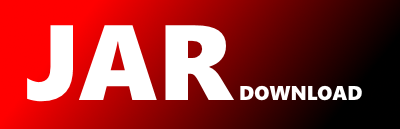
org.apache.commons.math3.random.package-info Maven / Gradle / Ivy
/*
* Licensed to the Apache Software Foundation (ASF) under one or more
* contributor license agreements. See the NOTICE file distributed with
* this work for additional information regarding copyright ownership.
* The ASF licenses this file to You under the Apache License, Version 2.0
* (the "License"); you may not use this file except in compliance with
* the License. You may obtain a copy of the License at
*
* http://www.apache.org/licenses/LICENSE-2.0
*
* Unless required by applicable law or agreed to in writing, software
* distributed under the License is distributed on an "AS IS" BASIS,
* WITHOUT WARRANTIES OR CONDITIONS OF ANY KIND, either express or implied.
* See the License for the specific language governing permissions and
* limitations under the License.
*/
/**
*
* Random number and random data generators.
* Commons-math provides a few pseudo random number generators. The top level interface is RandomGenerator.
* It is implemented by three classes:
*
* - {@link org.apache.commons.math3.random.JDKRandomGenerator JDKRandomGenerator}
* that extends the JDK provided generator
* - AbstractRandomGenerator as a helper for users generators
* - BitStreamGenerator which is an abstract class for several generators and
* which in turn is extended by:
*
* - {@link org.apache.commons.math3.random.MersenneTwister MersenneTwister}
* - {@link org.apache.commons.math3.random.Well512a Well512a}
* - {@link org.apache.commons.math3.random.Well1024a Well1024a}
* - {@link org.apache.commons.math3.random.Well19937a Well19937a}
* - {@link org.apache.commons.math3.random.Well19937c Well19937c}
* - {@link org.apache.commons.math3.random.Well44497a Well44497a}
* - {@link org.apache.commons.math3.random.Well44497b Well44497b}
*
*
*
*
*
*
* The JDK provided generator is a simple one that can be used only for very simple needs.
* The Mersenne Twister is a fast generator with very good properties well suited for
* Monte-Carlo simulation. It is equidistributed for generating vectors up to dimension 623
* and has a huge period: 219937 - 1 (which is a Mersenne prime). This generator
* is described in a paper by Makoto Matsumoto and Takuji Nishimura in 1998: Mersenne Twister:
* A 623-Dimensionally Equidistributed Uniform Pseudo-Random Number Generator, ACM
* Transactions on Modeling and Computer Simulation, Vol. 8, No. 1, January 1998, pp 3--30.
* The WELL generators are a family of generators with period ranging from 2512 - 1
* to 244497 - 1 (this last one is also a Mersenne prime) with even better properties
* than Mersenne Twister. These generators are described in a paper by François Panneton,
* Pierre L'Ecuyer and Makoto Matsumoto Improved Long-Period
* Generators Based on Linear Recurrences Modulo 2 ACM Transactions on Mathematical Software,
* 32, 1 (2006). The errata for the paper are in wellrng-errata.txt.
*
*
*
* For simple sampling, any of these generators is sufficient. For Monte-Carlo simulations the
* JDK generator does not have any of the good mathematical properties of the other generators,
* so it should be avoided. The Mersenne twister and WELL generators have equidistribution properties
* proven according to their bits pool size which is directly linked to their period (all of them
* have maximal period, i.e. a generator with size n pool has a period 2n-1). They also
* have equidistribution properties for 32 bits blocks up to s/32 dimension where s is their pool size.
* So WELL19937c for exemple is equidistributed up to dimension 623 (19937/32). This means a Monte-Carlo
* simulation generating a vector of n variables at each iteration has some guarantees on the properties
* of the vector as long as its dimension does not exceed the limit. However, since we use bits from two
* successive 32 bits generated integers to create one double, this limit is smaller when the variables are
* of type double. so for Monte-Carlo simulation where less the 16 doubles are generated at each round,
* WELL1024 may be sufficient. If a larger number of doubles are needed a generator with a larger pool
* would be useful.
*
*
*
* The WELL generators are more modern then MersenneTwister (the paper describing than has been published
* in 2006 instead of 1998) and fix some of its (few) drawbacks. If initialization array contains many
* zero bits, MersenneTwister may take a very long time (several hundreds of thousands of iterations to
* reach a steady state with a balanced number of zero and one in its bits pool). So the WELL generators
* are better to escape zeroland as explained by the WELL generators creators. The Well19937a and
* Well44497a generator are not maximally equidistributed (i.e. there are some dimensions or bits blocks
* size for which they are not equidistributed). The Well512a, Well1024a, Well19937c and Well44497b are
* maximally equidistributed for blocks size up to 32 bits (they should behave correctly also for double
* based on more than 32 bits blocks, but equidistribution is not proven at these blocks sizes).
*
*
*
* The MersenneTwister generator uses a 624 elements integer array, so it consumes less than 2.5 kilobytes.
* The WELL generators use 6 integer arrays with a size equal to the pool size, so for example the
* WELL44497b generator uses about 33 kilobytes. This may be important if a very large number of
* generator instances were used at the same time.
*
*
*
* All generators are quite fast. As an example, here are some comparisons, obtained on a 64 bits JVM on a
* linux computer with a 2008 processor (AMD phenom Quad 9550 at 2.2 GHz). The generation rate for
* MersenneTwister was about 27 millions doubles per second (remember we generate two 32 bits integers for
* each double). Generation rates for other PRNG, relative to MersenneTwister:
*
*
*
*
* Example of performances
* Name generation rate (relative to MersenneTwister)
* {@link org.apache.commons.math3.random.MersenneTwister MersenneTwister} 1
* {@link org.apache.commons.math3.random.JDKRandomGenerator JDKRandomGenerator} between 0.96 and 1.16
* {@link org.apache.commons.math3.random.Well512a Well512a} between 0.85 and 0.88
* {@link org.apache.commons.math3.random.Well1024a Well1024a} between 0.63 and 0.73
* {@link org.apache.commons.math3.random.Well19937a Well19937a} between 0.70 and 0.71
* {@link org.apache.commons.math3.random.Well19937c Well19937c} between 0.57 and 0.71
* {@link org.apache.commons.math3.random.Well44497a Well44497a} between 0.69 and 0.71
* {@link org.apache.commons.math3.random.Well44497b Well44497b} between 0.65 and 0.71
*
*
*
*
* So for most simulation problems, the better generators like {@link
* org.apache.commons.math3.random.Well19937c Well19937c} and {@link
* org.apache.commons.math3.random.Well44497b Well44497b} are probably very good choices.
*
*
*
* Note that none of these generators are suitable for cryptography. They are devoted
* to simulation, and to generate very long series with strong properties on the series as a whole
* (equidistribution, no correlation ...). They do not attempt to create small series but with
* very strong properties of unpredictability as needed in cryptography.
*
*
*
*/
package org.apache.commons.math3.random;