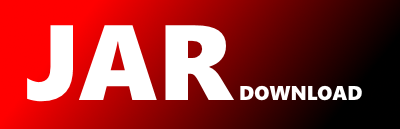
moa.gui.visualization.RunVisualizer Maven / Gradle / Ivy
Go to download
Show more of this group Show more artifacts with this name
Show all versions of moa Show documentation
Show all versions of moa Show documentation
Massive On-line Analysis is an environment for massive data mining. MOA
provides a framework for data stream mining and includes tools for evaluation
and a collection of machine learning algorithms. Related to the WEKA project,
also written in Java, while scaling to more demanding problems.
The newest version!
/*
* RunVisualizer.java
* Copyright (C) 2010 RWTH Aachen University, Germany
* @author Jansen ([email protected])
*
* Licensed under the Apache License, Version 2.0 (the "License");
* you may not use this file except in compliance with the License.
* You may obtain a copy of the License at
*
* http://www.apache.org/licenses/LICENSE-2.0
*
* Unless required by applicable law or agreed to in writing, software
* distributed under the License is distributed on an "AS IS" BASIS,
* WITHOUT WARRANTIES OR CONDITIONS OF ANY KIND, either express or implied.
* See the License for the specific language governing permissions and
* limitations under the License.
*
*
*/
package moa.gui.visualization;
import java.awt.Color;
import java.awt.event.ActionEvent;
import java.awt.event.ActionListener;
import java.io.BufferedWriter;
import java.io.FileWriter;
import java.io.IOException;
import java.io.PrintWriter;
import java.util.ArrayList;
import java.util.Iterator;
import java.util.LinkedList;
import java.util.List;
import java.util.logging.Level;
import java.util.logging.Logger;
import moa.cluster.Cluster;
import moa.cluster.Clustering;
import moa.clusterers.AbstractClusterer;
import moa.clusterers.ClusterGenerator;
import moa.evaluation.MeasureCollection;
import moa.gui.TextViewerPanel;
import moa.gui.clustertab.ClusteringSetupTab;
import moa.gui.clustertab.ClusteringVisualEvalPanel;
import moa.gui.clustertab.ClusteringVisualTab;
import moa.streams.clustering.ClusterEvent;
import com.yahoo.labs.samoa.instances.Instance;
import moa.gui.clustertab.ClusteringSetupTab;
import moa.streams.clustering.ClusterEventListener;
import moa.streams.clustering.ClusteringStream;
import moa.streams.clustering.RandomRBFGeneratorEvents;
import com.yahoo.labs.samoa.instances.Attribute;
import com.yahoo.labs.samoa.instances.DenseInstance;
import moa.core.FastVector;
import com.yahoo.labs.samoa.instances.Instances;
public class RunVisualizer implements Runnable, ActionListener, ClusterEventListener{
/** the pause interval, being read from the gui at startup */
public static final int initialPauseInterval = 5000;
/** factor to control the speed */
private int m_wait_frequency = 1000;
/** after how many instances do we repaint the streampanel?
