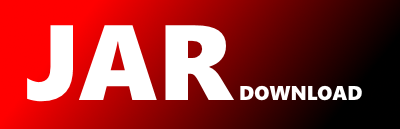
moa.streams.generators.SineGenerator Maven / Gradle / Ivy
Go to download
Show more of this group Show more artifacts with this name
Show all versions of moa Show documentation
Show all versions of moa Show documentation
Massive On-line Analysis is an environment for massive data mining. MOA
provides a framework for data stream mining and includes tools for evaluation
and a collection of machine learning algorithms. Related to the WEKA project,
also written in Java, while scaling to more demanding problems.
The newest version!
/*
* SineGenerator.java
* Copyright (C) 2016 Instituto Federal de Pernambuco
* @author Paulo Gonçalves ([email protected])
*
* This program is free software; you can redistribute it and/or modify
* it under the terms of the GNU General Public License as published by
* the Free Software Foundation; either version 3 of the License, or
* (at your option) any later version.
*
* This program is distributed in the hope that it will be useful,
* but WITHOUT ANY WARRANTY; without even the implied warranty of
* MERCHANTABILITY or FITNESS FOR A PARTICULAR PURPOSE. See the
* GNU General Public License for more details.
*
* You should have received a copy of the GNU General Public License
* along with this program. If not, see .
*
*/
package moa.streams.generators;
import com.github.javacliparser.FlagOption;
import com.github.javacliparser.IntOption;
import com.yahoo.labs.samoa.instances.Attribute;
import com.yahoo.labs.samoa.instances.DenseInstance;
import com.yahoo.labs.samoa.instances.Instance;
import com.yahoo.labs.samoa.instances.Instances;
import com.yahoo.labs.samoa.instances.InstancesHeader;
import java.util.ArrayList;
import java.util.List;
import java.util.Random;
import moa.core.InstanceExample;
import moa.core.ObjectRepository;
import moa.options.AbstractOptionHandler;
import moa.streams.InstanceStream;
import moa.tasks.TaskMonitor;
/**
* 1.SINE1. Abrupt concept drift, noise-free examples. It has two relevant
* attributes. Each attributes has values uniformly distributed in [0; 1]. In
* the first context all points below the curve y = sin(x) are classified as
* positive. After the context change the classification is reversed.
* 2.SINE2. The same two relevant attributes. The classification function is
* y < 0.5 + 0.3 sin(3 * PI * x). After the context change the classification
* is reversed.
* 3.SINIRREL1. Presence of irrelevant attributes. The same classification
* function of SINE1 but the examples have two more random attributes
* with no influence on the classification function.
* 4.SINIRREL2. The same classification function of SINE2 but the examples
* have two more random attributes with no influence on the classification
* function.
* Based on proposal by "Gama, Joao, et al. "Learning with drift
* detection." Advances in artificial intelligence–SBIA 2004. Springer Berlin
* Heidelberg, 2004. 286-295."
*
* @author Paulo Gonçalves ([email protected])
* @version $Revision: 1 $
*/
public class SineGenerator extends AbstractOptionHandler implements
InstanceStream {
public static final int NUM_IRRELEVANT_ATTRIBUTES = 2;
public IntOption instanceRandomSeedOption = new IntOption(
"instanceRandomSeed", 'i',
"Seed for random generation of instances.", 1);
public IntOption functionOption = new IntOption("function", 'f',
"Classification function used, as defined in the original paper.",
1, 1, 4);
public FlagOption suppressIrrelevantAttributesOption = new FlagOption(
"suppressIrrelevantAttributes", 's',
"Reduce the data to only contain 2 relevant numeric attributes.");
public FlagOption balanceClassesOption = new FlagOption("balanceClasses",
'b', "Balance the number of instances of each class.");
protected InstancesHeader streamHeader;
protected Random instanceRandom;
protected boolean nextClassShouldBeZero;
protected interface ClassFunction {
public int determineClass(double x, double y);
}
protected static ClassFunction[] classificationFunctions = {
// Values below the curve y = sin(x) are classified as positive.
new ClassFunction() {
@Override
public int determineClass(double x, double y) {
return (y < Math.sin(x)) ? 0 : 1;
}
},
// Values below the curve y = sin(x) are classified as negative.
new ClassFunction() {
@Override
public int determineClass(double x, double y) {
return (y >= Math.sin(x)) ? 0 : 1;
}
},
// Values below the curve y = 0.5 + 0.3*sin(3*PI*x) are classified as positive.
new ClassFunction() {
@Override
public int determineClass(double x, double y) {
return (y < 0.5 + 0.3 * Math.sin(3 * Math.PI * x)) ? 0 : 1;
}
},
// Values below the curve y = 0.5 + 0.3*sin(3*PI*x) are classified as negative.
new ClassFunction() {
@Override
public int determineClass(double x, double y) {
return (y >= 0.5 + 0.3 * Math.sin(3 * Math.PI * x)) ? 0 : 1;
}
},};
@Override
public void getDescription(StringBuilder sb, int indent) {
}
@Override
public InstancesHeader getHeader() {
return this.streamHeader;
}
@Override
public long estimatedRemainingInstances() {
return -1;
}
@Override
public boolean hasMoreInstances() {
return true;
}
@Override
public InstanceExample nextInstance() {
double a1 = 0, a2 = 0, group = 0;
boolean desiredClassFound = false;
while (!desiredClassFound) {
a1 = this.instanceRandom.nextDouble();
a2 = this.instanceRandom.nextDouble();
group = classificationFunctions[this.functionOption.getValue() - 1].determineClass(a1, a2);
if (!this.balanceClassesOption.isSet()) {
desiredClassFound = true;
} else {
// balance the classes
if ((this.nextClassShouldBeZero && (group == 0))
|| (!this.nextClassShouldBeZero && (group == 1))) {
desiredClassFound = true;
this.nextClassShouldBeZero = !this.nextClassShouldBeZero;
} // else keep searching
}
}
// construct instance
InstancesHeader header = getHeader();
Instance inst = new DenseInstance(header.numAttributes());
inst.setValue(0, a1);
inst.setValue(1, a2);
inst.setDataset(header);
if (!this.suppressIrrelevantAttributesOption.isSet()) {
for (int i = 0; i < NUM_IRRELEVANT_ATTRIBUTES; i++) {
inst.setValue(i + 2, this.instanceRandom.nextDouble());
}
}
inst.setClassValue(group);
return new InstanceExample(inst);
}
@Override
public boolean isRestartable() {
return true;
}
@Override
public void restart() {
this.instanceRandom = new Random(
this.instanceRandomSeedOption.getValue());
this.nextClassShouldBeZero = false;
}
@Override
protected void prepareForUseImpl(TaskMonitor monitor,
ObjectRepository repository) {
ArrayList attributes = new ArrayList();
int numAtts = 2;
if (!this.suppressIrrelevantAttributesOption.isSet()) {
numAtts += NUM_IRRELEVANT_ATTRIBUTES;
}
for (int i = 0; i < numAtts; i++) {
attributes.add(new Attribute("att" + (i + 1)));
}
List classLabels = new ArrayList();
classLabels.add("positive");
classLabels.add("negative");
Attribute classAtt = new Attribute("class", classLabels);
attributes.add(classAtt);
this.streamHeader = new InstancesHeader(new Instances(
getCLICreationString(InstanceStream.class), attributes, 0));
this.streamHeader.setClassIndex(this.streamHeader.numAttributes() - 1);
restart();
}
}
© 2015 - 2025 Weber Informatics LLC | Privacy Policy