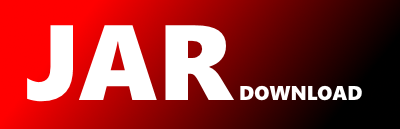
weka.classifiers.sklearn.ScikitLearnClassifier Maven / Gradle / Ivy
Go to download
Show more of this group Show more artifacts with this name
Show all versions of wekaPython Show documentation
Show all versions of wekaPython Show documentation
Integration with CPython for Weka. Python version 2.7.x or higher is required. Also requires the following packages to be installed in python: numpy, pandas, matplotlib and scikit-learn. This package provides a wrapper classifier and clusterer that, between them, cover 60+ scikit-learn algorithms. It also provides a general scripting step for the Knowlege Flow along with scripting plugin environments for the Explorer and Knowledge Flow.
The newest version!
/*
* This program is free software: you can redistribute it and/or modify
* it under the terms of the GNU General Public License as published by
* the Free Software Foundation, either version 3 of the License, or
* (at your option) any later version.
*
* This program is distributed in the hope that it will be useful,
* but WITHOUT ANY WARRANTY; without even the implied warranty of
* MERCHANTABILITY or FITNESS FOR A PARTICULAR PURPOSE. See the
* GNU General Public License for more details.
*
* You should have received a copy of the GNU General Public License
* along with this program. If not, see .
*/
/*
* ScikitLearnClassifier.java
* Copyright (C) 2015 University of Waikato, Hamilton, New Zealand
*
*/
package weka.classifiers.sklearn;
import java.util.List;
import weka.classifiers.AbstractClassifier;
import weka.classifiers.rules.ZeroR;
import weka.core.Attribute;
import weka.core.AttributeStats;
import weka.core.BatchPredictor;
import weka.core.Capabilities;
import weka.core.CapabilitiesHandler;
import weka.core.Instance;
import weka.core.Instances;
import weka.core.OptionMetadata;
import weka.core.SelectedTag;
import weka.core.Tag;
import weka.core.Utils;
import weka.core.WekaException;
import weka.filters.Filter;
import weka.filters.unsupervised.attribute.Remove;
import weka.filters.unsupervised.attribute.ReplaceMissingValues;
import weka.python.PythonSession;
/**
* Wrapper classifier for classifiers and regressors implemented in the
* scikit-learn Python package.
*
* @author Mark Hall (mhall{[at]}pentaho{[dot]}com)
* @version $Revision: $
*/
public class ScikitLearnClassifier extends AbstractClassifier
implements BatchPredictor, CapabilitiesHandler {
protected static final String TRAINING_DATA_ID = "scikit_classifier_training";
protected static final String TEST_DATA_ID = "scikit_classifier_test";
protected static final String MODEL_ID = "weka_scikit_learner";
/** For serialization */
private static final long serialVersionUID = -6212485658537766441L;
/** Holds info on the different learners available */
public static enum Learner {
DecisionTreeClassifier("tree", true, false, true, false,
"\tclass_weight=None, criterion='gini', max_depth=None,\n"
+ "\tmax_features=None, max_leaf_nodes=None, min_samples_leaf=1,\n"
+ "\tmin_samples_split=2, min_weight_fraction_leaf=0.0,\n"
+ "\trandom_state=None, splitter='best'"),
DecisionTreeRegressor("tree", false, true, false, false,
"\tcriterion='mse', max_depth=None, max_features=None,\n"
+ "\tmax_leaf_nodes=None, min_samples_leaf=1, min_samples_split=2,\n"
+ "\tmin_weight_fraction_leaf=0.0, random_state=None,\n"
+ "\tsplitter='best'"),
GaussianNB("naive_bayes", true, false, true, false, ""),
MultinomialNB("naive_bayes", true, false, true, false,
"alpha=1.0, class_prior=None, fit_prior=True"),
BernoulliNB("naive_bayes", true, false, true, false,
"alpha=1.0, binarize=0.0, class_prior=None, fit_prior=True"),
LDA("lda", true, false, true, false,
"\tn_components=None, priors=None, shrinkage=None, solver='svd',\n"
+ "\tstore_covariance=False, tol=0.0001"),
QDA("qda", true, false, true, false, "\tpriors=None, reg_param=0.0"),
LogisticRegression("linear_model", true, false, true, false,
"\tC=1.0, class_weight=None, dual=False, fit_intercept=True,\n"
+ "\tintercept_scaling=1, max_iter=100, multi_class='ovr',\n"
+ "\tpenalty='l2', random_state=None, solver='liblinear', tol=0.0001,\n"
+ "\tverbose=0"),
LogisticRegressionCV("linear_model", true, false, true, false,
"\tCs=10, class_weight=None, cv=None, dual=False,\n"
+ "\tfit_intercept=True, intercept_scaling=1.0, max_iter=100,\n"
+ "\tmulti_class='ovr', n_jobs=1, penalty='l2', refit=True,\n"
+ "\tscoring=None, solver='lbfgs', tol=0.0001, verbose=0"),
LinearRegression("linear_model", false, true, false, false,
"\tcopy_X=True, fit_intercept=True, n_jobs=1, normalize=False"),
ARDRegression("linear_model", false, true, false, false,
"\talpha_1=1e-06, alpha_2=1e-06, compute_score=False, copy_X=True,\n"
+ "\tfit_intercept=True, lambda_1=1e-06, lambda_2=1e-06, n_iter=300,\n"
+ "\tnormalize=False, threshold_lambda=10000.0, tol=0.001, verbose=False"),
BayesianRidge("linear_model", false, true, false, false,
"\talpha_1=1e-06, alpha_2=1e-06, compute_score=False, copy_X=True,\n"
+ "\tfit_intercept=True, lambda_1=1e-06, lambda_2=1e-06, n_iter=300,\n"
+ "\tnormalize=False, tol=0.001, verbose=False"),
ElasticNet("linear_model", false, true, false, false,
"\talpha=1.0, copy_X=True, fit_intercept=True, l1_ratio=0.5,\n"
+ "\tmax_iter=1000, normalize=False, positive=False, precompute=False,\n"
+ "\trandom_state=None, selection='cyclic', tol=0.0001, warm_start=False"),
Lars("linear_model", false, true, false, false,
"\tcopy_X=True, eps=2.2204460492503131e-16, fit_intercept=True,\n"
+ "\tfit_path=True, n_nonzero_coefs=500, normalize=True, precompute='auto',\n"
+ "\tverbose=False"),
LarsCV("linear_model", false, true, false, false,
