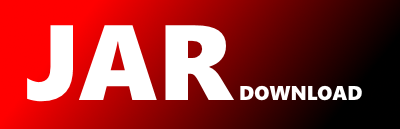
moa.classifiers.meta.DynamicWeightedMajority Maven / Gradle / Ivy
Go to download
Show more of this group Show more artifacts with this name
Show all versions of moa Show documentation
Show all versions of moa Show documentation
Massive On-line Analysis is an environment for massive data mining. MOA
provides a framework for data stream mining and includes tools for evaluation
and a collection of machine learning algorithms. Related to the WEKA project,
also written in Java, while scaling to more demanding problems.
The newest version!
/*
* DynamicWeightedMajority.java
* Copyright (C) 2016 Instituto Federal de Pernambuco
* @author Paulo Gonçalves ([email protected])
*
* This program is free software; you can redistribute it and/or modify
* it under the terms of the GNU General Public License as published by
* the Free Software Foundation; either version 3 of the License, or
* (at your option) any later version.
*
* This program is distributed in the hope that it will be useful,
* but WITHOUT ANY WARRANTY; without even the implied warranty of
* MERCHANTABILITY or FITNESS FOR A PARTICULAR PURPOSE. See the
* GNU General Public License for more details.
*
* You should have received a copy of the GNU General Public License
* along with this program. If not, see .
*
*/
package moa.classifiers.meta;
import com.github.javacliparser.FloatOption;
import com.github.javacliparser.IntOption;
import com.yahoo.labs.samoa.instances.Instance;
import java.util.ArrayList;
import java.util.List;
import moa.classifiers.AbstractClassifier;
import moa.classifiers.Classifier;
import moa.classifiers.MultiClassClassifier;
import moa.core.Measurement;
import moa.options.ClassOption;
import weka.core.Utils;
/**
* Dynamic weighted majority algorithm.
*
* Extends the Weighted Majority Algorithm to add and remove experts based on
* local and global accuracy.
*
*
* J. Zico Kolter and Marcus A. Maloof. Dynamic weighted majority: An ensemble
* method for drifting concepts. The Journal of Machine Learning Research,
* 8:2755-2790, December 2007. ISSN 1532-4435. URL
* http://dl.acm.org/citation.cfm?id=1314498.1390333.
*
*
*
* Based on the source code provided by the author at
*
* http://people.cs.georgetown.edu/~maloof/pubs/jmlr07.php
*
* @author Paulo Goncalves (paulogoncalves at recife dot ifpe dot edu dot br)
*
*/
public class DynamicWeightedMajority extends AbstractClassifier implements MultiClassClassifier {
public ClassOption baseLearnerOption = new ClassOption("baseLearner", 'l',
"Base classifiers to train.", Classifier.class, "bayes.NaiveBayes");
public IntOption periodOption = new IntOption("period", 'p',
"Period between expert removal, creation, and weight update.", 50,
1, Integer.MAX_VALUE);
public FloatOption betaOption = new FloatOption("beta", 'b',
"Factor to punish mistakes by.", 0.5, 0.0, 1.0);
public FloatOption thetaOption = new FloatOption("theta", 't',
"Minimum fraction of weight per model.", 0.01, 0.0, 1.0);
public IntOption maxExpertsOption = new IntOption("maxExperts", 'e',
"Maximum number of allowed experts.", Integer.MAX_VALUE, 2,
Integer.MAX_VALUE);
protected List experts;
protected List weights;
protected long epochs;
@Override
public void resetLearningImpl() {
this.experts = new ArrayList<>(50);
Classifier classifier = ((Classifier) getPreparedClassOption(this.baseLearnerOption)).copy();
classifier.resetLearning();
this.experts.add(classifier);
this.weights = new ArrayList<>(50);
this.weights.add(1.0);
this.epochs = 0;
}
protected void scaleWeights(double maxWeight) {
double sf = 1.0 / maxWeight;
for (int i = 0; i < weights.size(); i++) {
weights.set(i, weights.get(i) * sf);
}
}
protected void removeExperts() {
for (int i = experts.size() - 1; i >= 0; i--) {
if (weights.get(i) < this.thetaOption.getValue()) {
experts.remove(i);
weights.remove(i);
} // if
} // for
} // DWM::removeExperts
protected void removeWeakestExpert(int i) {
experts.remove(i);
weights.remove(i);
} // DWM::removeWeakestExpert
@Override
public void trainOnInstanceImpl(Instance inst) {
this.epochs++;
double[] Pr = new double[inst.numClasses()];
double maxWeight = 0.0;
double weakestExpertWeight = 1.0;
int weakestExpertIndex = -1;
// Loop over experts
for (int i = 0; i < this.experts.size(); i++) {
double[] pr = this.experts.get(i).getVotesForInstance(inst);
int yHat = Utils.maxIndex(pr);
if ((yHat != (int) inst.classValue())
&& this.epochs % this.periodOption.getValue() == 0) {
this.weights.set(i,
this.weights.get(i) * this.betaOption.getValue());
}
Pr[yHat] += this.weights.get(i);
maxWeight = Math.max(maxWeight, this.weights.get(i));
if (this.weights.get(i) < weakestExpertWeight) {
weakestExpertIndex = i;
weakestExpertWeight = weights.get(i);
}
}
int yHat = Utils.maxIndex(Pr);
if (this.epochs % this.periodOption.getValue() == 0) {
scaleWeights(maxWeight);
removeExperts();
if (yHat != (int) inst.classValue()) {
if (experts.size() == this.maxExpertsOption.getValue()) {
removeWeakestExpert(weakestExpertIndex);
}
Classifier classifier = ((Classifier) getPreparedClassOption(this.baseLearnerOption)).copy();
classifier.resetLearning();
this.experts.add(classifier);
this.weights.add(1.0);
}
}
// train experts
for (Classifier expert : this.experts) {
expert.trainOnInstance(inst);
}
}
@Override
public boolean isRandomizable() {
return false;
}
@Override
public double[] getVotesForInstance(Instance inst) {
double[] Pr = new double[inst.numClasses()];
for (int i = 0; i < this.experts.size(); i++) {
double[] pr = this.experts.get(i).getVotesForInstance(inst);
int yHat = Utils.maxIndex(pr);
Pr[yHat] += this.weights.get(i);
} // for
Utils.normalize(Pr);
return Pr;
}
@Override
protected Measurement[] getModelMeasurementsImpl() {
Measurement[] measurements = null;
if (this.weights != null) {
measurements = new Measurement[1];
measurements[0] = new Measurement("members size", this.weights.size());
}
return measurements;
}
@Override
public void getModelDescription(StringBuilder out, int indent) {
}
}
© 2015 - 2025 Weber Informatics LLC | Privacy Policy