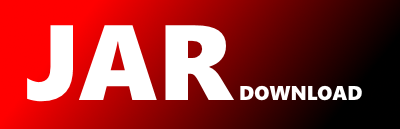
weka.clusterers.forOPTICSAndDBScan.DataObjects.ManhattanDataObject Maven / Gradle / Ivy
Go to download
Show more of this group Show more artifacts with this name
Show all versions of weka-stable Show documentation
Show all versions of weka-stable Show documentation
The Waikato Environment for Knowledge Analysis (WEKA), a machine
learning workbench. This is the stable version. Apart from bugfixes, this version
does not receive any other updates.
/*
* This program is free software: you can redistribute it and/or modify
* it under the terms of the GNU General Public License as published by
* the Free Software Foundation, either version 3 of the License, or
* (at your option) any later version.
*
* This program is distributed in the hope that it will be useful,
* but WITHOUT ANY WARRANTY; without even the implied warranty of
* MERCHANTABILITY or FITNESS FOR A PARTICULAR PURPOSE. See the
* GNU General Public License for more details.
*
* You should have received a copy of the GNU General Public License
* along with this program. If not, see .
*/
/*
* Copyright (C) 2004
* & Matthias Schubert ([email protected])
* & Zhanna Melnikova-Albrecht ([email protected])
* & Rainer Holzmann ([email protected])
*/
package weka.clusterers.forOPTICSAndDBScan.DataObjects;
import java.io.Serializable;
import weka.clusterers.forOPTICSAndDBScan.Databases.Database;
import weka.core.Attribute;
import weka.core.Instance;
import weka.core.RevisionHandler;
import weka.core.RevisionUtils;
import weka.core.Utils;
/**
*
* ManhattanDataObject.java
* Authors: Rainer Holzmann, Zhanna Melnikova-Albrecht, Matthias Schubert
* Date: Aug 19, 2004
* Time: 5:50:22 PM
* $ Revision 1.4 $
*
*
* @author Matthias Schubert ([email protected])
* @author Zhanna Melnikova-Albrecht ([email protected])
* @author Rainer Holzmann ([email protected])
* @version $Revision: 9420 $
*/
public class ManhattanDataObject implements DataObject, Serializable,
RevisionHandler {
/** for serialization */
private static final long serialVersionUID = -3417720553766544582L;
/**
* Holds the original instance
*/
private final Instance instance;
/**
* Holds the (unique) key that is associated with this DataObject
*/
private String key;
/**
* Holds the ID of the cluster, to which this DataObject is assigned
*/
private int clusterID;
/**
* Holds the status for this DataObject (true, if it has been processed, else
* false)
*/
private boolean processed;
/**
* Holds the coreDistance for this DataObject
*/
private double c_dist;
/**
* Holds the reachabilityDistance for this DataObject
*/
private double r_dist;
/**
* Holds the database, that is the keeper of this DataObject
*/
private final Database database;
// *****************************************************************************************************************
// constructors
// *****************************************************************************************************************
/**
* Constructs a new DataObject. The original instance is kept as
* instance-variable
*
* @param originalInstance the original instance
*/
public ManhattanDataObject(Instance originalInstance, String key,
Database database) {
this.database = database;
this.key = key;
instance = originalInstance;
clusterID = DataObject.UNCLASSIFIED;
processed = false;
c_dist = DataObject.UNDEFINED;
r_dist = DataObject.UNDEFINED;
}
// *****************************************************************************************************************
// methods
// *****************************************************************************************************************
/**
* Compares two DataObjects in respect to their attribute-values
*
* @param dataObject The DataObject, that is compared with this.dataObject;
* now assumed to be of the same type and with the same structure
* @return Returns true, if the DataObjects correspond in each value, else
* returns false
*/
public boolean equals(DataObject dataObject) {
if (this == dataObject)
return true;
for (int i = 0; i < getInstance().numValues(); i++) {