* the GUI becomes very slow with small values
* */
private int m_redrawInterval = 100;
/* flags to control the run behavior */
private static boolean work;
private boolean stop = false;
/* total amount of processed instances */
private static int timestamp;
private static int lastPauseTimestamp;
/* amount of instances to process in one step*/
private int m_processFrequency;
/* the stream that delivers the instances */
private final ClusteringStream m_stream0;
/* amount of relevant instances; older instances will be dropped;
creates the 'sliding window' over the stream;
is strongly connected to the decay rate and decay threshold*/
private int m_stream0_decayHorizon;
/* the decay threshold defines the minimum weight of an instance to be relevant */
private double m_stream0_decay_threshold;
/* the decay rate of the stream, often reffered to as lambda;
is being calculated from the horizion and the threshold
as these are more intuitive to define */
private double m_stream0_decay_rate;
/* the clusterer */
private AbstractClusterer m_clusterer0;
private AbstractClusterer m_clusterer1;
/* the measure collections contain all the measures */
private MeasureCollection[] m_measures0 = null;
private MeasureCollection[] m_measures1 = null;
/* left and right stream panel that datapoints and clusterings will be drawn to */
private StreamPanel m_streampanel0;
private StreamPanel m_streampanel1;
/* panel that shows the evaluation results */
private ClusteringVisualEvalPanel m_evalPanel;
/* panel to hold the graph */
private GraphCanvas m_graphcanvas;
/* reference to the visual panel */
private ClusteringVisualTab m_visualPanel;
/* all possible clusterings */
//not pretty to have all the clusterings, but otherwise we can't just redraw clusterings
private Clustering gtClustering0 = null;
private Clustering gtClustering1 = null;
private Clustering macro0 = null;
private Clustering macro1 = null;
private Clustering micro0 = null;
private Clustering micro1 = null;
/* holds all the events that have happend, if the stream supports events */
private ArrayList clusterEvents;
/* reference to the log panel */
private final TextViewerPanel m_logPanel;
public RunVisualizer(ClusteringVisualTab visualPanel, ClusteringSetupTab clusteringSetupTab){
m_visualPanel = visualPanel;
m_streampanel0 = visualPanel.getLeftStreamPanel();
m_streampanel1 = visualPanel.getRightStreamPanel();
m_graphcanvas = visualPanel.getGraphCanvas();
m_evalPanel = visualPanel.getEvalPanel();
m_logPanel = clusteringSetupTab.getLogPanel();
m_stream0 = clusteringSetupTab.getStream0();
m_stream0_decayHorizon = m_stream0.getDecayHorizon();
m_stream0_decay_threshold = m_stream0.getDecayThreshold();
m_stream0_decay_rate = (Math.log(1.0/m_stream0_decay_threshold)/Math.log(2)/m_stream0_decayHorizon);
timestamp = 0;
lastPauseTimestamp = 0;
work = true;
if(m_stream0 instanceof RandomRBFGeneratorEvents){
((RandomRBFGeneratorEvents)m_stream0).addClusterChangeListener(this);
clusterEvents = new ArrayList();
m_graphcanvas.setClusterEventsList(clusterEvents);
}
m_stream0.prepareForUse();
m_clusterer0 = clusteringSetupTab.getClusterer0();
m_clusterer0.prepareForUse();
m_clusterer1 = clusteringSetupTab.getClusterer1();
if(m_clusterer1!=null){
m_clusterer1.prepareForUse();
}
m_measures0 = clusteringSetupTab.getMeasures();
m_measures1 = clusteringSetupTab.getMeasures();
/* TODO this option needs to move from the stream panel to the setup panel */
m_processFrequency = m_stream0.getEvaluationFrequency();
//get those values from the generator
int dims = m_stream0.numAttsOption.getValue();
visualPanel.setDimensionComobBoxes(dims);
visualPanel.setPauseInterval(initialPauseInterval);
