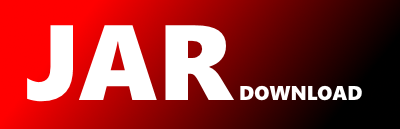
moa.classifiers.AbstractClassifier Maven / Gradle / Ivy
Go to download
Show more of this group Show more artifacts with this name
Show all versions of moa Show documentation
Show all versions of moa Show documentation
Massive On-line Analysis is an environment for massive data mining. MOA
provides a framework for data stream mining and includes tools for evaluation
and a collection of machine learning algorithms. Related to the WEKA project,
also written in Java, while scaling to more demanding problems.
The newest version!
/*
* AbstractClassifier.java
* Copyright (C) 2007 University of Waikato, Hamilton, New Zealand
* @author Richard Kirkby ([email protected])
*
* This program is free software; you can redistribute it and/or modify
* it under the terms of the GNU General Public License as published by
* the Free Software Foundation; either version 3 of the License, or
* (at your option) any later version.
*
* This program is distributed in the hope that it will be useful,
* but WITHOUT ANY WARRANTY; without even the implied warranty of
* MERCHANTABILITY or FITNESS FOR A PARTICULAR PURPOSE. See the
* GNU General Public License for more details.
*
* You should have received a copy of the GNU General Public License
* along with this program. If not, see .
*
*/
package moa.classifiers;
import java.util.Arrays;
import java.util.LinkedList;
import java.util.List;
import java.util.Random;
import moa.MOAObject;
import moa.capabilities.CapabilitiesHandler;
import moa.capabilities.Capability;
import moa.capabilities.ImmutableCapabilities;
import moa.core.Example;
import com.yahoo.labs.samoa.instances.InstancesHeader;
import moa.core.Measurement;
import moa.core.ObjectRepository;
import moa.core.StringUtils;
import moa.gui.AWTRenderer;
import moa.learners.Learner;
import moa.options.AbstractOptionHandler;
import com.github.javacliparser.IntOption;
import moa.tasks.TaskMonitor;
import com.yahoo.labs.samoa.instances.Instance;
import com.yahoo.labs.samoa.instances.Instances;
import com.yahoo.labs.samoa.instances.MultiLabelPrediction;
import com.yahoo.labs.samoa.instances.Prediction;
import moa.core.Utils;
public abstract class AbstractClassifier extends AbstractOptionHandler
implements Classifier, CapabilitiesHandler { //Learner> {
@Override
public String getPurposeString() {
return "MOA Classifier: " + getClass().getCanonicalName();
}
/** Header of the instances of the data stream */
protected InstancesHeader modelContext;
/** Sum of the weights of the instances trained by this model */
protected double trainingWeightSeenByModel = 0.0;
/** Random seed used in randomizable learners */
protected int randomSeed = 1;
/** Option for randomizable learners to change the random seed */
protected IntOption randomSeedOption;
/** Random Generator used in randomizable learners */
public Random classifierRandom;
/**
* Creates an classifier and setups the random seed option
* if the classifier is randomizable.
