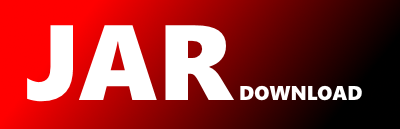
moa.classifiers.rules.multilabel.core.MultiLabelRule Maven / Gradle / Ivy
Go to download
Show more of this group Show more artifacts with this name
Show all versions of moa Show documentation
Show all versions of moa Show documentation
Massive On-line Analysis is an environment for massive data mining. MOA
provides a framework for data stream mining and includes tools for evaluation
and a collection of machine learning algorithms. Related to the WEKA project,
also written in Java, while scaling to more demanding problems.
The newest version!
package moa.classifiers.rules.multilabel.core;
import java.util.Iterator;
import java.util.LinkedList;
import java.util.List;
import java.util.Random;
import moa.classifiers.MultiLabelLearner;
import moa.classifiers.core.driftdetection.ChangeDetector;
import moa.classifiers.rules.core.anomalydetection.AnomalyDetector;
import moa.classifiers.rules.featureranking.messages.MeritCheckMessage;
import moa.classifiers.rules.featureranking.messages.RuleExpandedMessage;
import moa.classifiers.rules.multilabel.attributeclassobservers.NominalStatisticsObserver;
import moa.classifiers.rules.multilabel.attributeclassobservers.NumericStatisticsObserver;
import moa.classifiers.rules.multilabel.core.splitcriteria.MultiLabelSplitCriterion;
import moa.classifiers.rules.multilabel.errormeasurers.MultiLabelErrorMeasurer;
import moa.classifiers.rules.multilabel.inputselectors.InputAttributesSelector;
import moa.classifiers.rules.multilabel.instancetransformers.InstanceTransformer;
import moa.classifiers.rules.multilabel.outputselectors.OutputAttributesSelector;
import moa.core.DoubleVector;
import moa.core.StringUtils;
import com.yahoo.labs.samoa.instances.InstanceInformation;
import com.yahoo.labs.samoa.instances.InstancesHeader;
import com.yahoo.labs.samoa.instances.MultiLabelInstance;
import com.yahoo.labs.samoa.instances.Prediction;
public class MultiLabelRule extends ObservableMOAObject {
/**
*
*/
private static final long serialVersionUID = 1L;
protected List literalList = new LinkedList();
protected LearningLiteral learningLiteral;
protected int ruleNumberID;
protected MultiLabelRule otherBranchRule;
protected MultiLabelRule otherOutputsRule;
protected InstanceInformation instanceInformation;
//double [] attributesDemeritAccum; //forWeighted VoteFeatureRanking
public MultiLabelRule(LearningLiteral learningLiteral) {
this.learningLiteral=learningLiteral; //copy()?
}
public MultiLabelRule() {
}
public MultiLabelRule(int id) {
this();
ruleNumberID=id;
}
public int getRuleNumberID() {
return ruleNumberID;
}
public void setRuleNumberID(int ruleNumberID) {
this.ruleNumberID = ruleNumberID;
}
public boolean isCovering(MultiLabelInstance inst) {
boolean isCovering = true;
for (Literal l : literalList) {
if (l.evaluate(inst) == false) {
isCovering = false;
break;
}
}
return isCovering;
}
public int[] getOutputsCovered() {
return learningLiteral.getOutputsToLearn();
}
public int[] getInputsCovered() {
return learningLiteral.getInputsToLearn();
}
@Override
public void getDescription(StringBuilder out, int indent) {
StringUtils.appendIndented(out, indent+1, "Rule Nr." + this.ruleNumberID + " Instances seen:" + this.learningLiteral.getWeightSeenSinceExpansion() + "\n");
for (Literal literal : literalList) {
literal.getDescription(out, indent+1, instanceInformation);
StringUtils.appendIndented(out, indent+1, " ");
}
StringUtils.appendIndented(out, indent+1, " Output: " + this.learningLiteral.getStaticOutput(instanceInformation));
}
protected String getStaticOutput() {
return "";
}
public boolean updateChangeDetection(MultiLabelInstance instance) {
return this.learningLiteral.updateAndCheckChange(instance);
}
public boolean updateAnomalyDetection(MultiLabelInstance instance) {
return this.learningLiteral.updateAndCheckAnomalyDetection(instance);
}
public void trainOnInstance(MultiLabelInstance instance) {
if(this.instanceInformation==null)
this.instanceInformation=((InstancesHeader)instance.dataset()).getInstanceInformation();
learningLiteral.trainOnInstance(instance);
}
public double getWeightSeenSinceExpansion() {
return learningLiteral.getWeightSeenSinceExpansion();
}
public LearningLiteral getLearningNode() {
return learningLiteral;
}
public double [] getCurrentErrors() {
return learningLiteral.getErrors();
}
public Prediction getPredictionForInstance(MultiLabelInstance instance) {
