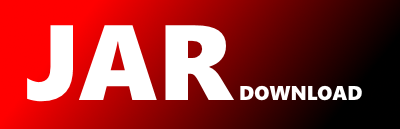
moa.clusterers.meta.BooleanParameter Maven / Gradle / Ivy
Go to download
Show more of this group Show more artifacts with this name
Show all versions of moa Show documentation
Show all versions of moa Show documentation
Massive On-line Analysis is an environment for massive data mining. MOA
provides a framework for data stream mining and includes tools for evaluation
and a collection of machine learning algorithms. Related to the WEKA project,
also written in Java, while scaling to more demanding problems.
The newest version!
package moa.clusterers.meta;
import java.util.ArrayList;
import java.util.HashMap;
import com.yahoo.labs.samoa.instances.Attribute;
// the representation of a boolean / binary / flag parameter
public class BooleanParameter implements IParameter {
private String parameter;
private int numericValue;
private String value;
private String[] range = { "false", "true" };
private Attribute attribute;
private ArrayList probabilities;
private boolean optimise;
public BooleanParameter(BooleanParameter x) {
this.parameter = x.parameter;
this.numericValue = x.numericValue;
this.value = x.value;
this.attribute = x.attribute;
this.optimise = x.optimise;
if(this.optimise){
this.range = x.range.clone();
this.probabilities = new ArrayList(x.probabilities);
}
}
public BooleanParameter(ParameterConfiguration x) {
this.parameter = x.parameter;
this.value = String.valueOf(x.value);
for (int i = 0; i < this.range.length; i++) {
if (this.range[i].equals(this.value)) {
this.numericValue = i; // get index of init value
}
}
this.attribute = new Attribute(x.parameter);
this.optimise = x.optimise;
if(this.optimise){
this.probabilities = new ArrayList(2);
for (int i = 0; i < 2; i++) {
this.probabilities.add(0.5); // equal probabilities
}
}
}
public BooleanParameter copy() {
return new BooleanParameter(this);
}
public String getCLIString() {
// if option is set
if (this.numericValue == 1) {
return ("-" + this.parameter); // only the parameter
}
return "";
}
public String getCLIValueString() {
if (this.numericValue == 1) {
return ("");
} else {
return (null);
}
}
public double getValue() {
return this.numericValue;
}
public String getParameter() {
return this.parameter;
}
public String[] getRange() {
return this.range;
}
public void sampleNewConfig(double lambda, double reset, int verbose) {
if(!this.optimise){
return;
}
if (Math.random() < reset) {
for (int i = 0; i < this.probabilities.size(); i++) {
this.probabilities.set(i, 1.0/this.probabilities.size());
}
}
HashMap map = new HashMap();
for (int i = 0; i < this.probabilities.size(); i++) {
map.put(i, this.probabilities.get(i));
}
// update configuration
this.numericValue = EnsembleClustererAbstract.sampleProportionally(map, true);
String newValue = this.range[this.numericValue];
if (verbose >= 3) {
System.out
.print("Sample new configuration for boolean parameter -" + this.parameter + " with probabilities");
for (int i = 0; i < this.probabilities.size(); i++) {
System.out.print(" " + this.probabilities.get(i));
}
System.out.println("\t=>\t -" + this.parameter + " " + newValue);
}
this.value = newValue;
// adapt distribution
// this.probabilities.set(this.numericValue,
// this.probabilities.get(this.numericValue) + (1.0/iter));
this.probabilities.set(this.numericValue,
this.probabilities.get(this.numericValue) * (2 - Math.pow(2, -1 * lambda)));
// divide by sum
double sum = 0.0;
for (int i = 0; i < this.probabilities.size(); i++) {
sum += this.probabilities.get(i);
}
for (int i = 0; i < this.probabilities.size(); i++) {
this.probabilities.set(i, this.probabilities.get(i) / sum);
}
}
}
© 2015 - 2025 Weber Informatics LLC | Privacy Policy