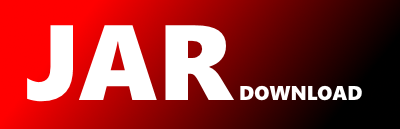
moa.classifiers.meta.PairedLearners Maven / Gradle / Ivy
Go to download
Show more of this group Show more artifacts with this name
Show all versions of moa Show documentation
Show all versions of moa Show documentation
Massive On-line Analysis is an environment for massive data mining. MOA
provides a framework for data stream mining and includes tools for evaluation
and a collection of machine learning algorithms. Related to the WEKA project,
also written in Java, while scaling to more demanding problems.
The newest version!
/*
* PairedLearners.java
* Copyright (C) 2015 Instituto Federal de Pernambuco, Recife, Brazil
* @author Paulo Gonçalves ([email protected])
*
* This program is free software; you can redistribute it and/or modify
* it under the terms of the GNU General Public License as published by
* the Free Software Foundation; either version 3 of the License, or
* (at your option) any later version.
*
* This program is distributed in the hope that it will be useful,
* but WITHOUT ANY WARRANTY; without even the implied warranty of
* MERCHANTABILITY or FITNESS FOR A PARTICULAR PURPOSE. See the
* GNU General Public License for more details.
*
* You should have received a copy of the GNU General Public License
* along with this program. If not, see .
*/
package moa.classifiers.meta;
import com.github.javacliparser.FloatOption;
import com.github.javacliparser.IntOption;
import java.util.Arrays;
import java.util.LinkedList;
import java.util.List;
import moa.classifiers.AbstractClassifier;
import moa.classifiers.Classifier;
import moa.classifiers.MultiClassClassifier;
import moa.core.Measurement;
import moa.options.ClassOption;
import com.yahoo.labs.samoa.instances.Instance;
import moa.core.MiscUtils;
/**
* Creates two classifiers: a stable and a reactive. The first represents the
* actual stable concept, while the second is trained on the most recent data.
* If the accuracy of the reactive is higher than that of the stable, it means
* the concept has changed. The stable classifier is then substituted by the
* reactive, and the reactive is reset.
*
*
* Stephen H. Bach, Marcus A. Maloof, "Paired Learners for Concept Drift",
* Eighth IEEE International Conference on Data Mining (ICDM), 2008,
* pp.23-32
*
* @author Paulo Gonçalves (paulogoncalves at recife.ifpe.edu.br)
*
*/
public class PairedLearners extends AbstractClassifier implements MultiClassClassifier {
private static final long serialVersionUID = 1L;
public ClassOption stableLearnerOption = new ClassOption("stableLearner",
's', "Stable learner", Classifier.class, "bayes.NaiveBayes");
public ClassOption reactiveLearnerOption = new ClassOption("reactiveLearner",
'r', "Reactive learner", Classifier.class, "bayes.NaiveBayes");
public IntOption windowSizeOption = new IntOption("windowSize",
'w', "Window size for the reactive learner",
12, 1, Integer.MAX_VALUE);
public FloatOption thresholdOption = new FloatOption("threshold",
't', "Threashold for creating a new stable learner",
0.2, 0, 1);
protected int[] c;
protected Classifier stableLearner;
protected Classifier reactiveLearner;
protected int t;
protected int w;
protected int theta;
protected Instance[] instances;
protected int changeDetected = 0;
protected int numberOfErrors = 0;
protected int i;
@Override
public void resetLearningImpl() {
this.t = 0;
this.w = this.windowSizeOption.getValue();
this.c = new int[this.w];
this.theta = (int) (this.w * this.thresholdOption.getValue());
this.instances = new Instance[this.w];
this.stableLearner = ((Classifier) getPreparedClassOption(this.stableLearnerOption)).copy();
this.stableLearner.resetLearning();
this.reactiveLearner = ((Classifier) getPreparedClassOption(this.reactiveLearnerOption)).copy();
this.reactiveLearner.resetLearning();
}
@Override
public void trainOnInstanceImpl(Instance inst) {
this.instances[this.t] = inst;
int trueClass = (int) inst.classValue();
boolean stablePrediction = MiscUtils.maxIndex(this.stableLearner.getVotesForInstance(inst)) == trueClass;
boolean reactivePrediction = MiscUtils.maxIndex(this.reactiveLearner.getVotesForInstance(inst)) == trueClass;
this.numberOfErrors = this.numberOfErrors - this.c[this.t];
if(!stablePrediction && reactivePrediction) {
this.c[this.t] = 1;
this.numberOfErrors++;
} else {
this.c[this.t] = 0;
}
if (this.theta < this.numberOfErrors) {
this.changeDetected++;
this.stableLearner = this.reactiveLearner.copy();
Arrays.fill(this.c, 0); // Resets c
this.numberOfErrors = 0;
}
this.stableLearner.trainOnInstance(inst);
this.reactiveLearner.resetLearning();
for (i=0; i measurementList = new LinkedList();
measurementList.add(new Measurement("Change detected", this.changeDetected));
Measurement[] modelMeasurements = ((AbstractClassifier) this.stableLearner).getModelMeasurements();
if (modelMeasurements != null) {
measurementList.addAll(Arrays.asList(modelMeasurements));
}
this.changeDetected = 0;
return measurementList.toArray(new Measurement[measurementList.size()]);
}
@Override
public void getModelDescription(StringBuilder out, int indent) {
}
}
© 2015 - 2025 Weber Informatics LLC | Privacy Policy