"\tcopy_X=True, cv=None, eps=2.2204460492503131e-16, fit_intercept=True,\n"
+ "\tmax_iter=500, max_n_alphas=1000, n_jobs=1, normalize=True,\n"
+ "\tprecompute='auto', verbose=False"),
Lasso("linear_model", false, true, false, false,
"\talpha=1.0, copy_X=True, fit_intercept=True, max_iter=1000,\n"
+ "\tnormalize=False, positive=False, precompute=False, random_state=None,\n"
+ "\tselection='cyclic', tol=0.0001, warm_start=False"),
LassoCV("linear_model", false, true, false, false,
"\talphas=None, copy_X=True, cv=None, eps=0.001, fit_intercept=True,\n"
+ "\tmax_iter=1000, n_alphas=100, n_jobs=1, normalize=False, positive=False,\n"
+ "\tprecompute='auto', random_state=None, selection='cyclic', tol=0.0001,\n"
+ "\tverbose=False"),
LassoLars("linear_model", false, true, false, false,
"\talpha=1.0, copy_X=True, eps=2.2204460492503131e-16,\n"
+ "\tfit_intercept=True, fit_path=True, max_iter=500, normalize=True,\n"
+ "\tprecompute='auto', verbose=False"),
LassoLarsCV("linear_model", false, true, false, false,
"\tcopy_X=True, cv=None, eps=2.2204460492503131e-16,\n"
+ "\tfit_intercept=True, max_iter=500, max_n_alphas=1000, n_jobs=1,\n"
+ "\tnormalize=True, precompute='auto', verbose=False"),
LassoLarsIC("linear_model", false, true, false, false,
"\tcopy_X=True, criterion='aic', eps=2.2204460492503131e-16,\n"
+ "\tfit_intercept=True, max_iter=500, normalize=True, precompute='auto',\n"
+ "\tverbose=False"),
MLPClassifier("neural_network", true, false, true, false,
"\thidden_layer_sizes=(100,), " + "activation='relu', solver='adam',\n"
+ "\talpha=0.0001, batch_size='auto', learning_rate='constant',\n"
+ "\tlearning_rate_init=0.001, power_t=0.5, max_iter=200,\n"
+ "\tshuffle=True, random_state=None, tol=0.0001, verbose=False,\n"
+ "\twarm_start=False, momentum=0.9, nesterovs_momentum=True,\n"
+ "\tearly_stopping=False, validation_fraction=0.1, beta_1=0.9,\n"
+ "\tbeta_2=0.999, epsilon=1e-08"),
MLPRegressor("neural_network", false, true, false, false,
"\thidden_layer_sizes=(100,), " + "activation='relu', solver='adam',\n"
+ "\talpha=0.0001, batch_size='auto', learning_rate='constant',\n"
+ "\tlearning_rate_init=0.001, power_t=0.5, max_iter=200,\n"
+ "\tshuffle=True, random_state=None, tol=0.0001, verbose=False,\n"
+ "\twarm_start=False, momentum=0.9, nesterovs_momentum=True,\n"
+ "\tearly_stopping=False, validation_fraction=0.1, beta_1=0.9,\n"
+ "\tbeta_2=0.999, epsilon=1e-08"),
OrthogonalMatchingPursuit("linear_model", false, true, false, false,
"\tfit_intercept=True, n_nonzero_coefs=None,\n"
+ "\tnormalize=True, precompute='auto', tol=None"),
OrthogonalMatchingPursuitCV("linear_model", false, true, false, false,
"\tcopy=True, cv=None, fit_intercept=True,\n"
+ "\tmax_iter=None, n_jobs=1, normalize=True, verbose=False"),
PassiveAggressiveClassifier("linear_model", true, false, false, false,
"\tC=1.0, fit_intercept=True, loss='hinge', n_iter=5,\n"
+ "\tn_jobs=1, random_state=None, shuffle=True, verbose=0,\n"
+ "\twarm_start=False"),
PassiveAggressiveRegressor("linear_model", false, true, false, false,
"\tC=1.0, class_weight=None, epsilon=0.1,\n"
+ "\tfit_intercept=True, loss='epsilon_insensitive', n_iter=5,\n"
+ "\trandom_state=None, shuffle=True, verbose=0, warm_start=False"),
Perceptron("linear_model", true, false, false, false,
"\talpha=0.0001, class_weight=None, " + "eta0=1.0, fit_intercept=True,\n"
+ "\tn_iter=5, n_jobs=1, penalty=None, random_state=0, shuffle=True,\n"
+ "\tverbose=0, warm_start=False"),
RANSACRegressor("linear_model", false, true, false, false,
"\tbase_estimator=None, is_data_valid=None, is_model_valid=None,\n"
+ "\tmax_trials=100, min_samples=None, random_state=None,\n"
+ "\tresidual_metric=None, residual_threshold=None, stop_n_inliers=inf,\n"
+ "\tstop_probability=0.99, stop_score=inf"),
Ridge("linear_model", false, true, false, false,
"\talpha=1.0, copy_X=True, fit_intercept=True, max_iter=None,\n"
+ "\tnormalize=False, solver='auto', tol=0.001"),
RidgeClassifier("linear_model", true, false, false, false,
"\talpha=1.0, class_weight=None, copy_X=True, fit_intercept=True,\n"
+ "\tmax_iter=None, normalize=False, solver='auto', tol=0.001"),
RidgeClassifierCV("linear_model", true, false, false, false,
"alphas=array([ 0.1, 1. , 10. ]), class_weight=None,\n"
+ "\tcv=None, fit_intercept=True, normalize=False, scoring=None"),
RidgeCV("linear_model", false, true, false, false,
"alphas=array([ 0.1, 1. , 10. ]), cv=None, fit_intercept=True,\n"
+ "\tgcv_mode=None, normalize=False, scoring=None, store_cv_values=False"),
SGDClassifier("linear_model", true, false, false, false,
"\talpha=0.0001, average=False, class_weight=None, epsilon=0.1,\n"
+ "\teta0=0.0, fit_intercept=True, l1_ratio=0.15,\n"
+ "\tlearning_rate='optimal', loss='hinge', n_iter=5, n_jobs=1,\n"
+ "\tpenalty='l2', power_t=0.5, random_state=None, shuffle=True,\n"
+ "\tverbose=0, warm_start=False") {
@Override
public boolean producesProbabilities(String params) {
return params.contains("log") || params.contains("modified_huber");
}
},
SGDRegressor("linear_model", false, true, false, false,
"\talpha=0.0001, average=False, epsilon=0.1, eta0=0.01,\n"
+ "\tfit_intercept=True, l1_ratio=0.15, learning_rate='invscaling',\n"
+ "\tloss='squared_loss', n_iter=5, penalty='l2', power_t=0.25,\n"
+ "\trandom_state=None, shuffle=True, verbose=0, warm_start=False"),
TheilSenRegressor("linear_model", false, true, false, false,
"\tcopy_X=True, fit_intercept=True, max_iter=300,\n"
+ "\tmax_subpopulation=10000, n_jobs=1, n_subsamples=None,\n"
+ "\trandom_state=None, tol=0.001, verbose=False"),
GaussianProcessRegressor("gaussian_process", false, true, false, false,
"\tkernel=None, alpha=1e-10,\n"
