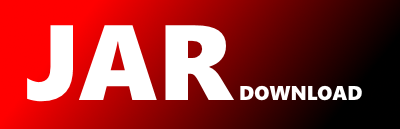
moa.classifiers.multilabel.core.splitcriteria.PCTWeightedICVarianceReduction Maven / Gradle / Ivy
Go to download
Show more of this group Show more artifacts with this name
Show all versions of moa Show documentation
Show all versions of moa Show documentation
Massive On-line Analysis is an environment for massive data mining. MOA
provides a framework for data stream mining and includes tools for evaluation
and a collection of machine learning algorithms. Related to the WEKA project,
also written in Java, while scaling to more demanding problems.
The newest version!
package moa.classifiers.multilabel.core.splitcriteria;
import moa.core.DoubleVector;
public class PCTWeightedICVarianceReduction extends WeightedICVarianceReduction {
private static final long serialVersionUID = 1L;
public DoubleVector inputWeights;
public double targetInputTradeoff; // \in [0, 1]
public PCTWeightedICVarianceReduction(DoubleVector targetWeights, DoubleVector inputWeights,
double targetInputTradeoff) {
super(targetWeights);
this.inputWeights = inputWeights;
this.targetInputTradeoff = targetInputTradeoff;
}
public double getMeritOfSplit(DoubleVector[] preSplitTargetDist, DoubleVector[][] postSplitTargetDists,
DoubleVector[] preSplitInputDist, DoubleVector[][] postSplitInputDists) {
double targetError = 0;
double inputError = 0;
int numTargets = preSplitTargetDist.length;
int numInputs = preSplitInputDist.length;
for (int i = 0; i < numTargets; i++) {
if (postSplitTargetDists[i][0].getValue(0) == 0 || postSplitTargetDists[i][1].getValue(0) == 0)
// If the split would result in a leaf without labeled instances, discard it
return Double.NaN;
targetError += weights.getValue(i)
* getMeritOfSplitForAttribute(preSplitTargetDist[i], postSplitTargetDists[i]);
}
for (int i = 0; i < numInputs; i++)
inputError += inputWeights.getValue(i)
* getMeritOfSplitForAttribute(preSplitInputDist[i], postSplitInputDists[i]);
return targetInputTradeoff * targetError / weights.sumOfValues()
+ (1 - targetInputTradeoff) * inputError / inputWeights.sumOfValues();
}
//@Override
protected double getMeritOfSplitForAttribute(DoubleVector preSplitDist, DoubleVector[] postSplitDists) {
double merit = 0;
// count number of branches with weightSeen higher than threshold
int count = 0;
for (int i = 0; i < postSplitDists.length; i++)
if (postSplitDists[i].getValue(0) >= 0.05 * preSplitDist.getValue(0))
count = count + 1;
// Consider split if all branches have required weight seen
if (count == postSplitDists.length) {
double varPreSplit = computeVariance(preSplitDist);
double sumVarPostSplit = 0;
double weightTotal = 0;
for (int i = 0; i < postSplitDists.length; i++) {
weightTotal += postSplitDists[i].getValue(0);
}
double[] variances = getBranchSplitVarianceOutput(postSplitDists);
for (int i = 0; i < variances.length; i++)
if (postSplitDists[i].getValue(0) > 0)
sumVarPostSplit += (postSplitDists[i].getValue(0) / weightTotal * variances[i]); // weight variance
if (varPreSplit > 0.0)
merit = 1 - sumVarPostSplit / varPreSplit;
else
merit = 0.0;
}
/*
* if(merit<0 || merit>1) System.out.println("out of range");
*/
return merit;
}
// private double computeVariance(double n, double sum, double squares) {
// if (n > 1) {
// return (squares - sum * sum / n) / (n - 1);
// }
// return 0;
// }
// private double computeVariance(DoubleVector v) {
// return computeVariance(v.getValue(0), v.getValue(1), v.getValue(2));
// }
public double getMeritOfSplit(DoubleVector[] preSplitTargetDist, DoubleVector[] preSplitInputDist,
DoubleVector[][] postSplitTargetDists, DoubleVector[][] postSplitInputDists) {
double error = 0;
int numOutputs = preSplitTargetDist.length;
for (int i = 0; i < numOutputs; i++)
error += weights.getValue(i) * getMeritOfSplitForOutput(preSplitTargetDist, postSplitTargetDists, i);
return error / weights.sumOfValues();
}
}
© 2015 - 2025 Weber Informatics LLC | Privacy Policy