m_evalPanel.setMeasures(m_measures0, m_measures1, this);
m_graphcanvas.setGraph(m_measures0[0], m_measures1[0],0,m_processFrequency);
}
public void run() {
runVisual();
}
public void runVisual() {
int processCounter = 0;
int speedCounter = 0;
LinkedList pointBuffer0 = new LinkedList();
LinkedList pointBuffer1 = new LinkedList();
ArrayList pointarray0 = null;
ArrayList pointarray1 = null;
while(work || processCounter!=0){
if (m_stream0.hasMoreInstances()) {
timestamp++;
speedCounter++;
processCounter++;
if(timestamp%100 == 0){
m_visualPanel.setProcessedPointsCounter(timestamp);
}
Instance next0 = m_stream0.nextInstance().getData();
DataPoint point0 = new DataPoint(next0,timestamp);
pointBuffer0.add(point0);
while(pointBuffer0.size() > m_stream0_decayHorizon){
pointBuffer0.removeFirst();
}
DataPoint point1 = null;
if(m_clusterer1!=null){
point1 = new DataPoint(next0,timestamp);
pointBuffer1.add(point1);
while(pointBuffer1.size() > m_stream0_decayHorizon){
pointBuffer1.removeFirst();
}
}
if(m_visualPanel.isEnabledDrawPoints()){
m_streampanel0.drawPoint(point0);
if(m_clusterer1!=null)
m_streampanel1.drawPoint(point1);
if(processCounter%m_redrawInterval==0){
m_streampanel0.applyDrawDecay(m_stream0_decayHorizon/(float)(m_redrawInterval));
if(m_clusterer1!=null)
m_streampanel1.applyDrawDecay(m_stream0_decayHorizon/(float)(m_redrawInterval));
}
}
Instance traininst0 = new DenseInstance(point0);
if(m_clusterer0.keepClassLabel())
traininst0.setDataset(point0.dataset());
else
traininst0.deleteAttributeAt(point0.classIndex());
m_clusterer0.trainOnInstanceImpl(traininst0);
if(m_clusterer1!=null){
Instance traininst1 = new DenseInstance(point1);
if(m_clusterer1.keepClassLabel())
traininst1.setDataset(point1.dataset());
else
traininst1.deleteAttributeAt(point1.classIndex());
m_clusterer1.trainOnInstanceImpl(traininst1);
}
if (processCounter >= m_processFrequency) {
processCounter = 0;
for(DataPoint p:pointBuffer0)
p.updateWeight(timestamp, m_stream0_decay_rate);
pointarray0 = new ArrayList(pointBuffer0);
if(m_clusterer1!=null){
for(DataPoint p:pointBuffer1)
p.updateWeight(timestamp, m_stream0_decay_rate);
pointarray1 = new ArrayList(pointBuffer1);
}
processClusterings(pointarray0, pointarray1);
int pauseInterval = m_visualPanel.getPauseInterval();
if(pauseInterval!=0 && lastPauseTimestamp+pauseInterval<=timestamp){
m_visualPanel.toggleVisualizer(true);
}
}
} else {
System.out.println("DONE");
return;
}
if(speedCounter > m_wait_frequency*30 && m_wait_frequency < 15){
try {
synchronized (this) {
if(m_wait_frequency == 0)
wait(50);
else
wait(1);
}
} catch (InterruptedException ex) {
}
speedCounter = 0;
}
}
if(!stop){
m_streampanel0.drawPointPanels(pointarray0, timestamp, m_stream0_decay_rate, m_stream0_decay_threshold);
if(m_clusterer1!=null)
m_streampanel1.drawPointPanels(pointarray1, timestamp, m_stream0_decay_rate, m_stream0_decay_threshold);
work_pause();
}
}
private void processClusterings(ArrayList points0, ArrayList points1){
gtClustering0 = new Clustering(points0);
gtClustering1 = null;
if(m_clusterer1!=null){
gtClustering1 = new Clustering(points1);
}
Clustering evalClustering0 = null;
Clustering evalClustering1 = null;
//special case for ClusterGenerator
if(gtClustering0!= null){
if(m_clusterer0 instanceof ClusterGenerator)
((ClusterGenerator)m_clusterer0).setSourceClustering(gtClustering0);
if(m_clusterer1 instanceof ClusterGenerator)
((ClusterGenerator)m_clusterer1).setSourceClustering(gtClustering1);
}
macro0 = m_clusterer0.getClusteringResult();
evalClustering0 = macro0;
//TODO: should we check if micro/macro is being drawn or needed for evaluation and skip otherwise to speed things up?