+ "\toptimizer='fmin_l_bfgs_b', n_restarts_optimizer=0, normalize_y=False,\n "
+ "\trandom_start=1,\n "
+ "\tnormalize=False, random_state=None"),
GaussianProcessClassifier("gaussian_process", true, false, true, false,
"\tkernel=None, optimizer='fmin_l_bfgs_b', n_restarts_optimizer=0, normalize_y=False,\n "
+ "\trandom_start=1, normalize=False, max_iter_predict=100, multi_class='one_vs_rest', random_state=None"),
KernelRidge("kernel_ridge", false, true, false, false,
"\talpha=1, coef0=1, degree=3, gamma=None, kernel='linear',\n"
+ "\tkernel_params=None"),
KNeighborsClassifier("neighbors", true, false, true, false,
"\talgorithm='auto', leaf_size=30, metric='minkowski',\n"
+ "\tmetric_params=None, n_neighbors=5, p=2, weights='uniform'"),
RadiusNeighborsClassifier("neighbors", true, false, false, false,
"\talgorithm='auto', leaf_size=30, metric='minkowski',\n"
+ "\tmetric_params=None, outlier_label=None, p=2, radius=1.0,\n"
+ "\tweights='uniform'"),
KNeighborsRegressor("neighbors", false, true, false, false,
"algorithm='auto', leaf_size=30, metric='minkowski',\n"
+ "\tmetric_params=None, n_neighbors=5, p=2, weights='uniform'"),
RadiusNeighborsRegressor("neighbors", false, true, false, false, ""),
SVC("svm", true, false, false, false,
"\tC=1.0, cache_size=200, class_weight=None, coef0=0.0, degree=3, gamma=0.0,\n"
+ "\tkernel='rbf', max_iter=-1, probability=False, random_state=None,\n"
+ "\tshrinking=True, tol=0.001, verbose=False"),
LinearSVC("svm", true, false, false, false,
"\tC=1.0, class_weight=None, dual=True, fit_intercept=True,\n"
+ "\tintercept_scaling=1, loss='squared_hinge', max_iter=1000,\n"
+ "\tmulti_class='ovr', penalty='l2', random_state=None, tol=0.0001,\n"
+ "\tverbose=0"),
NuSVC("svm", true, false, false, false,
"\tcache_size=200, coef0=0.0, degree=3, gamma=0.0, kernel='rbf',\n"
+ "\tmax_iter=-1, nu=0.5, probability=False, random_state=None,\n"
+ "\tshrinking=True, tol=0.001, verbose=False"),
SVR("svm", false, true, false, false,
"\tC=1.0, cache_size=200, coef0=0.0, degree=3, epsilon=0.1, gamma=0.0,\n"
+ "\tkernel='rbf', max_iter=-1, shrinking=True, tol=0.001, verbose=False"),
LinearSVR("svm", false, true, false, false,
"\tC=1.0, dual=True, fit_intercept=True,\n"
+ "\tintercept_scaling=1, loss='epsilon_insensitive', max_iter=1000,\n"
+ "\trandom_state=None, tol=0.0001,\n"
+ "\tverbose=0"),
NuSVR("svm", false, true, false, false,
"\tC=1.0, cache_size=200, coef0=0.0, degree=3, gamma=0.0, kernel='rbf',\n"
+ "\tmax_iter=-1, nu=0.5, shrinking=True, tol=0.001, verbose=False"),
AdaBoostClassifier("ensemble", true, false, true, false,
"\talgorithm='SAMME.R', base_estimator=None,\n"
+ "\tlearning_rate=1.0, n_estimators=50, random_state=None"),
AdaBoostRegressor("ensemble", false, true, false, false,
"\tbase_estimator=None, learning_rate=1.0, loss='linear',\n"
+ "\tn_estimators=50, random_state=None"),
BaggingClassifier("ensemble", true, false, true, false,
"\tbase_estimator=None, bootstrap=True,\n"
+ "\tbootstrap_features=False, max_features=1.0, max_samples=1.0,\n"
+ "\tn_estimators=10, n_jobs=1, oob_score=False, random_state=None,\n"
+ "\tverbose=0"),
BaggingRegressor("ensemble", false, true, false, false,
"\tbase_estimator=None, bootstrap=True,\n"
+ "\tbootstrap_features=False, max_features=1.0, max_samples=1.0,\n"
+ "\tn_estimators=10, n_jobs=1, oob_score=False, random_state=None,\n"
+ "\tverbose=0"),
ExtraTreeClassifier("tree", true, false, true, false,
"\tclass_weight=None, criterion='gini', max_depth=None,\n"
+ "\tmax_features='auto', max_leaf_nodes=None, min_samples_leaf=1,\n"
+ "\tmin_samples_split=2, min_weight_fraction_leaf=0.0,\n"
+ "\trandom_state=None, splitter='random'"),
ExtraTreeRegressor("tree", false, true, false, false,
"\tcriterion='mse', max_depth=None, max_features='auto',\n"
+ "\tmax_leaf_nodes=None, min_samples_leaf=1, min_samples_split=2,\n"
+ "\tmin_weight_fraction_leaf=0.0, random_state=None,\n"
+ "\tsplitter='random'"),
ExtraTreesClassifier("ensemble", true, false, true, false,
"\tn_estimators=100, criterion='gini', max_depth=None,\n"
+ "\tmin_samples_split=2, min_samples_leaf=1, min_weight_fraction_leaf=0.0,\n"
+ "\tmax_features='auto', max_leaf_nodes=None, min_impurity_decrease=0.0,\n"
+ "\tbootstrap=False, oob_score=False, n_jobs=None,\n"
+ "\trandom_state=None, verbose=0, warm_start=False, class_weight=None,\n"),
ExtraTreesRegressor("ensemble", false, true, false, false,
"\tn_estimators=100, criterion='mse', max_depth=None,\n"
+ "\tmin_samples_split=2, min_samples_leaf=1, min_weight_fraction_leaf=0.0,\n"
+ "\tmax_features='auto', max_leaf_nodes=None, min_impurity_decrease=0.0,\n"
+ "\tbootstrap=False, oob_score=False, n_jobs=None,\n"
+ "\trandom_state=None, verbose=0, warm_start=False, class_weight=None,\n"),
GradientBoostingClassifier("ensemble", true, false, true, false,
"\tinit=None, learning_rate=0.1, loss='deviance',\n"
+ "\tmax_depth=3, max_features=None, max_leaf_nodes=None,\n"
+ "\tmin_samples_leaf=1, min_samples_split=2,\n"
+ "\tmin_weight_fraction_leaf=0.0, n_estimators=100,\n"
+ "\trandom_state=None, subsample=1.0, verbose=0,\n"
+ "\twarm_start=False"),
GradientBoostingRegressor("ensemble", false, true, false, false,
"\talpha=0.9, init=None, learning_rate=0.1, loss='ls',\n"
+ "\tmax_depth=3, max_features=None, max_leaf_nodes=None,\n"
+ "\tmin_samples_leaf=1, min_samples_split=2,\n"
+ "\tmin_weight_fraction_leaf=0.0, n_estimators=100,\n"
+ "\trandom_state=None, subsample=1.0, verbose=0, warm_start=False"),
RandomForestClassifier("ensemble", true, false, true, false,
"\tbootstrap=True, class_weight=None, criterion='gini',\n"
+ "\tmax_depth=None, max_features='auto', max_leaf_nodes=None,\n"