*/
public AbstractClassifier() {
if (isRandomizable()) {
this.randomSeedOption = new IntOption("randomSeed", 'r',
"Seed for random behaviour of the classifier.", 1);
}
}
@Override
public void prepareForUseImpl(TaskMonitor monitor,
ObjectRepository repository) {
if (this.randomSeedOption != null) {
this.randomSeed = this.randomSeedOption.getValue();
}
if (!trainingHasStarted()) {
resetLearning();
}
}
@Override
public double[] getVotesForInstance(Example example){
return getVotesForInstance(example.getData());
}
@Override
public abstract double[] getVotesForInstance(Instance inst);
@Override
public Prediction getPredictionForInstance(Example example){
return getPredictionForInstance(example.getData());
}
@Override
public Prediction getPredictionForInstance(Instance inst){
Prediction prediction= new MultiLabelPrediction(1);
prediction.setVotes(getVotesForInstance(inst));
return prediction;
}
@Override
public void setModelContext(InstancesHeader ih) {
if ((ih != null) && (ih.classIndex() < 0)) {
throw new IllegalArgumentException(
"Context for a classifier must include a class to learn");
}
if (trainingHasStarted()
&& (this.modelContext != null)
&& ((ih == null) || !contextIsCompatible(this.modelContext, ih))) {
throw new IllegalArgumentException(
"New context is not compatible with existing model");
}
this.modelContext = ih;
}
@Override
public InstancesHeader getModelContext() {
return this.modelContext;
}
@Override
public void setRandomSeed(int s) {
this.randomSeed = s;
if (this.randomSeedOption != null) {
// keep option consistent
this.randomSeedOption.setValue(s);
}
}
@Override
public boolean trainingHasStarted() {
return this.trainingWeightSeenByModel > 0.0;
}
@Override
public double trainingWeightSeenByModel() {
return this.trainingWeightSeenByModel;
}
@Override
public void resetLearning() {
this.trainingWeightSeenByModel = 0.0;
if (isRandomizable()) {
this.classifierRandom = new Random(this.randomSeed);
}
resetLearningImpl();
}
@Override
public void trainOnInstance(Instance inst) {
boolean isTraining = (inst.weight() > 0.0);
if (this instanceof SemiSupervisedLearner == false &&
inst.classIsMissing() == true){
isTraining = false;
}
if (isTraining) {
this.trainingWeightSeenByModel += inst.weight();
trainOnInstanceImpl(inst);
}
}
@Override
public Measurement[] getModelMeasurements() {
List measurementList = new LinkedList();
measurementList.add(new Measurement("model training instances",
trainingWeightSeenByModel()));
measurementList.add(new Measurement("model serialized size (bytes)",
measureByteSize()));
Measurement[] modelMeasurements = getModelMeasurementsImpl();
if (modelMeasurements != null) {
measurementList.addAll(Arrays.asList(modelMeasurements));
}
// add average of sub-model measurements
Learner[] subModels = getSublearners();
if ((subModels != null) && (subModels.length > 0)) {
List subMeasurements = new LinkedList();
for (Learner subModel : subModels) {
if (subModel != null) {
subMeasurements.add(subModel.getModelMeasurements());
}
}
Measurement[] avgMeasurements = Measurement.averageMeasurements(subMeasurements.toArray(new Measurement[subMeasurements.size()][]));
measurementList.addAll(Arrays.asList(avgMeasurements));
}
return measurementList.toArray(new Measurement[measurementList.size()]);
}
@Override
public void getDescription(StringBuilder out, int indent) {
StringUtils.appendIndented(out, indent, "Model type: ");
out.append(this.getClass().getName());
StringUtils.appendNewline(out);
Measurement.getMeasurementsDescription(getModelMeasurements(), out,
indent);
StringUtils.appendNewlineIndented(out, indent, "Model description:");
StringUtils.appendNewline(out);
if (trainingHasStarted()) {
getModelDescription(out, indent);
} else {
StringUtils.appendIndented(out, indent,
"Model has not been trained.");
}
}
@Override
public Learner[] getSublearners() {
return getSubClassifiers();
}
@Override
public Classifier[] getSubClassifiers() {
return null;
}
@Override
public Classifier copy() {
return (Classifier) super.copy();
}
@Override
public MOAObject getModel(){
return this;
};
@Override
public void trainOnInstance(Example example){
trainOnInstance(example.getData());
}
@Override
public boolean correctlyClassifies(Instance inst) {
return Utils.maxIndex(getVotesForInstance(inst)) == (int) inst.classValue();
}
/**
* Gets the name of the attribute of the class from the header.
*
* @return the string with name of the attribute of the class
*/
public String getClassNameString() {
return InstancesHeader.getClassNameString(this.modelContext);
}
/**
* Gets the name of a label of the class from the header.
*
* @param classLabelIndex the label index
* @return the name of the label of the class
*/
public String getClassLabelString(int classLabelIndex) {
return InstancesHeader.getClassLabelString(this.modelContext,
classLabelIndex);
}
/**
* Gets the name of an attribute from the header.
*
* @param attIndex the attribute index
* @return the name of the attribute
*/
public String getAttributeNameString(int attIndex) {
return InstancesHeader.getAttributeNameString(this.modelContext,
attIndex);
}
/**
* Gets the name of a value of an attribute from the header.
