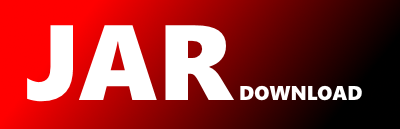
weka.distributed.CorrelationMatrixRowReduceTask Maven / Gradle / Ivy
Go to download
Show more of this group Show more artifacts with this name
Show all versions of distributedWekaBase Show documentation
Show all versions of distributedWekaBase Show documentation
This package provides generic configuration class and distributed map/reduce style tasks for Weka
/*
* This program is free software: you can redistribute it and/or modify
* it under the terms of the GNU General Public License as published by
* the Free Software Foundation, either version 3 of the License, or
* (at your option) any later version.
*
* This program is distributed in the hope that it will be useful,
* but WITHOUT ANY WARRANTY; without even the implied warranty of
* MERCHANTABILITY or FITNESS FOR A PARTICULAR PURPOSE. See the
* GNU General Public License for more details.
*
* You should have received a copy of the GNU General Public License
* along with this program. If not, see .
*/
/*
* CorrelationMatrixMapTask.java
* Copyright (C) 2013 University of Waikato, Hamilton, New Zealand
*
*/
package weka.distributed;
import java.awt.Image;
import java.awt.image.BufferedImage;
import java.io.IOException;
import java.io.OutputStream;
import java.io.Serializable;
import java.util.List;
import javax.imageio.ImageIO;
import org.tc33.jheatchart.HeatChart;
import weka.core.Attribute;
import weka.core.Instances;
import weka.core.Utils;
import weka.core.matrix.Matrix;
import weka.core.stats.ArffSummaryNumericMetric;
import weka.filters.Filter;
import weka.filters.unsupervised.attribute.Remove;
/**
* A reduce task that sums the incoming partial covariance sums for a given row
* of the matrix and then computes the final correlation/ covariance values.
*
* @author Mark Hall (mhall{[at]}pentaho{[dot]}com)
* @version $Revision: 10926 $
*/
public class CorrelationMatrixRowReduceTask implements Serializable {
/** For serialization */
private static final long serialVersionUID = -2314677791571204717L;
public static Image getHeatMapForMatrix(Matrix matrix,
List rowAttNames) {
double[][] m = matrix.getArray();
// generate the heat map
// need to reverse the order of the rows
double[][] mm = new double[m.length][];
for (int i = 0; i < m.length; i++) {
mm[m.length - 1 - i] = m[i];
}
String[] xLabels = new String[rowAttNames.size()];
String[] yLabels = new String[rowAttNames.size()];
for (int i = 0; i < rowAttNames.size(); i++) {
xLabels[i] = rowAttNames.get(i);
yLabels[rowAttNames.size() - 1 - i] = rowAttNames.get(i);
}
HeatChart map = new HeatChart(mm, true);
map.setTitle("Correlation matrix heat map");
map.setCellSize(new java.awt.Dimension(30, 30));
map.setHighValueColour(java.awt.Color.RED);
map.setLowValueColour(java.awt.Color.BLUE);
map.setXValues(xLabels);
map.setYValues(yLabels);
return map.getChartImage();
}
public static void writeHeatMapImage(Image map, OutputStream dest)
throws IOException {
ImageIO.write((BufferedImage) map, "png", dest);
dest.flush();
dest.close();
}
/**
* Aggregate a list of partial rows of the matrix.
