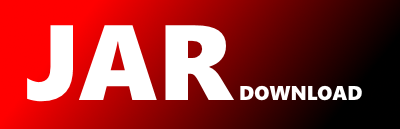
org.aika.training.LongTermLearning Maven / Gradle / Ivy
Go to download
Show more of this group Show more artifacts with this name
Show all versions of aika Show documentation
Show all versions of aika Show documentation
The Aika Text Mining Algorithm
/*
* Licensed to the Apache Software Foundation (ASF) under one or more
* contributor license agreements. See the NOTICE file distributed with
* this work for additional information regarding copyright ownership.
* The ASF licenses this file to You under the Apache License, Version 2.0
* (the "License"); you may not use this file except in compliance with
* the License. You may obtain a copy of the License at
*
* http://www.apache.org/licenses/LICENSE-2.0
*
* Unless required by applicable law or agreed to in writing, software
* distributed under the License is distributed on an "AS IS" BASIS,
* WITHOUT WARRANTIES OR CONDITIONS OF ANY KIND, either express or implied.
* See the License for the specific language governing permissions and
* limitations under the License.
*/
package org.aika.training;
import org.aika.Document;
import org.aika.Utils;
import org.aika.neuron.activation.Activation;
import org.aika.neuron.INeuron;
import org.aika.neuron.Synapse;
import org.aika.training.SynapseEvaluation.Result;
import java.util.Set;
import java.util.TreeSet;
/**
*
* Implements the biologically inspired learning algorithms for long-term potentiation and long-term depression.
*
* Wikipedia: "In neuroscience, long-term potentiation (LTP) is a persistent strengthening of synapses based on recent
* patterns of activity. These are patterns of synaptic activity that produce a long-lasting increase in signal
* transmission between two neurons. The opposite of LTP is long-term depression, which produces a long-lasting
* decrease in synaptic strength."
*
*
* @author Lukas Molzberger
*/
public class LongTermLearning {
public static class Config {
public SynapseEvaluation synapseEvaluation;
public double ltpLearnRate;
public double ltdLearnRate;
public double beta;
public boolean createNewSynapses;
/**
* Determines whether a synapse should be created between two neurons during training.
*
* @param synapseEvaluation
* @return
*/
public Config setSynapseEvaluation(SynapseEvaluation synapseEvaluation) {
this.synapseEvaluation = synapseEvaluation;
return this;
}
public Config setLTPLearnRate(double learnRate) {
this.ltpLearnRate = learnRate;
return this;
}
public Config setLTDLearnRate(double learnRate) {
this.ltdLearnRate = learnRate;
return this;
}
public Config setBeta(double beta) {
this.beta = beta;
return this;
}
public Config setCreateNewSynapses(boolean createNewSynapses) {
this.createNewSynapses = createNewSynapses;
return this;
}
}
public static void train(Document doc, Config config) {
doc.getActivations()
.filter(act -> act.targetValue == null ? act.isFinalActivation() : act.targetValue > 0.0)
.forEach(act -> {
longTermPotentiation(doc, config, act);
longTermDepression(doc, config, act, false);
longTermDepression(doc, config, act, true);
});
// doc.commit();
}
private static double hConj(Activation act) {
INeuron n = act.getINeuron();
return act.getFinalState().net / (n.biasSum + n.posDirSum + n.posRecSum);
}
/**
* The long-term potentiation algorithm is a variant of the Hebb learning rule.
*
* @param doc
* @param config
* @param act
*/
public static void longTermPotentiation(Document doc, Config config, Activation act) {
INeuron n = act.getINeuron();
double iv = Utils.nullSafeMax(act.getFinalState().value, act.targetValue);
double x = config.ltpLearnRate * (1.0 - act.getFinalState().value) * iv;
if(config.createNewSynapses) {
doc.getActivations()
.filter(iAct -> iAct.targetValue == null ? iAct.isFinalActivation() : iAct.targetValue > 0.0)
.filter(iAct -> iAct.key.node != act.key.node)
.forEach(iAct -> {
synapseLTP(config, null, iAct, act, x);
});
} else {
act.neuronInputs
.stream()
.filter(sa -> sa.input.targetValue == null ? sa.input.isFinalActivation() : sa.input.targetValue > 0.0)
.forEach(sa -> {
synapseLTP(config, sa.synapse, sa.input, act, x);
});
}
}
private static void synapseLTP(Config config, Synapse s, Activation iAct, Activation act, double x) {
Result r = config.synapseEvaluation.evaluate(s, iAct, act);
if(r == null) return;
double h = s.isConjunction(false, false) ? hConj(act) : 1.0;
double sDelta = iAct.getFinalState().value * x * r.significance * h;
if(sDelta > 0.0) {
Synapse synapse = Synapse.createOrLookup(act.doc, r.synapseKey, iAct.getNeuron(), act.getNeuron());
synapse.updateDelta(act.doc, sDelta, -config.beta * sDelta);
}
}
/**
* The long-term depression algorithm decreases the strength of a synapse if only one side of the synapse is
* firing. The algorithm tries however to preserve the logical characteristic of the synapse. If for example the
* synapse has an or-characteristic, then a non firing input neuron and a firing output neuron will not change
* the synapse weight. On the other hand, if the synapse has an and-characteristic, then a firing input neuron
* and a non firing output neuron will not change the synapse weight, too.
*
* @param doc
* @param config
* @param act
* @param dir
*/
public static void longTermDepression(Document doc, Config config, Activation act, boolean dir) {
if(act.getFinalState().value <= 0.0) return;
INeuron n = act.getINeuron();
Set actSyns = new TreeSet<>(dir ? Synapse.OUTPUT_SYNAPSE_COMP : Synapse.INPUT_SYNAPSE_COMP);
(dir ? act.neuronOutputs : act.neuronInputs)
.forEach(sa -> {
Activation rAct = dir ? sa.output : sa.input;
if(rAct.targetValue == null ? rAct.isFinalActivation() : rAct.targetValue > 0.0) {
actSyns.add(sa.synapse);
}
});
(dir ? n.outputSynapses : n.inputSynapses).values().stream()
.filter(s -> !s.isNegative() && !actSyns.contains(s))
.forEach(s -> {
if(s.isConjunction(false, false) != dir) {
Result r = config.synapseEvaluation.evaluate(s, dir ? act : null, dir ? null : act);
if (r != null) {
s.updateDelta(doc,-config.ltdLearnRate * act.getFinalState().value * r.significance, 0.0);
r.deleteMode.checkIfDelete(s, false);
}
}
});
}
}
© 2015 - 2025 Weber Informatics LLC | Privacy Policy