if(m_clusterer0.implementsMicroClusterer()){
micro0 = m_clusterer0.getMicroClusteringResult();
if(macro0 == null && micro0 != null){
//TODO: we need a Macro Clusterer Interface and the option for kmeans to use the non optimal centers
macro0 = moa.clusterers.KMeans.gaussianMeans(gtClustering0, micro0);
}
if(m_clusterer0.evaluateMicroClusteringOption.isSet())
evalClustering0 = micro0;
else
evalClustering0 = macro0;
}
if(m_clusterer1!=null){
macro1 = m_clusterer1.getClusteringResult();
evalClustering1 = macro1;
if(m_clusterer1.implementsMicroClusterer()){
micro1 = m_clusterer1.getMicroClusteringResult();
if(macro1 == null && micro1 != null){
macro1 = moa.clusterers.KMeans.gaussianMeans(gtClustering1, micro1);
}
if(m_clusterer1.evaluateMicroClusteringOption.isSet())
evalClustering1 = micro1;
else
evalClustering1 = macro1;
}
}
evaluateClustering(evalClustering0, gtClustering0, points0, true);
evaluateClustering(evalClustering1, gtClustering1, points1, false);
drawClusterings(points0, points1);
}
private void evaluateClustering(Clustering found_clustering, Clustering trueClustering, ArrayList points, boolean algorithm0){
StringBuilder sb = new StringBuilder();
for (int i = 0; i < m_measures0.length; i++) {
if(algorithm0){
if(found_clustering!=null && found_clustering.size() > 0){
try {
double msec = m_measures0[i].evaluateClusteringPerformance(found_clustering, trueClustering, points);
sb.append(m_measures0[i].getClass().getSimpleName()+" took "+msec+"ms (Mean:"+m_measures0[i].getMeanRunningTime()+")");
sb.append("\n");
} catch (Exception ex) { ex.printStackTrace(); }
}
else{
for(int j = 0; j < m_measures0[i].getNumMeasures(); j++){
m_measures0[i].addEmptyValue(j);
}
}
}
else{
if(m_clusterer1!=null && found_clustering!=null && found_clustering.size() > 0){
try {
double msec = m_measures1[i].evaluateClusteringPerformance(found_clustering, trueClustering, points);
sb.append(m_measures1[i].getClass().getSimpleName()+" took "+msec+"ms (Mean:"+m_measures1[i].getMeanRunningTime()+")");
sb.append("\n");
}
catch (Exception ex) { ex.printStackTrace(); }
}
else{
for(int j = 0; j < m_measures1[i].getNumMeasures(); j++){
m_measures1[i].addEmptyValue(j);
}
}
}
}
m_logPanel.setText(sb.toString());
m_evalPanel.update();
m_graphcanvas.updateCanvas();
}
public void drawClusterings(List points0, List points1){
if(macro0!= null && macro0.size() > 0)
m_streampanel0.drawMacroClustering(macro0, points0, Color.RED);
if(micro0!= null && micro0.size() > 0)
m_streampanel0.drawMicroClustering(micro0, points0, Color.GREEN);
if(gtClustering0!= null && gtClustering0.size() > 0)
m_streampanel0.drawGTClustering(gtClustering0, points0, Color.BLACK);
if(m_clusterer1!=null){
if(macro1!= null && macro1.size() > 0)
m_streampanel1.drawMacroClustering(macro1, points1, Color.BLUE);
if(micro1!= null && micro1.size() > 0)
m_streampanel1.drawMicroClustering(micro1, points1, Color.GREEN);
if(gtClustering1!= null && gtClustering1.size() > 0)
m_streampanel1.drawGTClustering(gtClustering1, points1, Color.BLACK);
}
}
public void redraw(){
m_streampanel0.repaint();
m_streampanel1.repaint();
}
public static int getCurrentTimestamp(){
return timestamp;
}
private void work_pause(){
while(!work && !stop){
try {
synchronized (this) {
wait(1000);
}
} catch (InterruptedException ex) {
}
}
run();
}
public static void pause(){
work = false;
lastPauseTimestamp = timestamp;
}
public static void resume(){
work = true;
}
public void stop(){
work = false;
stop = true;
}
public void setSpeed(int speed) {
m_wait_frequency = speed;
}
public void actionPerformed(ActionEvent e) {
//reacte on graph selection and find out which measure was selected
int selected = Integer.parseInt(e.getActionCommand());
int counter = selected;
int m_select = 0;
int m_select_offset = 0;
boolean found = false;