*
* @param matrixRowNumber the index of the row in the matrix to be aggregated
* @param toAggregate a list rows to be aggregated
* @param coOccurrencesToAgg a list of co-occurrence counts to aggregate (will
* be null if missings have been replaced with means)
* @param headerWithSummaryAtts the header of the data (including summary
* attributes)
* @param missingsWereReplacedWithMeans true if missing values were replaced
* by means in the map tasks
* @param covarianceInsteadOfCorrelation final matrix is to be a covariance
* one rather than correlation
* @param deleteClassIfSet true if the class attribute is to be deleted (if
* set)
* @return the aggregated row
* @throws DistributedWekaException if a problem occurs
*/
public double[] aggregate(int matrixRowNumber, List toAggregate,
List coOccurrencesToAgg, Instances headerWithSummaryAtts,
boolean missingsWereReplacedWithMeans,
boolean covarianceInsteadOfCorrelation, boolean deleteClassIfSet)
throws DistributedWekaException {
StringBuilder rem = new StringBuilder();
Instances trainingHeader =
CSVToARFFHeaderReduceTask.stripSummaryAtts(headerWithSummaryAtts);
if (trainingHeader.classIndex() >= 0 && deleteClassIfSet) {
rem.append("" + (trainingHeader.classIndex() + 1)).append(",");
}
// remove all nominal attributes
for (int i = 0; i < trainingHeader.numAttributes(); i++) {
if (!trainingHeader.attribute(i).isNumeric()) {
rem.append("" + (i + 1)).append(",");
}
}
if (rem.length() > 0) {
Remove remove = new Remove();
rem.deleteCharAt(rem.length() - 1); // remove the trailing ,
String attIndices = rem.toString();
remove.setAttributeIndices(attIndices);
remove.setInvertSelection(false);
try {
remove.setInputFormat(trainingHeader);
trainingHeader = Filter.useFilter(trainingHeader, remove);
} catch (Exception ex) {
throw new DistributedWekaException(ex);
}
}
Attribute origCorrespondingToRow =
trainingHeader.attribute(matrixRowNumber);
Attribute correspondingSummaryAtt =
headerWithSummaryAtts
.attribute(CSVToARFFHeaderMapTask.ARFF_SUMMARY_ATTRIBUTE_PREFIX
+ origCorrespondingToRow.name());
if (correspondingSummaryAtt == null) {
throw new DistributedWekaException(
"Was unable to find the summary stats attribute for "
+ "original attribute '" + origCorrespondingToRow.name()
+ "' corresponding to matrix row number: " + matrixRowNumber);
}
if (toAggregate.size() == 0) {
throw new DistributedWekaException("Nothing to aggregate!");
}
double[] aggregated = toAggregate.get(0).clone();
for (int i = 1; i < toAggregate.size(); i++) {
double[] toAgg = toAggregate.get(i);
for (int j = 0; j < toAgg.length; j++) {
aggregated[j] += toAgg[j];
}
}
int[] coOccAgg = null;
if (!missingsWereReplacedWithMeans) {
coOccAgg = coOccurrencesToAgg.get(0).clone();
for (int i = 1; i < coOccurrencesToAgg.size(); i++) {
int[] toAgg = coOccurrencesToAgg.get(i);
for (int j = 0; j < toAgg.length; j++) {
coOccAgg[j] += toAgg[j];
}
}
}
// correlation or covariance?
double[] statsForRowAtt =
CSVToARFFHeaderReduceTask.attributeToStatsArray(correspondingSummaryAtt);
for (int i = 0; i < aggregated.length; i++) {
Attribute attI = trainingHeader.attribute(i);
Attribute currespondingSummaryI =
headerWithSummaryAtts
.attribute(CSVToARFFHeaderMapTask.ARFF_SUMMARY_ATTRIBUTE_PREFIX
+ attI.name());
double[] statsForI =
CSVToARFFHeaderReduceTask.attributeToStatsArray(currespondingSummaryI);
// if missings were replaced then sum is divided by the total count (i.e.
// count + #missing); otherwise use the co-occurrence count for rowAtt,
// col i
double denom =
missingsWereReplacedWithMeans ? statsForRowAtt[ArffSummaryNumericMetric.COUNT
.ordinal()]
+ statsForRowAtt[ArffSummaryNumericMetric.MISSING.ordinal()]
: coOccAgg[i];
// Math
// .min(statsForRowAtt[ArffSummaryNumericMetric.COUNT.ordinal()],
// statsForI[ArffSummaryNumericMetric.COUNT.ordinal()]);
if (covarianceInsteadOfCorrelation) {
if (denom > 1) {
aggregated[i] /= (denom - 1);
} else {
if (denom == 1) {
aggregated[i] = Double.POSITIVE_INFINITY;
} else {
aggregated[i] = Utils.missingValue(); // never co-occurred
}
}
} else {
if (matrixRowNumber == i || denom <= 1) {
if (missingsWereReplacedWithMeans) {
aggregated[i] = 1.0;
} else {
aggregated[i] = Utils.missingValue(); // never co-occurred
}
} else {
double sR = statsForRowAtt[ArffSummaryNumericMetric.STDDEV.ordinal()];
double sI = statsForI[ArffSummaryNumericMetric.STDDEV.ordinal()];
if (sR * sI > 0.0) {
aggregated[i] = aggregated[i] / ((denom - 1) * sR * sI);
}
}
}
}
return aggregated;
}
}
© 2015 - 2025 Weber Informatics LLC | Privacy Policy