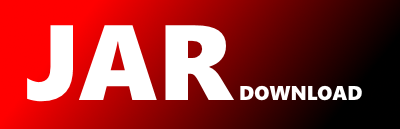
moa.classifiers.trees.iadem.IademGaussianNumericAttributeClassObserver Maven / Gradle / Ivy
Go to download
Show more of this group Show more artifacts with this name
Show all versions of moa Show documentation
Show all versions of moa Show documentation
Massive On-line Analysis is an environment for massive data mining. MOA
provides a framework for data stream mining and includes tools for evaluation
and a collection of machine learning algorithms. Related to the WEKA project,
also written in Java, while scaling to more demanding problems.
The newest version!
/*
* IademGaussianNumericAttributeClassObserver.java
*
* @author Isvani Frias-Blanco
*
* Licensed under the Apache License, Version 2.0 (the "License");
* you may not use this file except in compliance with the License.
* You may obtain a copy of the License at
*
* http://www.apache.org/licenses/LICENSE-2.0
*
* Unless required by applicable law or agreed to in writing, software
* distributed under the License is distributed on an "AS IS" BASIS,
* WITHOUT WARRANTIES OR CONDITIONS OF ANY KIND, either express or implied.
* See the License for the specific language governing permissions and
* limitations under the License.
*
*
*/
package moa.classifiers.trees.iadem;
import java.util.Arrays;
import java.util.ArrayList;
import moa.classifiers.core.attributeclassobservers.GaussianNumericAttributeClassObserver;
import moa.core.AutoExpandVector;
import moa.core.DoubleVector;
import moa.core.GaussianEstimator;
import weka.core.Utils;
public class IademGaussianNumericAttributeClassObserver
extends GaussianNumericAttributeClassObserver
implements IademNumericAttributeObserver {
private static final long serialVersionUID = 1L;
private int valueCount = 0;
protected DoubleVector classDist = new DoubleVector();
public IademGaussianNumericAttributeClassObserver() {
super();
}
public IademGaussianNumericAttributeClassObserver(int maxTuples) {
super();
this.numBinsOption.setValue(maxTuples);
}
@Override
public void addValue(double attValue, int classValue, double weight) {
if (Utils.isMissingValue(attValue)) {
} else {
this.valueCount += weight;
this.classDist.addToValue(classValue, weight);
observeAttributeClass(attValue, classValue, weight);
}
}
@Override
public long getValueCount() {
return this.valueCount;
}
@Override
public long[] getClassDist() {
long[] classDistCopy = new long[this.classDist.numValues()];
for (int i = 0; i < this.classDist.numValues(); i++) {
classDistCopy[i] = (long) this.classDist.getValue(i);
}
return classDistCopy;
}
@Override
public long getNumberOfCutPoints() {
return getSplitPointSuggestions().length;
}
@Override
public long[] getLeftClassDist(double cutValue) {
long[] lhsDist = new long[this.classDist.numValues()];
Arrays.fill(lhsDist, 0);
for (int i = 0; i < this.attValDistPerClass.size(); i++) {
GaussianEstimator estimator = this.attValDistPerClass.get(i);
if (estimator != null) {
if (cutValue < this.minValueObservedPerClass.getValue(i)) {
} else if (cutValue >= this.maxValueObservedPerClass.getValue(i)) {
lhsDist[i] = (long) estimator.getTotalWeightObserved();
} else {
double[] weightDist = estimator.estimatedWeight_LessThan_EqualTo_GreaterThan_Value(cutValue);
lhsDist[i] = (long) (weightDist[0] + weightDist[1]);
}
}
}
return lhsDist;
}
@Override
public double getCut(int index) {
return getSplitPointSuggestions()[index];
}
@Override
public void computeClassDistProbabilities(double[][][] cut_value_classDist_lower,
double[][][] cut_value_classDist_upper,
double[][] counts_cut_value,
