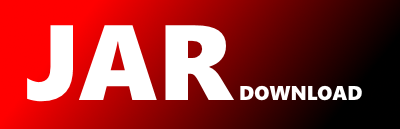
network.aika.lattice.InputNode Maven / Gradle / Ivy
/*
* Licensed to the Apache Software Foundation (ASF) under one or more
* contributor license agreements. See the NOTICE file distributed with
* this work for additional information regarding copyright ownership.
* The ASF licenses this file to You under the Apache License, Version 2.0
* (the "License"); you may not use this file except in compliance with
* the License. You may obtain a copy of the License at
*
* http://www.apache.org/licenses/LICENSE-2.0
*
* Unless required by applicable law or agreed to in writing, software
* distributed under the License is distributed on an "AS IS" BASIS,
* WITHOUT WARRANTIES OR CONDITIONS OF ANY KIND, either express or implied.
* See the License for the specific language governing permissions and
* limitations under the License.
*/
package network.aika.lattice;
import network.aika.Document;
import network.aika.Model;
import network.aika.Provider;
import network.aika.neuron.Neuron;
import network.aika.neuron.activation.Position;
import network.aika.neuron.relation.Relation;
import network.aika.neuron.activation.Activation;
import network.aika.lattice.AndNode.AndActivation;
import network.aika.lattice.InputNode.InputActivation;
import network.aika.lattice.AndNode.Refinement;
import network.aika.lattice.AndNode.RefValue;
import network.aika.lattice.AndNode.RelationsMap;
import java.io.DataInput;
import java.io.DataOutput;
import java.io.IOException;
import java.util.*;
/**
* The {@code InputNode} class is the input layer for the boolean logic. The input-node has two sources of
* activations. First, it might be underlying logic node of an {@code InputNeuron} in which case the input
* activations come from the outside. The second option is that the activation come from the output of another neuron.
*
* @author Lukas Molzberger
*/
public class InputNode extends Node {
public static int CHILD_NODE_THRESHOLD = 10;
private Neuron inputNeuron;
private TreeMap nonExactAndChildren;
public InputNode() {
}
public InputNode(Model m) {
super(m, 1);
}
public void setInputNeuron(Neuron n) {
inputNeuron = n;
}
public void addActivation(Activation inputAct) {
// if(inputAct.repropagateV != null && inputAct.repropagateV != markedCreated) return;
InputActivation act = new InputActivation(inputAct, this);
addActivation(act);
}
public void reprocessInputs(Document doc) {
inputNeuron.get(doc).getActivations(doc, false).forEach(act -> {
// act.repropagateV = markedCreated;
if(act.upperBound > 0.0) {
act.getINeuron().propagate(act);
}
});
}
void addAndChild(AndNode.Refinement ref, AndNode.RefValue child) {
super.addAndChild(ref, child);
if(!ref.relations.isExact()) {
if (nonExactAndChildren == null) {
nonExactAndChildren = new TreeMap<>();
}
AndNode.RefValue n = nonExactAndChildren.put(ref, child);
assert n == null;
}
}
void removeAndChild(AndNode.Refinement ref) {
super.removeAndChild(ref);
if(!ref.relations.isExact()) {
if (nonExactAndChildren != null) {
nonExactAndChildren.remove(ref);
if (nonExactAndChildren.isEmpty()) {
nonExactAndChildren = null;
}
}
}
}
public RefValue expand(int threadId, Document doc, Refinement ref) {
if(!ref.isConvertible()) return null;
Relation rel = ref.relations.get(0);
if(rel == null) {
return null;
}
RefValue rv = getAndChild(ref);
if(rv != null) {
return rv;
}
List nlParents = new ArrayList<>();
Refinement mirrorRef = new Refinement(new AndNode.RelationsMap(new Relation[]{rel.invert()}), provider);