+ "\tmin_samples_leaf=1, min_samples_split=2,\n"
+ "\tmin_weight_fraction_leaf=0.0, n_estimators=10, n_jobs=1,\n"
+ "\toob_score=False, random_state=None, verbose=0,\n"
+ "\twarm_start=False"),
RandomForestRegressor("ensemble", false, true, false, false,
"\tbootstrap=True, criterion='mse', max_depth=None,\n"
+ "\tmax_features='auto', max_leaf_nodes=None, min_samples_leaf=1,\n"
+ "\tmin_samples_split=2, min_weight_fraction_leaf=0.0,\n"
+ "\tn_estimators=10, n_jobs=1, oob_score=False, random_state=None,\n"
+ "\tverbose=0, warm_start=False"),
// Not actually an sklearn scheme, but does have an sklearn API
XGBClassifier("xgboost", true, false, true, true,
"\tmax_depth=3, learning_rate=0.1, n_estimators=100, silent=True,\n"
+ "\tobjective='binary:logistic', booster='gbtree', n_jobs=1,\n"
+ "\tnthread=None, gamma=0, min_child_weight=1, max_delta_step=0,\n"
+ "\tsubsample=1, colsample_bytree=1, colsample_bylevel=1,\n"
+ "\treg_alpha=0, reg_lambda=1, scale_pos_weight=1, base_score=0.5,\n"
+ "\trandom_state=0, seed=None, missing=None, tree_method='hist'"),
XGBRegressor("xgboost", false, true, false, true,
"\tmax_depth=3, learning_rate=0.1, n_estimators=100, silent=True,\n"
+ "\tobjective='reg:linear', booster='gbtree', n_jobs=1,\n"
+ "\tnthread=None, gamma=0, min_child_weight=1, max_delta_step=0,\n"
+ "\tsubsample=1, colsample_bytree=1, colsample_bylevel=1,\n"
+ "\treg_alpha=0, reg_lambda=1, scale_pos_weight=1, base_score=0.5,\n"
+ "\trandom_state=0, seed=None, missing=None, tree_method='hist'");
private String m_module;
private boolean m_classification;
private boolean m_regression;
private boolean m_producesProbabilities;
/**
* True to have the model variable cleared in python after training and
* batch prediction. This conserves memory, and might allow additional
* off-heap resources (e.g. GPU device memory) to be freed. However, there
* will be additional overhead in transferring the model back into python
* each time predictions are required.
*/
private boolean m_removeModelFromPyPostTraining;
private String m_defaultParameters;
/**
* Enum constructor
*
* @param module the scikit-learn module of the given scheme
* @param classification true if it is a classifier
* @param regression true if it is a regressor
* @param producesProbabilities true if it produces probabilities
* @param defaultParameters the list of default parameter settings
*/
Learner(String module, boolean classification, boolean regression,
boolean producesProbabilities, boolean removeModel,
String defaultParameters) {
m_module = module;
m_producesProbabilities = producesProbabilities;
m_classification = classification;
m_regression = regression;
m_removeModelFromPyPostTraining = removeModel;
m_defaultParameters = defaultParameters;
}
/**
* Get the scikit-learn module of this scheme
*
* @return the scikit-learn module of this scheme
*/
public String getModule() {
return m_module;
}
/**
* Default implementation of producesProbabilities given parameter settings.
* Specific enum values can override if there are specific parameter
* settings where probabilities can or can't be produced
*
* @param params the current parameter settings for the scheme
* @return true if probabilities can be produced given the parameter
* settings
*/
public boolean producesProbabilities(String params) {
return m_producesProbabilities;
}
/**
* Return true if the variable containing the model in python should be
* cleared after training and each batch prediction operation. This can be
* advantageous for specific methods that might either consume lots of host
* memory or device (e.g. GPU) memory. The disadvantage is that the model
* will need to be transferred into python prior to each batch prediction
* call (cross-validation will be slower).
*
* @return true if the model variable should be cleared in python
*/
public boolean removeModelFromPythonPostTrainPredict() {
return m_removeModelFromPyPostTraining;
}
/**
* Return true if this scheme is a classifier
*
* @return true if this scheme is a classifier
*/
public boolean isClassifier() {
return m_classification;
}
/**
* Return true if this scheme is a regressor
*
* @return true if this scheme is a regressor
*/
public boolean isRegressor() {
return m_regression;
}
/**
* Get the default settings for parameters for this scheme
*
* @return the default parameter settings of this scheme
*/
public String getDefaultParameters() {
return m_defaultParameters;
}
};
/** The tags for the GUI drop-down for learner selection */
public static final Tag[] TAGS_LEARNER = new Tag[Learner.values().length];
static {
for (Learner l : Learner.values()) {
TAGS_LEARNER[l.ordinal()] = new Tag(l.ordinal(), l.toString());
}
}
/**
* Holds the version number of scikit-learn. API for LDA and QDA changed in
* version 0.17.0, so we need to check for this in order to adjust code for
* these methods.
*/
protected double m_scikitVersion = -1;
/** Will be set to true if xgboost is available */
protected boolean m_xgboostInstalled;
/** The scikit learner to use */
protected Learner m_learner = Learner.DecisionTreeClassifier;
/** The parameters to pass to the learner */
protected String m_learnerOpts = "";
/** True if the supervised nominal to binary filter should be used */
protected boolean m_useSupervisedNominalToBinary;
/** The nominal to binary filter */
protected Filter m_nominalToBinary;
/** For replacing missing values */
protected Filter m_replaceMissing = new ReplaceMissingValues();
/** Holds the python serialized model */
protected String m_pickledModel;
/**
* If true, then the pickled model is not fetched from Python. Not fetching
* the model means that this classifier can't be serialized and used later.
