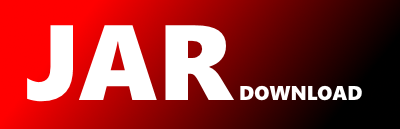
weka.classifiers.bayes.DMNBtext Maven / Gradle / Ivy
Go to download
Show more of this group Show more artifacts with this name
Show all versions of weka-stable Show documentation
Show all versions of weka-stable Show documentation
The Waikato Environment for Knowledge Analysis (WEKA), a machine
learning workbench. This is the stable version. Apart from bugfixes, this version
does not receive any other updates.
/*
* This program is free software; you can redistribute it and/or modify
* it under the terms of the GNU General Public License as published by
* the Free Software Foundation; either version 2 of the License, or
* (at your option) any later version.
*
* This program is distributed in the hope that it will be useful,
* but WITHOUT ANY WARRANTY; without even the implied warranty of
* MERCHANTABILITY or FITNESS FOR A PARTICULAR PURPOSE. See the
* GNU General Public License for more details.
*
* You should have received a copy of the GNU General Public License
* along with this program; if not, write to the Free Software
* Foundation, Inc., 675 Mass Ave, Cambridge, MA 02139, USA.
*/
/*
* Discriminative Multinomial Naive Bayes for Text Classification
* Copyright (C) 2008 Jiang Su
*/
package weka.classifiers.bayes;
import weka.classifiers.Classifier;
import weka.core.Instance;
import weka.core.Instances;
import weka.core.Option;
import weka.core.TechnicalInformation;
import weka.core.TechnicalInformationHandler;
import weka.core.Utils;
import weka.core.WeightedInstancesHandler;
import weka.core.Capabilities.Capability;
import weka.core.TechnicalInformation.Field;
import weka.core.TechnicalInformation.Type;
import weka.classifiers.UpdateableClassifier;
import java.util.*;
import java.io.Serializable;
import weka.core.Capabilities;
import weka.core.OptionHandler;
/**
* Class for building and using a Discriminative Multinomial Naive Bayes classifier. For more information see,
*
* Jiang Su,Harry Zhang,Charles X. Ling,Stan Matwin: Discriminative Parameter Learning for Bayesian Networks. In: ICML 2008', 2008.
*
* The core equation for this classifier:
*
* P[Ci|D] = (P[D|Ci] x P[Ci]) / P[D] (Bayes rule)
*
* where Ci is class i and D is a document.
*
*
* BibTeX:
*
* @inproceedings{JiangSu2008,
* author = {Jiang Su,Harry Zhang,Charles X. Ling,Stan Matwin},
* booktitle = {ICML 2008'},
* title = {Discriminative Parameter Learning for Bayesian Networks},
* year = {2008}
* }
*
*
*
* Valid options are:
*
* -I <iterations>
* The number of iterations that the classifier
* will scan the training data (default = 1)
*
* -M
* Use the frequency information in data
*
*
* @author Jiang Su ([email protected]) 2008
* @version $Revision: 6363 $
*/
public class DMNBtext extends Classifier
implements OptionHandler, WeightedInstancesHandler,
TechnicalInformationHandler, UpdateableClassifier {
/** for serialization */
static final long serialVersionUID = 5932177450183457085L;
/** The number of iterations. */
protected int m_NumIterations = 1;
protected boolean m_MultinomialWord = false;
int m_numClasses=-1;
protected Instances m_headerInfo;
DNBBinary[] m_binaryClassifiers = null;
/**
* Returns a string describing this classifier
* @return a description of the classifier suitable for
* displaying in the explorer/experimenter gui
*/
public String globalInfo() {
return
"Class for building and using a Discriminative Multinomial Naive Bayes classifier. "
+ "For more information see,\n\n"
+ getTechnicalInformation().toString() + "\n\n"
+ "The core equation for this classifier:\n\n"
+ "P[Ci|D] = (P[D|Ci] x P[Ci]) / P[D] (Bayes rule)\n\n"
+ "where Ci is class i and D is a document.";
}
/**
* Returns an instance of a TechnicalInformation object, containing
* detailed information about the technical background of this class,
* e.g., paper reference or book this class is based on.
*
* @return the technical information about this class
*/
public TechnicalInformation getTechnicalInformation() {
TechnicalInformation result;
result = new TechnicalInformation(Type.INPROCEEDINGS);
result.setValue(Field.AUTHOR, "Jiang Su,Harry Zhang,Charles X. Ling,Stan Matwin");
result.setValue(Field.YEAR, "2008");
result.setValue(Field.TITLE, "Discriminative Parameter Learning for Bayesian Networks");
result.setValue(Field.BOOKTITLE, "ICML 2008'");
return result;
}
/**
* Returns default capabilities of the classifier.
*
* @return the capabilities of this classifier
*/
public Capabilities getCapabilities() {
Capabilities result = super.getCapabilities();
result.disableAll();
// attributes
result.enable(Capability.NUMERIC_ATTRIBUTES);
// class
result.enable(Capability.NOMINAL_CLASS);
result.enable(Capability.MISSING_CLASS_VALUES);
// instances
result.setMinimumNumberInstances(0);
return result;
}
/**
* Generates the classifier.
*
* @param instances set of instances serving as training data
* @exception Exception if the classifier has not been generated successfully
*/
public void buildClassifier(Instances data) throws Exception {
// can classifier handle the data?
getCapabilities().testWithFail(data);
// remove instances with missing class
Instances instances = new Instances(data);
instances.deleteWithMissingClass();
m_binaryClassifiers = new DNBBinary[instances.numClasses()];
m_numClasses=instances.numClasses();
m_headerInfo = new Instances(instances, 0);
for (int i = 0; i < instances.numClasses(); i++) {
m_binaryClassifiers[i] = new DNBBinary();
m_binaryClassifiers[i].setTargetClass(i);
m_binaryClassifiers[i].initClassifier(instances);
}
if (instances.numInstances() == 0)
return;
//Iterative update
Random random = new Random();
for (int it = 0; it < m_NumIterations; it++) {
for (int i = 0; i < instances.numInstances(); i++) {
updateClassifier(instances.instance(i));
}
}
// Utils.normalize(m_oldClassDis);
// Utils.normalize(m_ClassDis);
// m_originalPositive = m_oldClassDis[0];
// m_positive = m_ClassDis[0];
}
/**
* Updates the classifier with the given instance.
*
* @param instance the new training instance to include in the model
* @exception Exception if the instance could not be incorporated in
* the model.
*/
public void updateClassifier(Instance instance) throws Exception {
if (m_numClasses == 2) {
m_binaryClassifiers[0].updateClassifier(instance);
} else {
for (int i = 0; i < instance.numClasses(); i++)
m_binaryClassifiers[i].updateClassifier(instance);
}
}
/**
* Calculates the class membership probabilities for the given test
* instance.
*
* @param instance the instance to be classified
* @return predicted class probability distribution
* @exception Exception if there is a problem generating the prediction
*/
public double[] distributionForInstance(Instance instance) throws Exception {
if (m_numClasses == 2) {
// System.out.println(m_binaryClassifiers[0].getProbForTargetClass(instance));
return m_binaryClassifiers[0].distributionForInstance(instance);
}
double[] logDocGivenClass = new double[instance.numClasses()];
for (int i = 0; i < m_numClasses; i++)
logDocGivenClass[i] = m_binaryClassifiers[i].getLogProbForTargetClass(instance);
double max = logDocGivenClass[Utils.maxIndex(logDocGivenClass)];
for(int i = 0; i listOptions() {
Vector
© 2015 - 2025 Weber Informatics LLC | Privacy Policy