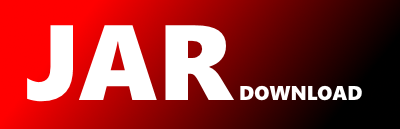
moa.evaluation.BasicMultiTargetPerformanceEvaluator Maven / Gradle / Ivy
Go to download
Show more of this group Show more artifacts with this name
Show all versions of moa Show documentation
Show all versions of moa Show documentation
Massive On-line Analysis is an environment for massive data mining. MOA
provides a framework for data stream mining and includes tools for evaluation
and a collection of machine learning algorithms. Related to the WEKA project,
also written in Java, while scaling to more demanding problems.
The newest version!
/*
* BasicRegressionPerformanceEvaluator.java
* Copyright (C) 2011 University of Waikato, Hamilton, New Zealand
* @author Albert Bifet (abifet at cs dot waikato dot ac dot nz)
*
* This program is free software; you can redistribute it and/or modify
* it under the terms of the GNU General Public License as published by
* the Free Software Foundation; either version 3 of the License, or
* (at your option) any later version.
*
* This program is distributed in the hope that it will be useful,
* but WITHOUT ANY WARRANTY; without even the implied warranty of
* MERCHANTABILITY or FITNESS FOR A PARTICULAR PURPOSE. See the
* GNU General Public License for more details.
*
* You should have received a copy of the GNU General Public License
* along with this program. If not, see .
*
*/
package moa.evaluation;
import moa.AbstractMOAObject;
import moa.core.Example;
import moa.core.Measurement;
import com.yahoo.labs.samoa.instances.DenseInstance;
import com.yahoo.labs.samoa.instances.DenseInstanceData;
import com.yahoo.labs.samoa.instances.Instance;
import com.yahoo.labs.samoa.instances.InstanceData;
import com.yahoo.labs.samoa.instances.MultiLabelInstance;
import com.yahoo.labs.samoa.instances.Prediction;
/**
* Regression evaluator that performs basic incremental evaluation.
*
* @author Albert Bifet (abifet at cs dot waikato dot ac dot nz)
* @version $Revision: 7 $
*/
public class BasicMultiTargetPerformanceEvaluator extends AbstractMOAObject
implements MultiTargetPerformanceEvaluator, RegressionPerformanceEvaluator{
private static final long serialVersionUID = 1L;
protected double weightObserved;
protected double squareError;
protected double averageError;
protected int numberOutputs;
@Override
public void reset() {
this.weightObserved = 0.0;
this.squareError = 0.0;
this.averageError = 0.0;
}
@Override
public void addResult(Example example, Prediction prediction) {
MultiLabelInstance inst = (MultiLabelInstance) example.getData();
if (numberOutputs == 0) {
numberOutputs = inst.numberOutputTargets();
}
if (inst.weight() > 0.0) {
this.weightObserved += inst.weight();
if (prediction != null ) {
for (int i = 0; i< numberOutputs;i++){
double err = inst.classValue(i) - ((prediction.numOutputAttributes()==0) ? 0.0 : prediction.getVote(i,0));
this.squareError += (err) * (err);
this.averageError += Math.abs(err);
}
}
//System.out.println(inst.classValue()+", "+prediction);
}
}
@Override
public Measurement[] getPerformanceMeasurements() {
return new Measurement[]{
new Measurement("classified instances",
getTotalWeightObserved()),
new Measurement("mean absolute error",
getMeanError()),
new Measurement("root mean squared error",
getSquareError())};
}
public double getTotalWeightObserved() {
return this.weightObserved;
}
public double getMeanError() {
return this.weightObserved > 0.0 ? this.averageError
/ (this.weightObserved * this.numberOutputs) : 0.0;
}
public double getSquareError() {
return Math.sqrt(this.weightObserved > 0.0 ? this.squareError
/ (this.weightObserved * this.numberOutputs): 0.0);
}
@Override
public void getDescription(StringBuilder sb, int indent) {
Measurement.getMeasurementsDescription(getPerformanceMeasurements(),
sb, indent);
}
@Override
public void addResult(Example example, double[] classVotes) {
// TODO Auto-generated method stub
}
}
© 2015 - 2025 Weber Informatics LLC | Privacy Policy