* However, execution will be faster when running a cross-validation, and it
* is not necessary to fetch the model as it exists in the python environment
* for the duration of the virtual machine. In some cases, the size of the
* pickled model may exceed the current limits for transfer (Integer.MAX_VALUE
* bytes, about 2.1Gb).
*/
protected boolean m_dontFetchModelFromPython;
/** Holds the textual description of the scikit learner */
protected String m_learnerToString = "";
/** For making this model unique in python */
protected String m_modelHash;
/**
* True for nominal class labels that don't occur in the training data
*/
protected boolean[] m_nominalEmptyClassIndexes;
/**
* Fall back to Zero R if there are no instances with non-missing class or
* only the class is present in the data
*/
protected ZeroR m_zeroR;
/** Class priors for use if there are numerical problems */
protected double[] m_classPriors;
/** Default - use python in PATH */
protected String m_pyCommand = "default";
/** Default - use PATH as is for executing python */
protected String m_pyPath = "default";
/**
* Optional server name/ID by which to force a new server for this instance
* (or share amongst specific instances). The string "none" indicates no
* specific server name. Python command + server name uniquely identifies a
* server to use
*/
protected String m_serverID = "none";
/**
* Global help info
*
* @return the global help info for this scheme
*/
public String globalInfo() {
StringBuilder b = new StringBuilder();
b.append("A wrapper for classifiers implemented in the scikit-learn "
+ "python library. The following learners are available:\n\n");
for (Learner l : Learner.values()) {
b.append(l.toString()).append("\n");
b.append("[");
if (l.isClassifier()) {
b.append(" classification ");
}
if (l.isRegressor()) {
b.append(" regression ");
}
b.append("]").append("\nDefault parameters:\n");
b.append(l.getDefaultParameters()).append("\n");
}
return b.toString();
}
/**
* Batch prediction size (default: 100 instances)
*/
protected String m_batchPredictSize = "100";
/**
* Whether to try and continue after script execution reports output on system
* error from Python. Some schemes output warning (not error) messages to the
* sys err stream
*/
protected boolean m_continueOnSysErr;
/**
* Get the capabilities of this learner
*
* @param doServerChecks true if the python server checks should be run
* @return the Capabilities of this learner
*/
protected Capabilities getCapabilities(boolean doServerChecks) {
Capabilities result = super.getCapabilities();
result.disableAll();
if (doServerChecks) {
boolean pythonAvailable = true;
PythonSession session = null;
try {
session = getSession();
} catch (WekaException e) {
pythonAvailable = false;
}
if (pythonAvailable) {
if (m_scikitVersion < 0) {
// try and establish scikit-learn version
try {
// PythonSession session = PythonSession.acquireSession(this);
String script = "import sklearn\nskv = sklearn.__version__\n";
List outAndErr = session.executeScript(script, getDebug());
String versionNumber =
session.getVariableValueFromPythonAsPlainString("skv", getDebug());
if (versionNumber != null && versionNumber.length() > 0) {
// strip minor version
versionNumber = versionNumber.substring(0, versionNumber.lastIndexOf('.'));
m_scikitVersion = Double.parseDouble(versionNumber);
}
// check for xgboost
m_xgboostInstalled = true;
script = "import xgboost\n";
outAndErr = session.executeScript(script, getDebug());
if (outAndErr.get(1).length() > 0) {
m_xgboostInstalled = false;
}
} catch (WekaException e) {
m_xgboostInstalled = false;
e.printStackTrace();
} finally {
// PythonSession.releaseSession(this);
try {
releaseSession();
session = null;
} catch (WekaException e) {
e.printStackTrace();
}
}
}
result.enable(Capabilities.Capability.NUMERIC_ATTRIBUTES);
result.enable(Capabilities.Capability.NOMINAL_ATTRIBUTES);
result.enable(Capabilities.Capability.DATE_ATTRIBUTES);
result.enable(Capabilities.Capability.MISSING_VALUES);
result.enable(Capabilities.Capability.MISSING_CLASS_VALUES);
if (m_learner.isClassifier()) {
result.enable(Capabilities.Capability.BINARY_CLASS);
result.enable(Capabilities.Capability.NOMINAL_CLASS);
}
if (m_learner.isRegressor()) {
result.enable(Capabilities.Capability.NUMERIC_CLASS);
}
if (session != null) {
try {
releaseSession();
} catch (WekaException e) {
e.printStackTrace();
}
}
} else {
try {
String pyCheckResults = getPythonEnvCheckResults();
throw new RuntimeException(String.format("The python environment is either not available or is not configured correctly:\n\n%s", pyCheckResults));
} catch (WekaException e) {
throw new RuntimeException("The python environment is either not available or is not configured correctly", e);
}
}
} else {
result.enable(Capabilities.Capability.NUMERIC_ATTRIBUTES);
result.enable(Capabilities.Capability.NOMINAL_ATTRIBUTES);
result.enable(Capabilities.Capability.DATE_ATTRIBUTES);
result.enable(Capabilities.Capability.MISSING_VALUES);
result.enable(Capabilities.Capability.MISSING_CLASS_VALUES);
if (m_learner.isClassifier()) {
result.enable(Capabilities.Capability.BINARY_CLASS);
result.enable(Capabilities.Capability.NOMINAL_CLASS);
}
if (m_learner.isRegressor()) {
result.enable(Capabilities.Capability.NUMERIC_CLASS);
}
}
return result;
}
/**
* Get the capabilities of this learner
*
* @return the capabilities of this scheme
*/
@Override
public Capabilities getCapabilities() {
// note that we don't do the server checks from here because the GenericObjectEditor
// in the GUI calls this method for every character typed in a text field!
