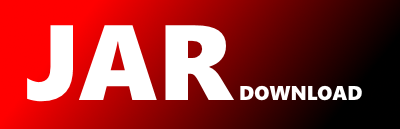
com.clearspring.analytics.stream.quantile.TDigest Maven / Gradle / Ivy
Go to download
Show more of this group Show more artifacts with this name
Show all versions of distributedWekaBase Show documentation
Show all versions of distributedWekaBase Show documentation
This package provides generic configuration class and distributed map/reduce style tasks for Weka
/*
* Licensed to the Apache Software Foundation (ASF) under one or more
* contributor license agreements. See the NOTICE file distributed with
* this work for additional information regarding copyright ownership.
* The ASF licenses this file to You under the Apache License, Version 2.0
* (the "License"); you may not use this file except in compliance with
* the License. You may obtain a copy of the License at
*
* http://www.apache.org/licenses/LICENSE-2.0
*
* Unless required by applicable law or agreed to in writing, software
* distributed under the License is distributed on an "AS IS" BASIS,
* WITHOUT WARRANTIES OR CONDITIONS OF ANY KIND, either express or implied.
* See the License for the specific language governing permissions and
* limitations under the License.
*/
/*
* TDigest
* Taken from the stream-lib project:
* https://github.com/addthis/stream-lib
*
*/
package com.clearspring.analytics.stream.quantile;
import java.nio.ByteBuffer;
import java.util.Collections;
import java.util.Iterator;
import java.util.List;
import java.util.Random;
import java.util.concurrent.atomic.AtomicInteger;
import com.clearspring.analytics.util.Lists;
import com.clearspring.analytics.util.Preconditions;
/**
* Adaptive histogram based on something like streaming k-means crossed with
* Q-digest.
*
* The special characteristics of this algorithm are:
*
* a) smaller summaries than Q-digest
*
* b) works on doubles as well as integers.
*
* c) provides part per million accuracy for extreme quantiles and typically
* <1000 ppm accuracy for middle quantiles
*
* d) fast
*
* e) simple
*
* f) test coverage > 90%
*
* g) easy to adapt for use with map-reduce
*/
public class TDigest {
private final Random gen;
private double compression = 100;
private GroupTree summary = new GroupTree();
private int count = 0;
private boolean recordAllData = false;
/**
* A histogram structure that will record a sketch of a distribution.
*
* @param compression How should accuracy be traded for size? A value of N
* here will give quantile errors almost always less than 3/N with
* considerably smaller errors expected for extreme quantiles.
* Conversely, you should expect to track about 5 N centroids for
* this accuracy.
*/
public TDigest(double compression) {
this(compression, new Random());
}
public TDigest(double compression, Random random) {
this.compression = compression;
gen = random;
}
/**
* Adds a sample to a histogram.
*
* @param x The value to add.
*/
public void add(double x) {
add(x, 1);
}
/**
* Adds a sample to a histogram.
*
* @param x The value to add.
* @param w The weight of this point.
*/
public void add(double x, int w) {
// note that because of a zero id, this will be sorted *before* any existing
// Group with the same mean
Group base = createGroup(x, 0);
add(x, w, base);
}
private void add(double x, int w, Group base) {
Group start = summary.floor(base);
if (start == null) {
start = summary.ceiling(base);
}
if (start == null) {
summary.add(Group.createWeighted(x, w, base.data()));
count = w;
} else {
Iterable neighbors = summary.tailSet(start);
double minDistance = Double.MAX_VALUE;
int lastNeighbor = 0;
int i = summary.headCount(start);
for (Group neighbor : neighbors) {
double z = Math.abs(neighbor.mean() - x);
if (z <= minDistance) {
minDistance = z;
lastNeighbor = i;
} else {
break;
}
i++;
}
Group closest = null;
int sum = summary.headSum(start);
i = summary.headCount(start);
double n = 1;
for (Group neighbor : neighbors) {
if (i > lastNeighbor) {
break;
}
double z = Math.abs(neighbor.mean() - x);
double q = (sum + neighbor.count() / 2.0) / count;
double k = 4 * count * q * (1 - q) / compression;
// this slightly clever selection method improves accuracy with lots of
// repeated points
if (z == minDistance && neighbor.count() + w <= k) {
if (gen.nextDouble() < 1 / n) {
closest = neighbor;
}
n++;
}
sum += neighbor.count();
i++;
}
if (closest == null) {
summary.add(Group.createWeighted(x, w, base.data()));
} else {
summary.remove(closest);
closest.add(x, w, base.data());
summary.add(closest);
}
count += w;
if (summary.size() > 100 * compression) {
// something such as sequential ordering of data points
// has caused a pathological expansion of our summary.
// To fight this, we simply replay the current centroids
// in random order.