*
* @param attIndex the attribute index
* @param valIndex the value of the attribute
* @return the name of the value of the attribute
*/
public String getNominalValueString(int attIndex, int valIndex) {
return InstancesHeader.getNominalValueString(this.modelContext,
attIndex, valIndex);
}
/**
* Returns if two contexts or headers of instances are compatible.
*
* Two contexts are compatible if they follow the following rules:
* Rule 1: num classes can increase but never decrease
* Rule 2: num attributes can increase but never decrease
* Rule 3: num nominal attribute values can increase but never decrease
* Rule 4: attribute types must stay in the same order (although class
* can move; is always skipped over)
*
* Attribute names are free to change, but should always still represent
* the original attributes.
*
* @param originalContext the first context to compare
* @param newContext the second context to compare
* @return true if the two contexts are compatible.
*/
public static boolean contextIsCompatible(InstancesHeader originalContext,
InstancesHeader newContext) {
if (newContext.numClasses() < originalContext.numClasses()) {
return false; // rule 1
}
if (newContext.numAttributes() < originalContext.numAttributes()) {
return false; // rule 2
}
int oPos = 0;
int nPos = 0;
while (oPos < originalContext.numAttributes()) {
if (oPos == originalContext.classIndex()) {
oPos++;
if (!(oPos < originalContext.numAttributes())) {
break;
}
}
if (nPos == newContext.classIndex()) {
nPos++;
}
if (originalContext.attribute(oPos).isNominal()) {
if (!newContext.attribute(nPos).isNominal()) {
return false; // rule 4
}
if (newContext.attribute(nPos).numValues() < originalContext.attribute(oPos).numValues()) {
return false; // rule 3
}
} else {
assert (originalContext.attribute(oPos).isNumeric());
if (!newContext.attribute(nPos).isNumeric()) {
return false; // rule 4
}
}
oPos++;
nPos++;
}
return true; // all checks clear
}
/**
* Returns the AWT Renderer
*
* @return the AWT Renderer
*/
@Override
public AWTRenderer getAWTRenderer() {
// TODO should return a default renderer here
// - or should null be interpreted as the default?
return null;
}
/**
* Resets this classifier. It must be similar to
* starting a new classifier from scratch.
*
* The reason for ...Impl methods: ease programmer burden by not requiring
* them to remember calls to super in overridden methods.
* Note that this will produce compiler errors if not overridden.
*/
public abstract void resetLearningImpl();
/**
* Trains this classifier incrementally using the given instance.
*
* The reason for ...Impl methods: ease programmer burden by not requiring
* them to remember calls to super in overridden methods.
* Note that this will produce compiler errors if not overridden.
*
* @param inst the instance to be used for training
*/
public abstract void trainOnInstanceImpl(Instance inst);
/**
* Gets the current measurements of this classifier.
*
* The reason for ...Impl methods: ease programmer burden by not requiring
* them to remember calls to super in overridden methods.
* Note that this will produce compiler errors if not overridden.
*
* @return an array of measurements to be used in evaluation tasks
*/
protected abstract Measurement[] getModelMeasurementsImpl();
/**
* Returns a string representation of the model.
*
* @param out the stringbuilder to add the description
* @param indent the number of characters to indent
*/
public abstract void getModelDescription(StringBuilder out, int indent);
/**
* Gets the index of the attribute in the instance,
* given the index of the attribute in the learner.
*
* @param index the index of the attribute in the learner
* @param inst the instance
* @return the index in the instance
*/
protected static int modelAttIndexToInstanceAttIndex(int index,
Instance inst) {
return inst.classIndex() > index ? index : index + 1;
}
/**
* Gets the index of the attribute in a set of instances,
* given the index of the attribute in the learner.
*
* @param index the index of the attribute in the learner
* @param insts the instances
* @return the index of the attribute in the instances
*/
protected static int modelAttIndexToInstanceAttIndex(int index,
Instances insts) {
return insts.classIndex() > index ? index : index + 1;
}
@Override
public ImmutableCapabilities defineImmutableCapabilities() {
// We are restricting classifiers based on view mode
return new ImmutableCapabilities(Capability.VIEW_STANDARD);
}
}
© 2015 - 2025 Weber Informatics LLC | Privacy Policy