return getCapabilities(false);
}
/**
* Get whether to use the supervised version of nominal to binary
*
* @return true if supervised nominal to binary is to be used
*/
@OptionMetadata(displayName = "Use supervised nominal to binary conversion",
description = "Use supervised nominal to binary conversion of nominal attributes.",
commandLineParamName = "S", commandLineParamSynopsis = "-S",
commandLineParamIsFlag = true, displayOrder = 3)
public boolean getUseSupervisedNominalToBinary() {
return m_useSupervisedNominalToBinary;
}
/**
* Set whether to use the supervised version of nominal to binary
*
* @param useSupervisedNominalToBinary true if supervised nominal to binary is
* to be used
*/
public void
setUseSupervisedNominalToBinary(boolean useSupervisedNominalToBinary) {
m_useSupervisedNominalToBinary = useSupervisedNominalToBinary;
}
/**
* Get the scikit-learn scheme to use
*
* @return the scikit-learn scheme to use
*/
@OptionMetadata(displayName = "Scikit-learn learner",
description = "Scikit-learn learner to use.\nAvailable learners:\nDecisionTreeClassifier"
+ ", DecisionTreeRegressor, GaussianNB, MultinomialNB,"
+ "BernoulliNB, LDA, QDA, "
+ "LogisticRegression, LogisticRegressionCV,\n"
+ "LinearRegression, ARDRegression, "
+ "BayesianRidge, ElasticNet, Lars,\nLarsCV, Lasso, LassoCV, LassoLars, "
+ "LassoLarsCV, LassoLarsIC, MLPClassifier, MLPRegressor, OrthogonalMatchingPursuit,\n"
+ "OrthogonalMatchingPursuitCV, PassiveAggressiveClassifier, "
+ "PassiveAggressiveRegressor, Perceptron, RANSACRegressor,\nRidge, "
+ "RidgeClassifier, RidgeClassifierCV, RidgeCV, SGDClassifier,\nSGDRegressor,"
+ "TheilSenRegressor, GaussianProcess, KernelRidge, KNeighborsClassifier, "
+ "\nRadiusNeighborsClassifier, KNeighborsRegressor, RadiusNeighborsRegressor, SVC,"
+ "\nLinearSVC, NuSVC, SVR, NuSVR, AdaBoostClassifier, AdaBoostRegressor,"
+ "BaggingClassifier, BaggingRegressor,\nExtraTreeClassifier, ExtraTreeRegressor,"
+ "GradientBoostingClassifier, GradientBoostingRegressor,\n"
+ "RandomForestClassifier, RandomForestRegressor, XGBClassifier, XGBRegressor."
+ "\n(default = DecisionTreeClassifier)",
commandLineParamName = "learner",
commandLineParamSynopsis = "-learner ", displayOrder = 1)
public SelectedTag getLearner() {
return new SelectedTag(m_learner.ordinal(), TAGS_LEARNER);
}
/**
* Set the scikit-learn scheme to use
*
* @param learner the scikit-learn scheme to use
*/
public void setLearner(SelectedTag learner) {
int learnerID = learner.getSelectedTag().getID();
for (Learner l : Learner.values()) {
if (l.ordinal() == learnerID) {
m_learner = l;
break;
}
}
}
/**
* Get the parameters to pass to the scikit-learn scheme
*
* @return the parameters to use
*/
@OptionMetadata(displayName = "Learner parameters",
description = "learner parameters to use", displayOrder = 2,
commandLineParamName = "parameters",
commandLineParamSynopsis = "-parameters ")
public String getLearnerOpts() {
return m_learnerOpts;
}
/**
* Set the parameters to pass to the scikit-learn scheme
*
* @param opts the parameters to use
*/
public void setLearnerOpts(String opts) {
m_learnerOpts = opts;
}
@Override
public void setBatchSize(String size) {
m_batchPredictSize = size;
}
@OptionMetadata(displayName = "Batch size", description = "The preferred "
+ "number of instances to transfer into python for prediction\n(if operating"
+ "in batch prediction mode). More or fewer instances than this will be "
+ "accepted.", commandLineParamName = "batch",
commandLineParamSynopsis = "-batch ", displayOrder = 4)
@Override
public String getBatchSize() {
return m_batchPredictSize;
}
/**
* Returns true, as we send entire test sets over to python for prediction
*
* @return true
*/
@Override
public boolean implementsMoreEfficientBatchPrediction() {
return true;
}
/**
* Set whether to try and continue after seeing output on the sys error
* stream. Some schemes write warnings (rather than errors) to sys error.
*
* @param c true if we should try to continue after seeing output on the sys
* error stream
*/
public void setContinueOnSysErr(boolean c) {
m_continueOnSysErr = c;
}
/**
* Get whether to try and continue after seeing output on the sys error
* stream. Some schemes write warnings (rather than errors) to sys error.
*
* @return true if we should try to continue after seeing output on the sys
* error stream
*/
@OptionMetadata(
displayName = "Try to continue after sys err output from script",
description = "Try to continue after sys err output from script.\nSome schemes"
+ " report warnings to the system error stream.",
displayOrder = 5, commandLineParamName = "continue-on-err",
commandLineParamSynopsis = "-continue-on-err",
commandLineParamIsFlag = true)
public boolean getContinueOnSysErr() {
return m_continueOnSysErr;
}
/**
* If true then don't retrieve the model from python. This can speed up
* cross-validation but will prevent the trained classifier from being used
* after deserialization. May also be necessary if a model in python is very
* large and exceeds the current transfer size (Integer.MAX_VALUE bytes, about
* 2.1Gb).
*
* @param dontFetchModelFromPython true to not fetch the model from python.
*/
public void setDontFetchModelFromPython(boolean dontFetchModelFromPython) {
m_dontFetchModelFromPython = dontFetchModelFromPython;
}
/**
* If true then don't retrieve the model from python. This can speed up
* cross-validation but will prevent the trained classifier from being used
* after deserialization. May also be necessary if a model in python is very
* large and exceeds the current transfer size (Integer.MAX_VALUE bytes, about
* 2.1Gb).
*
* @return true if not fetching the model from python.
*/
@OptionMetadata(displayName = "Don't retrieve model from python",
description = "Don't retrieve the model from python - speeds up "
+ "cross-validation,\nbut prevents this classifier from being "
+ "used after deserialization.\nSome models in python (e.g. large "
+ "random forests) may exceed the maximum size for transfer\n("
+ "currently Integer.MAX_VALUE bytes)",
displayOrder = 6, commandLineParamName = "dont-fetch-model",
commandLineParamSynopsis = "-dont-fetch-model",
commandLineParamIsFlag = true)
public boolean getDontFetchModelFromPython() {
return m_dontFetchModelFromPython;
}
/**
* Set the python command to use. Empty string or "default" indicate that the
* python present in the PATH should be used.
*
* @param pyCommand the path to the python executable (or empty
* string/"default" to use python in the PATH)
*/
public void setPythonCommand(String pyCommand) {
m_pyCommand = pyCommand;
}
/**
* Get the python command to use. Empty string or "default" indicate that the
* python present in the PATH should be used.
*
* @return the path to the python executable (or empty string/"default" to use
* python in the PATH)
*/
@OptionMetadata(displayName = "Python command ",
description = "Path to python executable ('default' to use python in the PATH)",
commandLineParamName = "py-command",
commandLineParamSynopsis = "-py-command ",
displayOrder = 7)
public String getPythonCommand() {
return m_pyCommand;
}
/**
* Set optional entries to prepend to the PATH so that python can execute
* correctly. Only applies when not using a default python.
*
* @param pythonPath additional entries to prepend to the PATH
*/
public void setPythonPath(String pythonPath) {
m_pyPath = pythonPath;
}
/**
* Get optional entries to prepend to the PATH so that python can execute
* correctly. Only applies when not using a default python.