return learningLiteral.getPredictionForInstance(instance);
}
public double getAnomalyScore(){
//xxxxxxxxxxxxxxxxxxxxxxxxxxxxxxxxxxxxxxxxxxxxxxxxxxxxxxxxxxxxxxxxxxxxxx
//System.out.print("MultiLabelRule.getAnomalyScore:AScore=" + this.learningLiteral.anomalyDetector.getAnomalyScore() + "\n");
//xxxxxxxxxxxxxxxxxxxxxxxxxxxxxxxxxxxxxxxxxxxxxxxxxxxxxxxxxxxxxxxxxxxxxx
return this.learningLiteral.anomalyDetector.getAnomalyScore();
}
public boolean tryToExpand(double splitConfidence, double tieThresholdOption) {
boolean hasExpanded=learningLiteral.tryToExpand(splitConfidence,tieThresholdOption);
//Merit check event
double[] merit=learningLiteral.getMeritInputAttributes();
if(merit!=null)
this.notifyAll( new MeritCheckMessage(new DoubleVector(merit), this.learningLiteral.getAttributeMask()));
if(hasExpanded){
LearningLiteral otherOutputsLiteral=learningLiteral.getOtherOutputsLearningLiteral();
//if && this.literalList.size()>0 is removed then consider including this code for default rule also
if(otherOutputsLiteral!=null && this.literalList.size()>0){ //only add "other outputs" rule if antecedent is not empty
otherOutputsRule=new MultiLabelRule();
otherOutputsRule.instanceInformation=instanceInformation;
otherOutputsRule.literalList=new LinkedList(this.literalList);
otherOutputsRule.learningLiteral=otherOutputsLiteral;
}
otherBranchRule=new MultiLabelRule((LearningLiteral)learningLiteral.getOtherBranchLearningLiteral());
//check for obsolete predicate
int attribIndex=learningLiteral.getBestSuggestion().getPredicate().getAttributeIndex();
boolean isEqualOrLess=learningLiteral.getBestSuggestion().getPredicate().isEqualOrLess();
boolean isSpecialization=false;
Iterator it=literalList.iterator();
while(it.hasNext()){
Literal l=it.next();
if(l.predicate.getAttributeIndex()==attribIndex && l.predicate.isEqualOrLess()==isEqualOrLess)
{
it.remove();
isSpecialization=true;
break;
}
}
//Rule expansion event
this.notifyAll(new RuleExpandedMessage(attribIndex, isSpecialization));
this.literalList.add(new Literal(learningLiteral.getBestSuggestion().getPredicate()));
learningLiteral=learningLiteral.getExpandedLearningLiteral();
}
return hasExpanded;
}
public MultiLabelRule getNewRuleFromOtherBranch(){
MultiLabelRule r=otherBranchRule;
otherBranchRule=null;
return r;
}
public MultiLabelRule getNewRuleFromOtherOutputs(){
MultiLabelRule r=otherOutputsRule;
return r;
}
@Override
public String toString()
{
StringBuilder out = new StringBuilder();
getDescription(out, 1);
return out.toString();
}
public void setSplitCriterion(MultiLabelSplitCriterion splitCriterion) {
learningLiteral.setSplitCriterion(splitCriterion);
}
public void setChangeDetector(ChangeDetector changeDetector) {
learningLiteral.setChangeDetector(changeDetector);
}
public void setAnomalyDetector(AnomalyDetector anomalyDetector) {
learningLiteral.setAnomalyDetector(anomalyDetector);
}
public void setNumericObserverOption(
NumericStatisticsObserver numericStatisticsObserver) {
learningLiteral.setNumericObserverOption(numericStatisticsObserver);
}
public void setLearner(MultiLabelLearner learner) {
learningLiteral.setLearner(learner);
}
public void setErrorMeasurer(MultiLabelErrorMeasurer errorMeasurer) {
learningLiteral.setErrorMeasurer(errorMeasurer);
}
public void setOutputAttributesSelector(OutputAttributesSelector outputSelector) {
learningLiteral.setOutputAttributesSelector(outputSelector);
}
public void setNominalObserverOption(NominalStatisticsObserver nominalStatisticsObserver) {
learningLiteral.setNominalObserverOption(nominalStatisticsObserver);
}
public void setRandomGenerator(Random random) {
learningLiteral.setRandomGenerator(random);
}
public void setAttributesPercentage(double attributesPercentage) {
learningLiteral.setAttributesPercentage(attributesPercentage);
}
public void setInputAttributesSelector(InputAttributesSelector inputSelector) {
learningLiteral.setInputAttributesSelector(inputSelector);
}
public boolean hasNewRuleFromOtherOutputs() {
return this.otherOutputsRule!=null;
}
public void setInstanceTransformer(InstanceTransformer instanceTransformer) {
learningLiteral.setInstanceTransformer(instanceTransformer);
}
@Override
public void addObserver(ObserverMOAObject o) {
observers.add(o);
}
public List getLiterals(){
return literalList;
}
public void clearOtherOutputs() {
otherOutputsRule=null;
}
}
© 2015 - 2025 Weber Informatics LLC | Privacy Policy