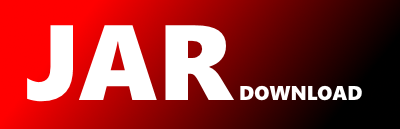
moa.classifiers.meta.HeterogeneousEnsembleBlastFadingFactors Maven / Gradle / Ivy
Go to download
Show more of this group Show more artifacts with this name
Show all versions of moa Show documentation
Show all versions of moa Show documentation
Massive On-line Analysis is an environment for massive data mining. MOA
provides a framework for data stream mining and includes tools for evaluation
and a collection of machine learning algorithms. Related to the WEKA project,
also written in Java, while scaling to more demanding problems.
The newest version!
/*
* HeterogeneousEnsembleBlastFadingFactors.java
* Copyright (C) 2017 University of Waikato, Hamilton, New Zealand
* @author Jan N. van Rijn ([email protected])
*
* This program is free software; you can redistribute it and/or modify
* it under the terms of the GNU General Public License as published by
* the Free Software Foundation; either version 3 of the License, or
* (at your option) any later version.
*
* This program is distributed in the hope that it will be useful,
* but WITHOUT ANY WARRANTY; without even the implied warranty of
* MERCHANTABILITY or FITNESS FOR A PARTICULAR PURPOSE. See the
* GNU General Public License for more details.
*
* You should have received a copy of the GNU General Public License
* along with this program. If not, see .
*
*/
package moa.classifiers.meta;
import com.github.javacliparser.FloatOption;
import com.yahoo.labs.samoa.instances.Instance;
import moa.classifiers.MultiClassClassifier;
/**
* BLAST (Best Last) for Heterogeneous Ensembles implemented with Fading Factors
*
*
* Given a set of (heterogeneous) classifiers, BLAST builds an ensemble, and
* determines the weights of all ensemble members based on their performance on
* recent observed instances. This implementation uses fading factors, to
* emphasize the importance of recent predictions and fade away old predictions.
*
*
*
* J. N. van Rijn, G. Holmes, B. Pfahringer, J. Vanschoren. Having a Blast:
* Meta-Learning and Heterogeneous Ensembles for Data Streams. In 2015 IEEE
* International Conference on Data Mining, pages 1003-1008. IEEE, 2015.
*
*
*
* Parameters:
*
*
* - -f : Fading factor
* - -b : Comma-separated string of classifiers
* - -g : Grace period (1 = optimal)
* - -k : Number of active classifiers
*
*
* @author Jan N. van Rijn ([email protected])
* @version $Revision: 1 $
*/
public class HeterogeneousEnsembleBlastFadingFactors
extends HeterogeneousEnsembleAbstract implements MultiClassClassifier {
private static final long serialVersionUID = 1L;
// Makes the model slightly more fault tolerant.
// Sometimes one of the base-models crashes, resulting
// in the meta-algorithm to crash as well. We ignore
// it when this happens only occasionally.
private static final int MAX_TOLLERATED_TRAINING_ERRROS = 100;
private int trainingErrors;
public FloatOption alphaOption = new FloatOption("alpha", 'a',
"The fading factor.", 0.999, 0, 1);
@Override
public void resetLearningImpl() {
this.historyTotal = new double[this.ensemble.length];
for (int i = 0; i < this.ensemble.length; ++i) {
this.historyTotal[i] = 1.0;
}
this.instancesSeen = 0;
this.trainingErrors = 0;
for (int i = 0; i < this.ensemble.length; i++) {
this.ensemble[i].resetLearning();
}
}
@Override
public void trainOnInstanceImpl(Instance inst) {
for (int i = 0; i < this.ensemble.length; i++) {
// Online Performance estimation
double[] votes = ensemble[i].getVotesForInstance(inst);
boolean correct = (maxIndex(votes) * 1.0 == inst.classValue());
historyTotal[i] = historyTotal[i] * alphaOption.getValue();
if (correct) {
historyTotal[i] += 1 - alphaOption.getValue();
}
try {
this.ensemble[i].trainOnInstance(inst);
} catch (RuntimeException e) {
this.trainingErrors += 1;
if (trainingErrors > MAX_TOLLERATED_TRAINING_ERRROS) {
throw new RuntimeException(
"Too much training errors! Latest: " + e.getMessage());
}
}
}
instancesSeen += 1;
if (instancesSeen % gracePerionOption.getValue() == 0) {
topK = topK(historyTotal, activeClassifiersOption.getValue());
}
}
}
© 2015 - 2025 Weber Informatics LLC | Privacy Policy