*
* @return additional entries to prepend to the PATH
*/
@OptionMetadata(displayName = "Python path",
description = "Optional elements to prepend to the PATH so that python can "
+ "execute correctly ('default' to use PATH as-is)",
commandLineParamName = "py-path",
commandLineParamSynopsis = "-py-path ", displayOrder = 8)
public String getPythonPath() {
return m_pyPath;
}
/**
* Set an optional server name by which to identify the python server to use.
* Can be used share a given server amongst selected instances or reserve a
* server specifically for this classifier. Python command + server name
* uniquely identifies the server to use.
*
* @param serverID the name of the server to use (or none for no specific
* server name).
*/
public void setServerID(String serverID) {
m_serverID = serverID;
}
/**
* Get an optional server name by which to identify the python server to use.
* Can be used share a given server amongst selected instances or reserve a
* server specifically for this classifier. Python command + server name
* uniquely identifies the server to use.
*
* @return the name of the server to use (or none) for no specific server
* name.
*/
@OptionMetadata(displayName = "Server name/ID",
description = "Optional name to identify this server, can be used to share "
+ "a given server instance - default = 'none' (i.e. no server name)",
commandLineParamName = "server-name",
commandLineParamSynopsis = "-server-name ",
displayOrder = 9)
public String getServerID() {
return m_serverID;
}
/**
* Gets a python session object to use for interacting with python
*
* @return a PythonSession object
* @throws WekaException if a problem occurs
*/
protected PythonSession getSession() throws WekaException {
PythonSession session = null;
String pyCommand = m_pyCommand != null && m_pyCommand.length() > 0
&& !m_pyCommand.equalsIgnoreCase("default") ? m_pyCommand : null;
String pyPath = m_pyPath != null && m_pyPath.length() > 0
&& !m_pyPath.equalsIgnoreCase("default") ? m_pyPath : null;
String serverID = m_serverID != null && m_serverID.length() > 0
&& !m_serverID.equalsIgnoreCase("none") ? m_serverID : null;
if (pyCommand != null) {
if (!PythonSession.pythonAvailable(pyCommand, serverID)) {
// try to create this environment/server
// System.err.println("Starting server: " + pyCommand + " " + serverID);
if (!PythonSession.initSession(pyCommand, serverID, pyPath, getDebug())) {
String envEvalResults =
PythonSession.getPythonEnvCheckResults(pyCommand, serverID);
throw new WekaException("Was unable to start python environment ("
+ pyCommand + ")\n\n" + envEvalResults);
}
}
session = PythonSession.acquireSession(pyCommand, serverID, this);
} else {
if (!PythonSession.pythonAvailable()) {
// try initializing
if (!PythonSession.initSession("python", getDebug())) {
String envEvalResults = PythonSession.getPythonEnvCheckResults();
throw new WekaException(
"Was unable to start python environment:\n\n" + envEvalResults);
}
}
session = PythonSession.acquireSession(this);
}
return session;
}
/**
* Release the python session
*
* @throws WekaException if a problem occurs
*/
protected void releaseSession() throws WekaException {
PythonSession session = null;
String pyCommand = m_pyCommand != null && m_pyCommand.length() > 0
&& !m_pyCommand.equalsIgnoreCase("default") ? m_pyCommand : null;
String serverID = m_serverID != null && m_serverID.length() > 0
&& !m_serverID.equalsIgnoreCase("none") ? m_serverID : null;
if (pyCommand != null) {
if (PythonSession.pythonAvailable(pyCommand, serverID)) {
PythonSession.releaseSession(pyCommand, serverID, this);
}
} else {
if (PythonSession.pythonAvailable()) {
PythonSession.releaseSession(this);
}
}
}
/**
* Get the results of executing the environment check in python
*
* @return the results of executing the environment check
* @throws WekaException if a problem occurs
*/
protected String getPythonEnvCheckResults() throws WekaException {
String result = "";
String pyCommand = m_pyCommand != null && m_pyCommand.length() > 0
&& !m_pyCommand.equalsIgnoreCase("default") ? m_pyCommand : null;
String serverID = m_serverID != null && m_serverID.length() > 0
&& !m_serverID.equalsIgnoreCase("none") ? m_serverID : null;
if (pyCommand != null) {
result = PythonSession.getPythonEnvCheckResults(pyCommand, serverID);
} else {
result = PythonSession.getPythonEnvCheckResults();
}
return result;
}
/**
* Check to see if python is available for the user-specified environment.
*
* @return
*/
protected boolean pythonAvailable() {
String pyCommand = m_pyCommand != null && m_pyCommand.length() > 0
&& !m_pyCommand.equalsIgnoreCase("default") ? m_pyCommand : null;
String serverID = m_serverID != null && m_serverID.length() > 0
&& !m_serverID.equalsIgnoreCase("none") ? m_serverID : null;
if (pyCommand != null) {
return PythonSession.pythonAvailable(pyCommand, serverID);
}
return PythonSession.pythonAvailable();
}
/**
* Build the classifier
*
* @param data set of instances serving as training data
* @throws Exception if a problem occurs
*/
@Override
public void buildClassifier(Instances data) throws Exception {
m_pickledModel = null;
getCapabilities(true).testWithFail(data);
m_zeroR = null;
PythonSession session = getSession();
if (m_learner == Learner.XGBClassifier && !m_xgboostInstalled) {
throw new Exception(
"xgboost does not seem to be available in your python "
+ "installation");
}
if (m_modelHash == null) {
m_modelHash = "" + hashCode();
}
data = new Instances(data);
data.deleteWithMissingClass();
m_zeroR = new ZeroR();
m_zeroR.buildClassifier(data);
m_classPriors = data.numInstances() > 0
? m_zeroR.distributionForInstance(data.instance(0))
: new double[data.classAttribute().numValues()];
if (data.numInstances() == 0 || data.numAttributes() == 1) {
if (data.numInstances() == 0) {
System.err
.println("No instances with non-missing class - using ZeroR model");
} else {
System.err.println("Only the class attribute is present in "
+ "the data - using ZeroR model");
}
return;
} else {
m_zeroR = null;
}
if (data.classAttribute().isNominal()) {
// check for empty classes
AttributeStats stats = data.attributeStats(data.classIndex());
m_nominalEmptyClassIndexes =
new boolean[data.classAttribute().numValues()];
for (int i = 0; i < stats.nominalWeights.length; i++) {
if (stats.nominalWeights[i] == 0) {
m_nominalEmptyClassIndexes[i] = true;
}
}
}
m_replaceMissing.setInputFormat(data);
data = Filter.useFilter(data, m_replaceMissing);
if (getUseSupervisedNominalToBinary()) {
m_nominalToBinary =
new weka.filters.supervised.attribute.NominalToBinary();
} else {
m_nominalToBinary =
new weka.filters.unsupervised.attribute.NominalToBinary();
}
m_nominalToBinary.setInputFormat(data);
data = Filter.useFilter(data, m_nominalToBinary);
try {
String learnerModule = m_learner.getModule();
String learnerMethod = m_learner.toString();
if (learnerMethod.equalsIgnoreCase("MLPClassifier")
|| learnerMethod.equalsIgnoreCase("MLPRegressor")) {
if (m_scikitVersion < 0.18) {
throw new Exception(
learnerMethod + " is not available in scikit-learn " + "version "
+ m_scikitVersion + ". Version 0.18 or higher is " + "required.");
}
}
// PythonSession session = PythonSession.acquireSession(this);
// transfer the data over to python
session.instancesToPythonAsScikitLearn(data, TRAINING_DATA_ID,
getDebug());
StringBuilder learnScript = new StringBuilder();
if (m_learner == Learner.XGBClassifier
|| m_learner == Learner.XGBRegressor) {
learnScript.append("import xgboost as xgb\n")
.append("import numpy as np").append("\n");
} else {
learnScript.append("from sklearn import *").append("\n")
.append("import numpy as np").append("\n");
}
if (m_scikitVersion > 0.18) {
if (learnerMethod.equalsIgnoreCase("LDA")) {
learnerMethod = "LinearDiscriminantAnalysis";
learnerModule = "discriminant_analysis";
} else if (learnerMethod.equalsIgnoreCase("QDA")) {
learnerMethod = "QuadraticDiscriminantAnalysis";
learnerModule = "discriminant_analysis";
}
}
if (m_learner == Learner.XGBClassifier
|| m_learner == Learner.XGBRegressor) {
learnScript.append(MODEL_ID + m_modelHash + " = xgb." + learnerMethod
+ "(" + getLearnerOpts() + ")").append("\n");
} else {
learnScript
.append(MODEL_ID + m_modelHash + " = " + learnerModule + "."
