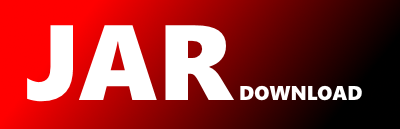
boofcv.alg.geo.trifocal.TrifocalLinearPoint7 Maven / Gradle / Ivy
Go to download
Show more of this group Show more artifacts with this name
Show all versions of geo Show documentation
Show all versions of geo Show documentation
BoofCV is an open source Java library for real-time computer vision and robotics applications.
/*
* Copyright (c) 2011-2014, Peter Abeles. All Rights Reserved.
*
* This file is part of BoofCV (http://boofcv.org).
*
* Licensed under the Apache License, Version 2.0 (the "License");
* you may not use this file except in compliance with the License.
* You may obtain a copy of the License at
*
* http://www.apache.org/licenses/LICENSE-2.0
*
* Unless required by applicable law or agreed to in writing, software
* distributed under the License is distributed on an "AS IS" BASIS,
* WITHOUT WARRANTIES OR CONDITIONS OF ANY KIND, either express or implied.
* See the License for the specific language governing permissions and
* limitations under the License.
*/
package boofcv.alg.geo.trifocal;
import boofcv.alg.geo.LowLevelMultiViewOps;
import boofcv.struct.geo.AssociatedTriple;
import boofcv.struct.geo.TrifocalTensor;
import georegression.struct.point.Point2D_F64;
import georegression.struct.point.Point3D_F64;
import org.ejml.alg.dense.decomposition.svd.SafeSvd;
import org.ejml.data.DenseMatrix64F;
import org.ejml.factory.DecompositionFactory;
import org.ejml.interfaces.decomposition.SingularValueDecomposition;
import org.ejml.ops.CommonOps;
import org.ejml.ops.SingularOps;
import java.util.List;
/**
*
* Estimates the {@link TrifocalTensor} using a linear algorithm from 7 or more image points correspondences
* from three views, see page 394 of [1] for details. After an initial linear solution has been computed
* it is improved upon by applying geometric constraints. Note that the solution will not be optimal in a geometric
* or algebraic sense, but can be used as an initial estimate for refinement algorithms.
*
*
*
* References:
*
* - R. Hartley, and A. Zisserman, "Multiple View Geometry in Computer Vision", 2nd Ed, Cambridge 2003
*
*
*
* @see EnforceTrifocalGeometry
*
* @author Peter Abeles
*/
public class TrifocalLinearPoint7 {
// trifocal tensor computed from normalized coordinates
protected TrifocalTensor solutionN = new TrifocalTensor();
// normalization matrices for points
protected DenseMatrix64F N1 = new DenseMatrix64F(3,3);
protected DenseMatrix64F N2 = new DenseMatrix64F(3,3);
protected DenseMatrix64F N3 = new DenseMatrix64F(3,3);
// the linear system which is solved for
protected DenseMatrix64F A = new DenseMatrix64F(7,27);
// svd used to extract the null space
protected SingularValueDecomposition svdNull;
// Solution in vector format
protected DenseMatrix64F vectorizedSolution = new DenseMatrix64F(27,1);
// triple of points after normalization
protected Point2D_F64 p1_norm = new Point2D_F64();
protected Point2D_F64 p2_norm = new Point2D_F64();
protected Point2D_F64 p3_norm = new Point2D_F64();
// enforces the geometry constraints
protected EnforceTrifocalGeometry enforce = new EnforceTrifocalGeometry();
protected TrifocalExtractEpipoles extractEpipoles = new TrifocalExtractEpipoles();
// Epipoles needed to enforce the above constraints
protected Point3D_F64 e2 = new Point3D_F64();
protected Point3D_F64 e3 = new Point3D_F64();
public TrifocalLinearPoint7() {
svdNull = DecompositionFactory.svd(24, 27, false, true, false);
svdNull = new SafeSvd(svdNull);
}
/**
* Estimates the trifocal tensor given the set of observations
*
* @param observations Set of observations
* @param solution Output: Where the solution is written to
* @return true if successful and false if it fails
*/
public boolean process( List observations , TrifocalTensor solution ) {
if( observations.size() < 7 )
throw new IllegalArgumentException("At least 7 correspondences must be provided");
// compute normalization to reduce numerical errors
LowLevelMultiViewOps.computeNormalization(observations, N1, N2, N3);