nlParents.add(new AndNode.Entry(mirrorRef, new RefValue(new Integer[] {1}, 0, ref.input)));
rv = new RefValue(new Integer[] {0}, 1, provider);
nlParents.add(new AndNode.Entry(ref, rv));
return AndNode.createAndNode(provider.model, doc, nlParents, level + 1) ? rv : null;
}
/**
* @param act
*/
@Override
protected void propagate(InputActivation act) {
try {
lock.acquireReadLock();
if (andChildren != null) {
TreeMap children;
if(andChildren.size() > CHILD_NODE_THRESHOLD) {
children = nonExactAndChildren;
propagateWithExactRelations(act);
} else {
children = andChildren;
}
if(children != null) {
children.forEach((ref, rv) -> {
InputNode in = ref.input.getIfNotSuspended();
if (in != null) {
in.addNextLevelActivations(ref, rv.child.get(act.getDocument()), act);
}
});
}
}
} finally {
lock.releaseReadLock();
}
propagateToOrNode(act);
}
private void propagateWithExactRelations(InputActivation act) {
Activation iAct = act.input;
Document doc = act.getDocument();
for (Map.Entry me : iAct.slots.entrySet()) {
for (Activation linkedAct : act.getDocument().getActivationsByPosition(me.getValue(), true, me.getValue(), true)) {
Provider in = linkedAct.getINeuron().getOutputNode();
for (Map.Entry mea : andChildren.subMap(
new Refinement(RelationsMap.MIN, in),
new Refinement(RelationsMap.MAX, in)).entrySet()) {
in.get(doc).addNextLevelActivations(mea.getKey(), mea.getValue().child.get(doc), act);
}
}
}
}
private void addNextLevelActivations(Refinement ref, AndNode nln, InputActivation act) {
Document doc = act.getDocument();
if(inputNeuron.get().isEmpty(doc)) return;
Activation iAct = act.input;
if(act.repropagateV != null && act.repropagateV != nln.markedCreated) return;
ref.relations.get(0).getActivations(inputNeuron.get(doc), iAct)
.filter(secondIAct -> secondIAct.getOutputNodeActivation() != null)
.map(secondIAct -> secondIAct.getOutputNodeActivation())
.filter(secondAct -> secondAct != null && secondAct.registered)
.forEach(secondAct -> {
// if (!Conflicts.isConflicting(iAct, secondIAct)) {
AndActivation oAct = new AndActivation(doc, nln);
for (AndNode.Entry e : nln.parents) {
boolean match = e.ref.compareTo(ref) == 0;
oAct.link(e.ref, e.rv, match ? secondAct : act, match ? act : secondAct);
}
nln.addActivation(oAct);
// }
}
);
}
@Override
public void cleanup() {
}
public String logicToString() {
StringBuilder sb = new StringBuilder();
sb.append("I");
sb.append("[");
if (inputNeuron != null) {
sb.append(inputNeuron.id);
if (inputNeuron.getLabel() != null) {
sb.append(",");
sb.append(inputNeuron.getLabel());
}
}
sb.append("]");
return sb.toString();
}
@Override
public void write(DataOutput out) throws IOException {
out.writeBoolean(false);
out.writeChar('I');
super.write(out);
out.writeBoolean(inputNeuron != null);
if (inputNeuron != null) {
out.writeInt(inputNeuron.id);
}
}
@Override
public void readFields(DataInput in, Model m) throws IOException {
super.readFields(in, m);
if (in.readBoolean()) {
inputNeuron = m.lookupNeuron(in.readInt());
}
}
public static class InputActivation extends NodeActivation {
public Activation input;
public InputActivation(Activation iAct, InputNode node) {
super(iAct.getDocument(), node);
input = iAct;
iAct.setOutputNodeActivation(this);
}
public Activation getInputActivation(int i) {
assert i == 0;
return input;
}
public String toString() {
return "I-ACT(" + input.getLabel() + " " + input.slotsToString() + ")";
}
}
}
© 2015 - 2025 Weber Informatics LLC | Privacy Policy