+ learnerMethod + "("
+ (getLearnerOpts().length() > 0 ? getLearnerOpts() : "") + ")")
.append("\n");
}
learnScript.append(MODEL_ID + m_modelHash + ".fit(X,np.ravel(Y))")
.append("\n");
List outAndErr =
session.executeScript(learnScript.toString(), getDebug());
if (outAndErr.size() == 2 && outAndErr.get(1).length() > 0) {
if (m_continueOnSysErr) {
System.err.println(outAndErr.get(1));
} else {
throw new Exception(outAndErr.get(1));
}
}
m_learnerToString = session.getVariableValueFromPythonAsPlainString(
MODEL_ID + m_modelHash, getDebug());
if (!getDontFetchModelFromPython()) {
// retrieve the model from python
m_pickledModel = session.getVariableValueFromPythonAsPickledObject(
MODEL_ID + m_modelHash, getDebug());
}
if (m_learner.removeModelFromPythonPostTrainPredict()) {
String cleanUp = "del " + MODEL_ID + m_modelHash + "\n";
outAndErr = session.executeScript(cleanUp, getDebug());
if (outAndErr.size() == 2 && outAndErr.get(1).length() > 0) {
if (m_continueOnSysErr) {
System.err.println(outAndErr.get(1));
} else {
throw new Exception(outAndErr.get(1));
}
}
}
// release session
} finally {
releaseSession();
}
}
private double[][] batchScoreWithZeroR(Instances insts) throws Exception {
double[][] result = new double[insts.numInstances()][];
for (int i = 0; i < insts.numInstances(); i++) {
Instance current = insts.instance(i);
result[i] = m_zeroR.distributionForInstance(current);
}
return result;
}
/**
* Return the predicted probabilities for the supplied instance
*
* @param instance the instance to be classified
* @return the predicted probabilities
* @throws Exception if a problem occurs
*/
@Override
public double[] distributionForInstance(Instance instance) throws Exception {
Instances temp = new Instances(instance.dataset(), 0);
temp.add(instance);
return distributionsForInstances(temp)[0];
}
/**
* Return the predicted probabilities for the supplied instances
*
* @param insts the instances to get predictions for
* @return the predicted probabilities for the supplied instances
* @throws Exception if a problem occurs
*/
@Override
@SuppressWarnings("unchecked")
public double[][] distributionsForInstances(Instances insts)
throws Exception {
if (m_zeroR != null) {
return batchScoreWithZeroR(insts);
}
insts = Filter.useFilter(insts, m_replaceMissing);
insts = Filter.useFilter(insts, m_nominalToBinary);
Attribute classAtt = insts.classAttribute();
// remove the class attribute
Remove r = new Remove();
r.setAttributeIndices("" + (insts.classIndex() + 1));
r.setInputFormat(insts);
insts = Filter.useFilter(insts, r);
insts.setClassIndex(-1);
double[][] results = null;
PythonSession session = null;
try {
session = getSession();
session.instancesToPythonAsScikitLearn(insts, TEST_DATA_ID, getDebug());
StringBuilder predictScript = new StringBuilder();
// check if model exists in python. If not, then transfer it over
if (!session.checkIfPythonVariableIsSet(MODEL_ID + m_modelHash,
getDebug())) {
if (m_pickledModel == null || m_pickledModel.length() == 0) {
throw new Exception("There is no model to transfer into Python!");
}
session.setPythonPickledVariableValue(MODEL_ID + m_modelHash,
m_pickledModel, getDebug());
}
String learnerModule = m_learner.getModule();
String learnerMethod = m_learner.toString();
if (m_scikitVersion > 0.18) {
if (learnerMethod.equalsIgnoreCase("LDA")) {
learnerMethod = "LinearDiscriminantAnalysis";
learnerModule = "discriminant_analysis";
} else if (learnerMethod.equalsIgnoreCase("QDA")) {
learnerMethod = "QuadraticDiscriminantAnalysis";
learnerModule = "discriminant_analysis";
}
}
if (m_learner == Learner.XGBClassifier
|| m_learner == Learner.XGBRegressor) {
predictScript.append("import xgboost as xgb\n");
} else {
predictScript
.append("from sklearn." + learnerModule + " import " + learnerMethod)
.append("\n");
}
predictScript.append("preds = " + MODEL_ID + m_modelHash + ".predict"
+ (m_learner.producesProbabilities(m_learnerOpts) ? "_proba" : "")
+ "(X)").append("\npreds = preds.tolist()\n");
List outAndErr =
session.executeScript(predictScript.toString(), getDebug());
if (outAndErr.size() == 2 && outAndErr.get(1).length() > 0) {
if (m_continueOnSysErr) {
System.err.println(outAndErr.get(1));
} else {
throw new Exception(outAndErr.get(1));
}
}
List
© 2015 - 2024 Weber Informatics LLC | Privacy Policy