// this causes us to forget the diagnostic recording of data points
compress();
}
}
}
public void add(TDigest other) {
List tmp = Lists.newArrayList(other.summary);
Collections.shuffle(tmp, gen);
for (Group group : tmp) {
add(group.mean(), group.count(), group);
}
}
public static TDigest merge(double compression, Iterable subData) {
Preconditions.checkArgument(subData.iterator().hasNext(),
"Can't merge 0 digests");
List elements = Lists.newArrayList(subData);
int n = Math.max(1, elements.size() / 4);
TDigest r = new TDigest(compression, elements.get(0).gen);
if (elements.get(0).recordAllData) {
r.recordAllData();
}
for (int i = 0; i < elements.size(); i += n) {
if (n > 1) {
r.add(merge(compression,
elements.subList(i, Math.min(i + n, elements.size()))));
} else {
r.add(elements.get(i));
}
}
return r;
}
public void compress() {
compress(summary);
}
private void compress(GroupTree other) {
TDigest reduced = new TDigest(compression, gen);
if (recordAllData) {
reduced.recordAllData();
}
List tmp = Lists.newArrayList(other);
Collections.shuffle(tmp, gen);
for (Group group : tmp) {
reduced.add(group.mean(), group.count(), group);
}
summary = reduced.summary;
}
/**
* Returns the number of samples represented in this histogram. If you want to
* know how many centroids are being used, try centroids().size().
*
* @return the number of samples that have been added.
*/
public int size() {
return count;
}
/**
* @param x the value at which the CDF should be evaluated
* @return the approximate fraction of all samples that were less than or
* equal to x.
*/
public double cdf(double x) {
GroupTree values = summary;
if (values.size() == 0) {
return Double.NaN;
} else if (values.size() == 1) {
return x < values.first().mean() ? 0 : 1;
} else {
double r = 0;
// we scan a across the centroids
Iterator it = values.iterator();
Group a = it.next();
// b is the look-ahead to the next centroid
Group b = it.next();
// initially, we set left width equal to right width
double left = (b.mean() - a.mean()) / 2;
double right = left;
// scan to next to last element
while (it.hasNext()) {
if (x < a.mean() + right) {
return (r + a.count()
* interpolate(x, a.mean() - left, a.mean() + right))
/ count;
}
r += a.count();
a = b;
b = it.next();
left = right;
right = (b.mean() - a.mean()) / 2;
}
// for the last element, assume right width is same as left
left = right;
a = b;
if (x < a.mean() + right) {
return (r + a.count()
* interpolate(x, a.mean() - left, a.mean() + right))
/ count;
} else {
return 1;
}
}
}
/**
* @param q The quantile desired. Can be in the range [0,1].
* @return The minimum value x such that we think that the proportion of
* samples is <= x is q.
*/
public double quantile(double q) {
GroupTree values = summary;
Preconditions.checkArgument(values.size() > 1);
Iterator it = values.iterator();
Group center = it.next();
Group leading = it.next();
if (!it.hasNext()) {
// only two centroids because of size limits
// both a and b have to have just a single element
double diff = (leading.mean() - center.mean()) / 2;
if (q > 0.75) {
return leading.mean() + diff * (4 * q - 3);
} else {
return center.mean() + diff * (4 * q - 1);
}
} else {
q *= count;
double right = (leading.mean() - center.mean()) / 2;
// we have nothing else to go on so make left hanging width same as right
// to start
double left = right;
double t = center.count();
while (it.hasNext()) {
if (t + center.count() / 2 >= q) {
// left side of center
return center.mean() - left * 2 * (q - t) / center.count();
} else if (t + leading.count() >= q) {
// right of b but left of the left-most thing beyond
return center.mean() + right * 2.0 * (center.count() - (q - t))
/ center.count();
}
t += center.count();
center = leading;
leading = it.next();
left = right;
right = (leading.mean() - center.mean()) / 2;
}
// ran out of data ... assume final width is symmetrical
center = leading;
left = right;
if (t + center.count() / 2 >= q) {
// left side of center
return center.mean() - left * 2 * (q - t) / center.count();
} else if (t + leading.count() >= q) {
// right of center but left of leading
return center.mean() + right * 2.0 * (center.count() - (q - t))
/ center.count();
} else {
// shouldn't be possible
return 1;
}
}
}
public int centroidCount() {
return summary.size();
}
public Iterable extends Group> centroids() {
return summary;
}
public double compression() {
return compression;
}
/**
* Sets up so that all centroids will record all data assigned to them. For
* testing only, really.
*/
public TDigest recordAllData() {
recordAllData = true;
return this;
}
/**
* Returns an upper bound on the number bytes that will be required to
* represent this histogram.