for (int i = 0; i < m_measures0.length; i++) {
for (int j = 0; j < m_measures0[i].getNumMeasures(); j++) {
if(m_measures0[i].isEnabled(j)){
counter--;
if(counter<0){
m_select = i;
m_select_offset = j;
found = true;
break;
}
}
}
if(found) break;
}
m_graphcanvas.setGraph(m_measures0[m_select], m_measures1[m_select],m_select_offset,m_processFrequency);
}
public void setPointLayerVisibility(boolean selected) {
m_streampanel0.setPointVisibility(selected);
m_streampanel1.setPointVisibility(selected);
}
public void setMicroLayerVisibility(boolean selected) {
m_streampanel0.setMicroLayerVisibility(selected);
m_streampanel1.setMicroLayerVisibility(selected);
}
public void setMacroVisibility(boolean selected) {
m_streampanel0.setMacroLayerVisibility(selected);
m_streampanel1.setMacroLayerVisibility(selected);
}
public void setGroundTruthVisibility(boolean selected) {
m_streampanel0.setGroundTruthLayerVisibility(selected);
m_streampanel1.setGroundTruthLayerVisibility(selected);
}
public void changeCluster(ClusterEvent e) {
if(clusterEvents!=null) clusterEvents.add(e);
System.out.println(e.getType()+": "+e.getMessage());
}
public void exportCSV(String filepath) {
PrintWriter out = null;
try {
if(!filepath.endsWith(".csv"))
filepath+=".csv";
out = new PrintWriter(new BufferedWriter(new FileWriter(filepath)));
String del = ";";
Iterator eventIt = null;
ClusterEvent event = null;
if(clusterEvents!=null && clusterEvents.size() > 0){
eventIt = clusterEvents.iterator();
event = eventIt.next();
}
//raw data
MeasureCollection measurecol[][] = new MeasureCollection[2][];
measurecol[0] = m_measures0;
measurecol[1] = m_measures1;
int numValues = 0;
//header
out.write("Nr"+del);
out.write("Event"+del);
for (int m = 0; m < 2; m++) {
for (int i = 0; i < measurecol[m].length; i++) {
for (int j = 0; j < measurecol[m][i].getNumMeasures(); j++) {
if(measurecol[m][i].isEnabled(j)){
out.write(m+"-"+measurecol[m][i].getName(j)+del);
numValues = measurecol[m][i].getNumberOfValues(j);
}
}
}
}
out.write("\n");
//rows
for (int v = 0; v < numValues; v++){
//Nr
out.write(v+del);
//events
if(event!=null && event.getTimestamp()<=m_stream0_decayHorizon*v){
out.write(event.getType()+del);
if(eventIt!= null && eventIt.hasNext()){
event=eventIt.next();
}
else
event = null;
}
else
out.write(del);
//values
for (int m = 0; m < 2; m++) {
for (int i = 0; i < measurecol[m].length; i++) {
for (int j = 0; j < measurecol[m][i].getNumMeasures(); j++) {
if(measurecol[m][i].isEnabled(j)){
double value = measurecol[m][i].getValue(j, v);
if(Double.isNaN(value))
out.write(del);
else
out.write(value+del);
}
}
}
}
out.write("\n");
}
out.close();
} catch (IOException ex) {
Logger.getLogger(RunVisualizer.class.getName()).log(Level.SEVERE, null, ex);
} finally {
out.close();
}
}
public void weka() {
try{
Class.forName("weka.gui.Logger");
}
catch (Exception e){
m_logPanel.addText("Please add weka.jar to the classpath to use the Weka explorer.");
return;
}
Clustering wekaClustering;
if(m_clusterer0.implementsMicroClusterer() && m_clusterer0.evaluateMicroClusteringOption.isSet())
wekaClustering = micro0;
else
wekaClustering = macro0;
if(wekaClustering == null || wekaClustering.size()==0){
m_logPanel.addText("Empty Clustering");
return;
}
int dims = wekaClustering.get(0).getCenter().length;
FastVector attributes = new FastVector();
for(int i = 0; i < dims; i++)
attributes.addElement( new Attribute("att" + i) );
Instances instances = new Instances("trainset",attributes,0);
for(int c = 0; c < wekaClustering.size(); c++){
Cluster cluster = wekaClustering.get(c);
Instance inst = new DenseInstance(cluster.getWeight(), cluster.getCenter());
inst.setDataset(instances);
instances.add(inst);
}
WekaExplorer explorer = new WekaExplorer(instances);
}
}
© 2015 - 2025 Weber Informatics LLC | Privacy Policy