultiTargetRegressorSemiSuper(double attributesPercentage) {
super(attributesPercentage);
}
}
© 2015 - 2025 Weber Informatics LLC | Privacy Policy