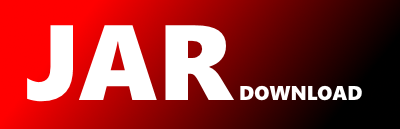
moa.classifiers.core.attributeclassobservers.BinaryTreeNumericAttributeClassObserver Maven / Gradle / Ivy
Go to download
Show more of this group Show more artifacts with this name
Show all versions of moa Show documentation
Show all versions of moa Show documentation
Massive On-line Analysis is an environment for massive data mining. MOA
provides a framework for data stream mining and includes tools for evaluation
and a collection of machine learning algorithms. Related to the WEKA project,
also written in Java, while scaling to more demanding problems.
The newest version!
/*
* BinaryTreeNumericAttributeClassObserver.java
* Copyright (C) 2007 University of Waikato, Hamilton, New Zealand
* @author Richard Kirkby ([email protected]), Andreas Hapfelmeier ([email protected])
*
* This program is free software; you can redistribute it and/or modify
* it under the terms of the GNU General Public License as published by
* the Free Software Foundation; either version 3 of the License, or
* (at your option) any later version.
*
* This program is distributed in the hope that it will be useful,
* but WITHOUT ANY WARRANTY; without even the implied warranty of
* MERCHANTABILITY or FITNESS FOR A PARTICULAR PURPOSE. See the
* GNU General Public License for more details.
*
* You should have received a copy of the GNU General Public License
* along with this program. If not, see .
*
*
*/
package moa.classifiers.core.attributeclassobservers;
import java.io.Serializable;
import moa.classifiers.core.AttributeSplitSuggestion;
import moa.classifiers.core.conditionaltests.NumericAttributeBinaryTest;
import moa.classifiers.core.splitcriteria.SplitCriterion;
import moa.core.DoubleVector;
import moa.core.ObjectRepository;
import moa.options.AbstractOptionHandler;
import moa.tasks.TaskMonitor;
/**
* Class for observing the class data distribution for a numeric attribute using a binary tree.
* This observer monitors the class distribution of a given attribute.
* Used in naive Bayes and decision trees to monitor data statistics on leaves.
*
* @author Richard Kirkby ([email protected])
* @version $Revision: 7 $
*/
public class BinaryTreeNumericAttributeClassObserver extends AbstractOptionHandler
implements NumericAttributeClassObserver {
private static final long serialVersionUID = 1L;
public class Node implements Serializable {
private static final long serialVersionUID = 1L;
public double cut_point;
public DoubleVector classCountsLeft = new DoubleVector();
public DoubleVector classCountsRight = new DoubleVector();
public Node left;
public Node right;
public Node(double val, int label, double weight) {
this.cut_point = val;
this.classCountsLeft.addToValue(label, weight);
}
public void insertValue(double val, int label, double weight) {
if (val == this.cut_point) {
this.classCountsLeft.addToValue(label, weight);
} else if (val <= this.cut_point) {
this.classCountsLeft.addToValue(label, weight);
if (this.left == null) {
this.left = new Node(val, label, weight);
} else {
this.left.insertValue(val, label, weight);
}
} else { // val > cut_point
this.classCountsRight.addToValue(label, weight);
if (this.right == null) {
this.right = new Node(val, label, weight);
} else {
this.right.insertValue(val, label, weight);
}
}
}
}
public Node root = null;
@Override
public void observeAttributeClass(double attVal, int classVal, double weight) {
if (Double.isNaN(attVal)) { //Instance.isMissingValue(attVal)
} else {
if (this.root == null) {
this.root = new Node(attVal, classVal, weight);
} else {
this.root.insertValue(attVal, classVal, weight);
}
}
}
@Override
public double probabilityOfAttributeValueGivenClass(double attVal,
int classVal) {
// TODO: NaiveBayes broken until implemented
return 0.0;
}
@Override
public AttributeSplitSuggestion getBestEvaluatedSplitSuggestion(
SplitCriterion criterion, double[] preSplitDist, int attIndex,
boolean binaryOnly) {
return searchForBestSplitOption(this.root, null, null, null, null, false,
criterion, preSplitDist, attIndex);
}
protected AttributeSplitSuggestion searchForBestSplitOption(
Node currentNode, AttributeSplitSuggestion currentBestOption,
double[] actualParentLeft,
double[] parentLeft, double[] parentRight, boolean leftChild,
SplitCriterion criterion, double[] preSplitDist, int attIndex) {
if (currentNode == null) {
return currentBestOption;
}
DoubleVector leftDist = new DoubleVector();
DoubleVector rightDist = new DoubleVector();
if (parentLeft == null) {
leftDist.addValues(currentNode.classCountsLeft);
rightDist.addValues(currentNode.classCountsRight);
} else {
leftDist.addValues(parentLeft);
rightDist.addValues(parentRight);
if (leftChild) {
//get the exact statistics of the parent value
DoubleVector exactParentDist = new DoubleVector();
exactParentDist.addValues(actualParentLeft);
exactParentDist.subtractValues(currentNode.classCountsLeft);
exactParentDist.subtractValues(currentNode.classCountsRight);
// move the subtrees
leftDist.subtractValues(currentNode.classCountsRight);
rightDist.addValues(currentNode.classCountsRight);
// move the exact value from the parent
rightDist.addValues(exactParentDist);
leftDist.subtractValues(exactParentDist);
} else {
leftDist.addValues(currentNode.classCountsLeft);
rightDist.subtractValues(currentNode.classCountsLeft);
}
}
double[][] postSplitDists = new double[][]{leftDist.getArrayRef(),
rightDist.getArrayRef()};
double merit = criterion.getMeritOfSplit(preSplitDist, postSplitDists);
if ((currentBestOption == null) || (merit > currentBestOption.merit)) {
currentBestOption = new AttributeSplitSuggestion(
new NumericAttributeBinaryTest(attIndex,
currentNode.cut_point, true), postSplitDists, merit);
}
currentBestOption = searchForBestSplitOption(currentNode.left,
currentBestOption, currentNode.classCountsLeft.getArrayRef(), postSplitDists[0], postSplitDists[1], true,
criterion, preSplitDist, attIndex);
currentBestOption = searchForBestSplitOption(currentNode.right,
currentBestOption, currentNode.classCountsLeft.getArrayRef(), postSplitDists[0], postSplitDists[1], false,
criterion, preSplitDist, attIndex);
return currentBestOption;
}
@Override
public void getDescription(StringBuilder sb, int indent) {
// TODO Auto-generated method stub
}
@Override
protected void prepareForUseImpl(TaskMonitor monitor, ObjectRepository repository) {
// TODO Auto-generated method stub
}
@Override
public void observeAttributeTarget(double attVal, double target) {
throw new UnsupportedOperationException("Not supported yet.");
}
}
© 2015 - 2025 Weber Informatics LLC | Privacy Policy