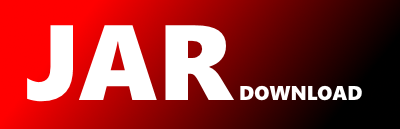
moa.clusterers.WekaClusteringAlgorithm Maven / Gradle / Ivy
Go to download
Show more of this group Show more artifacts with this name
Show all versions of moa Show documentation
Show all versions of moa Show documentation
Massive On-line Analysis is an environment for massive data mining. MOA
provides a framework for data stream mining and includes tools for evaluation
and a collection of machine learning algorithms. Related to the WEKA project,
also written in Java, while scaling to more demanding problems.
The newest version!
/*
* WekaClusteringAlgorithm.java
* Copyright (C) 2010 RWTH Aachen University, Germany
* @author Jansen ([email protected])
*
* This program is free software; you can redistribute it and/or modify
* it under the terms of the GNU General Public License as published by
* the Free Software Foundation; either version 3 of the License, or
* (at your option) any later version.
*
* This program is distributed in the hope that it will be useful,
* but WITHOUT ANY WARRANTY; without even the implied warranty of
* MERCHANTABILITY or FITNESS FOR A PARTICULAR PURPOSE. See the
* GNU General Public License for more details.
*
* You should have received a copy of the GNU General Public License
* along with this program. If not, see .
*
*/
package moa.clusterers;
import java.util.ArrayList;
import java.util.List;
import moa.cluster.Clustering;
import moa.core.AutoClassDiscovery;
import moa.core.AutoExpandVector;
import moa.core.Measurement;
import moa.options.ClassOption;
import com.github.javacliparser.IntOption;
import com.github.javacliparser.MultiChoiceOption;
import com.github.javacliparser.StringOption;
import moa.core.FastVector;
import com.yahoo.labs.samoa.instances.Attribute;
import com.yahoo.labs.samoa.instances.DenseInstance;
import com.yahoo.labs.samoa.instances.Instance;
import com.yahoo.labs.samoa.instances.Instances;
import com.yahoo.labs.samoa.instances.SamoaToWekaInstanceConverter;
import weka.core.Utils;
public class WekaClusteringAlgorithm extends AbstractClusterer {
private static final long serialVersionUID = 1L;
public IntOption horizonOption = new IntOption("horizon",
'h', "Range of the window.", 1000);
public MultiChoiceOption wekaAlgorithmOption;
public StringOption parameterOption = new StringOption("parameter", 'p',
"Parameters that will be passed to the weka algorithm. (e.g. '-N 5' for using SimpleKmeans with 5 clusters)", "-N 5 -S 8");
private Class>[] clustererClasses;
private Instances instances;
private weka.clusterers.AbstractClusterer clusterer;
protected SamoaToWekaInstanceConverter instanceConverter;
public WekaClusteringAlgorithm() {
clustererClasses = findWekaClustererClasses();
String[] optionLabels = new String[clustererClasses.length];
String[] optionDescriptions = new String[clustererClasses.length];
for (int i = 0; i < clustererClasses.length; i++) {
optionLabels[i] = clustererClasses[i].getSimpleName();
optionDescriptions[i] = clustererClasses[i].getName();
// We do have the parameter option info, but not really a place to show it somewhere
/*
//System.out.println(clustererClasses[i].getSimpleName());
for (Class c : clustererClasses[i].getInterfaces()) {
if (c.equals(weka.core.OptionHandler.class)) {
try {
Enumeration options = ((weka.core.OptionHandler)clustererClasses[i].newInstance()).listOptions();
while(options.hasMoreElements()){
weka.core.Option o = (weka.core.Option)options.nextElement();
System.out.print(o.synopsis()+" ");
}
} catch (InstantiationException e) {
e.printStackTrace();
} catch (IllegalAccessException e) {