double i_value_Instance_1 = getInstance().valueSparse(i);
double i_value_Instance_2 = dataObject.getInstance().valueSparse(i);
if (i_value_Instance_1 != i_value_Instance_2)
return false;
}
return true;
}
/**
* Calculates the manhattan-distance between dataObject and this.dataObject
*
* @param dataObject The DataObject, that is used for distance-calculation
* with this.dataObject now assumed to be of the same type and with
* the same structure
* @return double-value The manhattan-distance between dataObject and
* this.dataObject NaN, if the computation could not be performed
*/
public double distance(DataObject dataObject) {
double dist = 0.0;
for (int i = 0; i < getInstance().numValues(); i++) {
double cDistance = computeDistance(getInstance().index(i), getInstance()
.valueSparse(i), dataObject.getInstance().valueSparse(i));
dist += Math.abs(cDistance);
}
return dist;
}
/**
* Performs manhattan-distance-calculation between two given values
*
* @param index of the attribute within the DataObject's instance
* @param v value_1
* @param v1 value_2
* @return double norm-distance between value_1 and value_2
*/
private double computeDistance(int index, double v, double v1) {
switch (getInstance().attribute(index).type()) {
case Attribute.NOMINAL:
return (Instance.isMissingValue(v) || Instance.isMissingValue(v1) || ((int) v != (int) v1)) ? 1
: 0;
case Attribute.NUMERIC:
if (Instance.isMissingValue(v) || Instance.isMissingValue(v1)) {
if (Instance.isMissingValue(v) && Instance.isMissingValue(v1))
return 1;
else {
return (Instance.isMissingValue(v)) ? norm(v1, index)
: norm(v, index);
}
} else
return norm(v, index) - norm(v1, index);
default:
return 0;
}
}
/**
* Normalizes a given value of a numeric attribute.
*
* @param x the value to be normalized
* @param i the attribute's index
*/
private double norm(double x, int i) {
if (Double.isNaN(database.getAttributeMinValues()[i])
|| Utils.eq(database.getAttributeMaxValues()[i],
database.getAttributeMinValues()[i])) {
return 0;
} else {
return (x - database.getAttributeMinValues()[i])
/ (database.getAttributeMaxValues()[i] - database
.getAttributeMinValues()[i]);
}
}
/**
* Returns the original instance
*
* @return originalInstance
*/
public Instance getInstance() {
return instance;
}
/**
* Returns the key for this DataObject
*
* @return key
*/
public String getKey() {
return key;
}
/**
* Sets the key for this DataObject
*
* @param key The key is represented as string
*/
public void setKey(String key) {
this.key = key;
}
/**
* Sets the clusterID (cluster), to which this DataObject belongs to
*
* @param clusterID Number of the Cluster
*/
public void setClusterLabel(int clusterID) {
this.clusterID = clusterID;
}
/**
* Returns the clusterID, to which this DataObject belongs to
*
* @return clusterID
*/
public int getClusterLabel() {
return clusterID;
}
/**
* Marks this dataObject as processed
*
* @param processed True, if the DataObject has been already processed, false
* else
*/
public void setProcessed(boolean processed) {
this.processed = processed;
}
/**
* Gives information about the status of a dataObject
*
* @return True, if this dataObject has been processed, else false
*/
public boolean isProcessed() {
return processed;
}
/**
* Sets a new coreDistance for this dataObject
*
* @param c_dist coreDistance
*/
public void setCoreDistance(double c_dist) {
this.c_dist = c_dist;
}
/**
* Returns the coreDistance for this dataObject
*
* @return coreDistance
*/
public double getCoreDistance() {
return c_dist;
}
/**
* Sets a new reachability-distance for this dataObject
*/
public void setReachabilityDistance(double r_dist) {
this.r_dist = r_dist;
}
/**
* Returns the reachabilityDistance for this dataObject
*/
public double getReachabilityDistance() {
return r_dist;
}
@Override
public String toString() {
return instance.toString();
}
/**
* Returns the revision string.
*
* @return the revision
*/
public String getRevision() {
return RevisionUtils.extract("$Revision: 9420 $");
}
}
© 2015 - 2025 Weber Informatics LLC | Privacy Policy