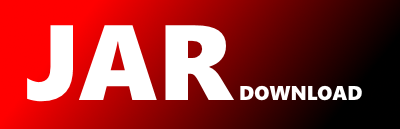
moa.classifiers.rules.featureranking.WeightedMajorityFeatureRanking Maven / Gradle / Ivy
Go to download
Show more of this group Show more artifacts with this name
Show all versions of moa Show documentation
Show all versions of moa Show documentation
Massive On-line Analysis is an environment for massive data mining. MOA
provides a framework for data stream mining and includes tools for evaluation
and a collection of machine learning algorithms. Related to the WEKA project,
also written in Java, while scaling to more demanding problems.
The newest version!
/*
* WeightedMajorityFeatureRanking.java
* Copyright (C) 2017 University of Porto, Portugal
* @author J. Duarte, J. Gama
*
* Licensed under the Apache License, Version 2.0 (the "License");
* you may not use this file except in compliance with the License.
* You may obtain a copy of the License at
*
* http://www.apache.org/licenses/LICENSE-2.0
*
* Unless required by applicable law or agreed to in writing, software
* distributed under the License is distributed on an "AS IS" BASIS,
* WITHOUT WARRANTIES OR CONDITIONS OF ANY KIND, either express or implied.
* See the License for the specific language governing permissions and
* limitations under the License.
*
*
*/
package moa.classifiers.rules.featureranking;
import java.util.HashMap;
import java.util.Iterator;
import java.util.LinkedList;
import java.util.List;
import moa.classifiers.rules.featureranking.messages.ChangeDetectedMessage;
import moa.classifiers.rules.featureranking.messages.MeritCheckMessage;
import moa.classifiers.rules.featureranking.messages.RuleExpandedMessage;
import moa.classifiers.rules.multilabel.core.ObservableMOAObject;
import moa.core.DoubleVector;
import com.github.javacliparser.FloatOption;
/**
* Weighted Majority Feature Ranking method
* João Duarte, João Gama,Feature ranking in hoeffding algorithms for regression. SAC 2017: 836-841
*/
public class WeightedMajorityFeatureRanking extends AbstractFeatureRanking implements FeatureRanking{
/**
*
*/
private static final long serialVersionUID = 1L;
protected double [] attributeImportance;
protected HashMap ruleInformation;
public FloatOption meritThresholdOption = new FloatOption(
"meritThreshold",
'm',
"Merit threshold value. If the merit of an input attribute is below the threshold its importance will decrease",
0.01, 0.0, 1.0);
public WeightedMajorityFeatureRanking() {
super();
this.ruleInformation = new HashMap();
}
public void update(ObservableMOAObject o, Object arg) {
if (arg instanceof MeritCheckMessage){
MeritCheckMessage msg = (MeritCheckMessage) arg;
//Get Learning attributes
boolean [] attributesMask=msg.getLearningAttributes().clone(); //because it will be modified
int numAttributes=attributesMask.length;
if(this.attributeImportance ==null){
this.attributeImportance= new double[numAttributes];
for (int i=0; i updated=ri.update(msg.getMerits().getArrayRef(), attributesMask, meritThresholdOption.getValue());
Iterator it = updated.iterator();
while(it.hasNext()){
int attIndex=it.next();
this.attributeImportance[attIndex]=(attributeImportance[attIndex]*numRules-
old[attIndex]+ri.getAttributeImportance(attIndex))/numRules;
}
}
}
//Rule expanded
else if (arg instanceof RuleExpandedMessage){
int numRules=ruleInformation.size();
RuleInformation ri=ruleInformation.get(o);
RuleExpandedMessage msg=((RuleExpandedMessage)arg);
int attIndex=msg.getAttributeIndex();
double oldValue=ri.getAttributeImportance(attIndex);
this.attributeImportance[attIndex]=(attributeImportance[attIndex]*numRules-oldValue+1)/numRules;
ri.addLiteralAttribute(attIndex);
}
//Rule will be removed
else if (arg instanceof ChangeDetectedMessage) {
RuleInformation ri=ruleInformation.get(o);
int numRules=ruleInformation.size();
double [] attribImportance=ri.getAttributesImportance();
for (int i=0; i literalAttributes= new LinkedList();
public RuleInformation(int numAttributes) {
attributeImportance=new double [numAttributes];
depth=0;
for(int i=0; i update(double [] merits, boolean [] attributesMask, double threshold){
boolean [] attributesMaskAux=attributesMask.clone();
Iterator it=literalAttributes.iterator();
while(it.hasNext())
attributesMaskAux[it.next()]=false;
List updated=new LinkedList();
for (int i=0; i
© 2015 - 2025 Weber Informatics LLC | Privacy Policy