e.printStackTrace();
}
}
}
*/
}
if (clustererClasses != null && clustererClasses.length > 0) {
wekaAlgorithmOption = new MultiChoiceOption("clusterer", 'w',
"Weka cluster algorithm to use.",
optionLabels, optionDescriptions, 6);
} else {
horizonOption = null;
parameterOption = null;
}
}
@Override
public void resetLearningImpl() {
try {
instances = null;
String clistring = clustererClasses[wekaAlgorithmOption.getChosenIndex()].getName();
clusterer = (weka.clusterers.AbstractClusterer) ClassOption.cliStringToObject(clistring, weka.clusterers.Clusterer.class, null);
String rawOptions = parameterOption.getValue();
String[] options = rawOptions.split(" ");
if (clusterer instanceof weka.core.OptionHandler) {
((weka.core.OptionHandler) clusterer).setOptions(options);
Utils.checkForRemainingOptions(options);
}
} catch (Exception e) {
e.printStackTrace();
}
this.instanceConverter = new SamoaToWekaInstanceConverter();
}
@Override
public void trainOnInstanceImpl(Instance inst) {
if (instances == null) {
instances = getDataset(inst.numAttributes(), 0);
}
instances.add(inst);
}
public Clustering getClusteringResult() {
Clustering clustering = null;
weka.core.Instances wekaInstances= this.instanceConverter.wekaInstances(instances);
try {
clusterer.buildClusterer(wekaInstances);
int numClusters = clusterer.numberOfClusters();
Instances dataset = getDataset(instances.numAttributes(), numClusters);
List newInstances = new ArrayList() ; //Instances(dataset);
for (int i = 0; i < wekaInstances.numInstances(); i++) {
weka.core.Instance inst = wekaInstances.get(i);
int cnum = clusterer.clusterInstance(inst);
Instance newInst = new DenseInstance(instances.instance(cnum));
newInst.insertAttributeAt(inst.numAttributes());
newInst.setDataset(dataset);
newInst.setClassValue(cnum);
newInstances.add(newInst);
}
clustering = new Clustering(newInstances);
} catch (Exception e) {
e.printStackTrace();
}
instances = null;
return clustering;
}
public Instances getDataset(int numdim, int numclass) {
FastVector attributes = new FastVector();
for (int i = 0; i < numdim; i++) {
attributes.addElement(new Attribute("att" + (i + 1)));
}
if (numclass > 0) {
FastVector classLabels = new FastVector();
for (int i = 0; i < numclass; i++) {
classLabels.addElement("class" + (i + 1));
}
attributes.addElement(new Attribute("class", classLabels));
}
Instances myDataset = new Instances("horizion", attributes, 0);
if (numclass > 0) {
myDataset.setClassIndex(myDataset.numAttributes() - 1);
}
return myDataset;
}
private Class>[] findWekaClustererClasses() {
AutoExpandVector> finalClasses = new AutoExpandVector>();
Class>[] classesFound = AutoClassDiscovery.findClassesOfType("weka.clusterers",
weka.clusterers.AbstractClusterer.class);
for (Class> foundClass : classesFound) {
finalClasses.add(foundClass);
}
return finalClasses.toArray(new Class>[finalClasses.size()]);
}
@Override
protected Measurement[] getModelMeasurementsImpl() {
throw new UnsupportedOperationException("Not supported yet.");
}
@Override
public void getModelDescription(StringBuilder out, int indent) {
}
public boolean isRandomizable() {
return false;
}
public double[] getVotesForInstance(Instance inst) {
return null;
}
@Override
public boolean keepClassLabel() {
return false;
}
@Override
public String getPurposeString() {
String purpose = "MOA Clusterer: " + getClass().getCanonicalName();
if (clustererClasses == null || clustererClasses.length == 0) {
purpose += "\nPlease add weka.jar to the classpath to use Weka clustering algorithms.";
}
return purpose;
}
}
© 2015 - 2025 Weber Informatics LLC | Privacy Policy