boolean withIntervalEstimates) {
ArrayList cuts = cutPointSuggestion(-1);
long[] totalDist = getClassDist();
for (int i = 0; i < cuts.size(); i++) {
long[] lDist = getLeftClassDist(cuts.get(i)),
rDist = new long[lDist.length];
long leftTotal = sum(lDist);
long total = sum(totalDist);
counts_cut_value[i][0] = leftTotal;
counts_cut_value[i][1] = total - leftTotal;
for (int j = 0; j < totalDist.length; j++) {
rDist[j] = totalDist[j] - lDist[j];
double leftEst = 0.0;
if (counts_cut_value[i][0] != 0) {
leftEst = (double) lDist[j] / counts_cut_value[i][0];
}
double leftError = 0.0;
if (withIntervalEstimates) {
leftError = IademCommonProcedures.getIADEM_HoeffdingBound(leftEst, counts_cut_value[i][0]);
}
cut_value_classDist_lower[i][0][j] = Math.max(0.0, leftEst - leftError);
cut_value_classDist_upper[i][0][j] = Math.min(1.0, leftEst + leftError);
double rightEst = 0.0;
if (counts_cut_value[i][1] != 0) {
rightEst = (double) rDist[j] / counts_cut_value[i][1];
}
double rightError = 0.0;
if (withIntervalEstimates) {
rightError = IademCommonProcedures.getIADEM_HoeffdingBound(rightEst, counts_cut_value[i][1]);
}
cut_value_classDist_lower[i][1][j] = Math.max(0.0, rightEst - rightError);
cut_value_classDist_upper[i][1][j] = Math.min(1.0, rightEst + rightError);
}
}
}
protected long sum(long[] arr) {
long count = 0;
for (int i = 0; i < arr.length; i++) {
count += arr[i];
}
return count;
}
@Override
public ArrayList cutPointSuggestion(int total) {
ArrayList cuts = new ArrayList();
double[] arr = getSplitPointSuggestions();
for (int i = 0; i < arr.length; i++) {
cuts.add(arr[i]);
}
return cuts;
}
@Override
public ArrayList computeConditionalProbPerBin(ArrayList cuts) {
ArrayList probClassDistPerCut = new ArrayList();
long total = getValueCount();
for (Double currentCut : cuts) {
long[] numInstances = getLeftClassDist(currentCut);
Double[] prob = new Double[numInstances.length];
for (int j = 0; j < prob.length; j++) {
prob[j] = (double) numInstances[j] / total;
}
probClassDistPerCut.add(prob);
}
return probClassDistPerCut;
}
@Override
public double[] computeConditionalProb(ArrayList cuts, double cutValue) {
double[] conditionalProbability = new double[this.attValDistPerClass.size()];
for (int i = 0; i < this.attValDistPerClass.size(); i++) {
conditionalProbability[i] = probabilityOfAttributeValueGivenClass(cutValue, i);
}
return conditionalProbability;
}
@Override
public void reset() {
this.minValueObservedPerClass = new DoubleVector();
this.maxValueObservedPerClass = new DoubleVector();
this.attValDistPerClass = new AutoExpandVector();
this.valueCount = 0;
this.classDist = new DoubleVector();
}
//***********************************************************************
// probably deprecated
@Override
public long getMaxOfValues() {
return this.numBinsOption.getValue();
}
@Override
public IademNumericAttributeObserver getCopy() {
throw new UnsupportedOperationException("Not supported yet."); //To change body of generated methods, choose Tools | Templates.
}
@Override
public void setMaxBins(int numberOfBins) {
this.numBinsOption.setValue(numberOfBins);
}
@Override
public void computeClassDist(double[][][] cutClassDist) {
ArrayList cuts = cutPointSuggestion(-1);
long[] totalDist = getClassDist();
for (int i = 0; i < cuts.size(); i++) {
long[] lDist = getLeftClassDist(cuts.get(i)),
rDist = new long[lDist.length];
for (int j = 0; j < totalDist.length; j++) {
rDist[j] = totalDist[j] - lDist[j];
cutClassDist[i][0][j] = lDist[j];
cutClassDist[i][1][j] = rDist[j];
}
}
}
}
© 2015 - 2025 Weber Informatics LLC | Privacy Policy