// compute solution in normalized pixel coordinates
createLinearSystem(observations);
// solve for the trifocal tensor
solveLinearSystem();
// enforce geometric constraints to improve solution
extractEpipoles.process(solutionN,e2,e3);
enforce.process(e2,e3,A);
enforce.extractSolution(solutionN);
// undo normalization
removeNormalization(solution);
return true;
}
/**
* Constructs the linear matrix that describes from the 3-point constraint with linear
* dependent rows removed
*/
protected void createLinearSystem( List observations ) {
int N = observations.size();
A.reshape(4*N,27);
A.zero();
int index1 = 0;
for( int i = 0; i < N; i++ ) {
AssociatedTriple t = observations.get(i);
LowLevelMultiViewOps.applyPixelNormalization(N1, t.p1, p1_norm);
LowLevelMultiViewOps.applyPixelNormalization(N2, t.p2, p2_norm);
LowLevelMultiViewOps.applyPixelNormalization(N3, t.p3, p3_norm);
int index2 = index1+9;
int index3 = index1+18;
// i = 1, j = 1
A.data[index1+8] = p1_norm.x*p2_norm.x*p3_norm.x;
A.data[index1+2] = -p1_norm.x*p3_norm.x;
A.data[index1+6] = -p1_norm.x*p2_norm.x;
A.data[index1] = p1_norm.x;
A.data[index2+8] = p1_norm.y*p2_norm.x*p3_norm.x;
A.data[index2+2] = -p1_norm.y*p3_norm.x;
A.data[index2+6] = -p1_norm.y*p2_norm.x;
A.data[index2] = p1_norm.y;
A.data[index3+8] = p2_norm.x*p3_norm.x;
A.data[index3+2] = -p3_norm.x;
A.data[index3+6] = -p2_norm.x;
A.data[index3] = 1;
// i = 1, j = 2;
index1 += 27;
index2 = index1+9;
index3 = index1+18;
A.data[index1+8] = p1_norm.x*p2_norm.x*p3_norm.y;
A.data[index1+2] = -p1_norm.x*p3_norm.y;
A.data[index1+7] = -p1_norm.x*p2_norm.x;
A.data[index1+1] = p1_norm.x;
A.data[index2+8] = p1_norm.y*p2_norm.x*p3_norm.y;
A.data[index2+2] = -p1_norm.y*p3_norm.y;
A.data[index2+7] = -p1_norm.y*p2_norm.x;
A.data[index2+1] = p1_norm.y;
A.data[index3+8] = p2_norm.x*p3_norm.y;
A.data[index3+2] = -p3_norm.y;
A.data[index3+7] = -p2_norm.x;
A.data[index3+1] = 1;
// i = 2, j = 2;
index1 += 27;
index2 = index1+9;
index3 = index1+18;
A.data[index1+8] = p1_norm.x*p2_norm.y*p3_norm.y;
A.data[index1+5] = -p1_norm.x*p3_norm.y;
A.data[index1+7] = -p1_norm.x*p2_norm.y;
A.data[index1+4] = p1_norm.x;
A.data[index2+8] = p1_norm.y*p2_norm.y*p3_norm.y;
A.data[index2+5] = -p1_norm.y*p3_norm.y;
A.data[index2+7] = -p1_norm.y*p2_norm.y;
A.data[index2+4] = p1_norm.y;
A.data[index3+8] = p2_norm.y*p3_norm.y;
A.data[index3+5] = -p3_norm.y;
A.data[index3+7] = -p2_norm.y;
A.data[index3+4] = 1;
// i = 2, j = 1;
index1 += 27;
index2 = index1+9;
index3 = index1+18;
A.data[index1+8] = p1_norm.x*p2_norm.y*p3_norm.x;
A.data[index1+5] = -p1_norm.x*p3_norm.x;
A.data[index1+6] = -p1_norm.x*p2_norm.y;
A.data[index1+3] = p1_norm.x;
A.data[index2+8] = p1_norm.y*p2_norm.y*p3_norm.x;
A.data[index2+5] = -p1_norm.y*p3_norm.x;
A.data[index2+6] = -p1_norm.y*p2_norm.y;
A.data[index2+3] = p1_norm.y;
A.data[index3+8] = p2_norm.y*p3_norm.x;
A.data[index3+5] = -p3_norm.x;
A.data[index3+6] = -p2_norm.y;
A.data[index3+3] = 1;
index1 += 27;
}
}
/**
* Computes the null space of the linear system to find the trifocal tensor
*/
protected boolean solveLinearSystem() {
if( !svdNull.decompose(A) )
return false;
SingularOps.nullVector(svdNull,true,vectorizedSolution);
solutionN.convertFrom(vectorizedSolution);
return true;
}
/**
* Translates the trifocal tensor back into regular coordinate system
*/
protected void removeNormalization( TrifocalTensor solution ) {
DenseMatrix64F N2_inv = new DenseMatrix64F(3,3);
DenseMatrix64F N3_inv = new DenseMatrix64F(3,3);
CommonOps.invert(N2,N2_inv);
CommonOps.invert(N3,N3_inv);
for( int i = 0; i < 3; i++ ) {
DenseMatrix64F T = solution.getT(i);
for( int j = 0; j < 3; j++ ) {
for( int k = 0; k < 3; k++ ) {
double sum = 0;
for( int r = 0; r < 3; r++ ) {
double n1 = N1.get(r,i);
DenseMatrix64F TN = solutionN.getT(r);
for( int s = 0; s < 3; s++ ) {
double n2 = N2_inv.get(j,s);
for( int t = 0; t < 3; t++ ) {
sum += n1*n2*N3_inv.get(k,t)*TN.get(s,t);
}
}
}
T.set(j,k,sum);
}
}
}
}
}
© 2015 - 2025 Weber Informatics LLC | Privacy Policy