*/
public int byteSize() {
return 4 + 8 + 4 + summary.size() * 12;
}
/**
* Returns an upper bound on the number of bytes that will be required to
* represent this histogram in the tighter representation.
*/
public int smallByteSize() {
int bound = byteSize();
ByteBuffer buf = ByteBuffer.allocate(bound);
asSmallBytes(buf);
return buf.position();
}
public final static int VERBOSE_ENCODING = 1;
public final static int SMALL_ENCODING = 2;
/**
* Outputs a histogram as bytes using a particularly cheesy encoding.
*/
public void asBytes(ByteBuffer buf) {
buf.putInt(VERBOSE_ENCODING);
buf.putDouble(compression());
buf.putInt(summary.size());
for (Group group : summary) {
buf.putDouble(group.mean());
}
for (Group group : summary) {
buf.putInt(group.count());
}
}
public void asSmallBytes(ByteBuffer buf) {
buf.putInt(SMALL_ENCODING);
buf.putDouble(compression());
buf.putInt(summary.size());
double x = 0;
for (Group group : summary) {
double delta = group.mean() - x;
x = group.mean();
buf.putFloat((float) delta);
}
for (Group group : summary) {
int n = group.count();
encode(buf, n);
}
}
public static void encode(ByteBuffer buf, int n) {
int k = 0;
while (n < 0 || n > 0x7f) {
byte b = (byte) (0x80 | (0x7f & n));
buf.put(b);
n = n >>> 7;
k++;
Preconditions.checkState(k < 6);
}
buf.put((byte) n);
}
public static int decode(ByteBuffer buf) {
int v = buf.get();
int z = 0x7f & v;
int shift = 7;
while ((v & 0x80) != 0) {
Preconditions.checkState(shift <= 28);
v = buf.get();
z += (v & 0x7f) << shift;
shift += 7;
}
return z;
}
/**
* Reads a histogram from a byte buffer
*
* @return The new histogram structure
*/
public static TDigest fromBytes(ByteBuffer buf) {
int encoding = buf.getInt();
if (encoding == VERBOSE_ENCODING) {
double compression = buf.getDouble();
TDigest r = new TDigest(compression);
int n = buf.getInt();
double[] means = new double[n];
for (int i = 0; i < n; i++) {
means[i] = buf.getDouble();
}
for (int i = 0; i < n; i++) {
r.add(means[i], buf.getInt());
}
return r;
} else if (encoding == SMALL_ENCODING) {
double compression = buf.getDouble();
TDigest r = new TDigest(compression);
int n = buf.getInt();
double[] means = new double[n];
double x = 0;
for (int i = 0; i < n; i++) {
double delta = buf.getFloat();
x += delta;
means[i] = x;
}
for (int i = 0; i < n; i++) {
int z = decode(buf);
r.add(means[i], z);
}
return r;
} else {
throw new IllegalStateException("Invalid format for serialized histogram");
}
}
private Group createGroup(double mean, int id) {
return new Group(mean, id, recordAllData);
}
private double interpolate(double x, double x0, double x1) {
return (x - x0) / (x1 - x0);
}
public static class Group implements Comparable {
private static final AtomicInteger uniqueCount = new AtomicInteger(1);
private double centroid = 0;
private int count = 0;
private int id;
private List actualData = null;
private Group(boolean record) {
id = uniqueCount.incrementAndGet();
if (record) {
actualData = Lists.newArrayList();
}
}
public Group(double x) {
this(false);
start(x, uniqueCount.getAndIncrement());
}
public Group(double x, int id) {
this(false);
start(x, id);
}
public Group(double x, int id, boolean record) {
this(record);
start(x, id);
}
private void start(double x, int id) {
this.id = id;
add(x, 1);
}
public void add(double x, int w) {
if (actualData != null) {
actualData.add(x);
}
count += w;
centroid += w * (x - centroid) / count;
}
public double mean() {
return centroid;
}
public int count() {
return count;
}
public int id() {
return id;
}
@Override
public String toString() {
return "Group{" +
"centroid=" + centroid +
", count=" + count +
'}';
}
@Override
public int hashCode() {
return id;
}
@Override
public int compareTo(Group o) {
int r = Double.compare(centroid, o.centroid);
if (r == 0) {
r = id - o.id;
}
return r;
}
public Iterable extends Double> data() {
return actualData;
}
public static Group createWeighted(double x, int w,
Iterable extends Double> data) {
Group r = new Group(data != null);
r.add(x, w, data);
return r;
}
private void add(double x, int w, Iterable extends Double> data) {
if (actualData != null) {
if (data != null) {
for (Double old : data) {
actualData.add(old);
}
} else {
actualData.add(x);
}
}
count += w;
centroid += w * (x - centroid) / count;
}
}
}
© 2015 - 2025 Weber Informatics LLC | Privacy Policy