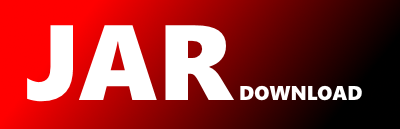
boofcv.alg.transform.pyramid.PyramidDiscreteSampleBlur Maven / Gradle / Ivy
Go to download
Show more of this group Show more artifacts with this name
Show all versions of ip Show documentation
Show all versions of ip Show documentation
BoofCV is an open source Java library for real-time computer vision and robotics applications.
/*
* Copyright (c) 2011-2013, Peter Abeles. All Rights Reserved.
*
* This file is part of BoofCV (http://boofcv.org).
*
* Licensed under the Apache License, Version 2.0 (the "License");
* you may not use this file except in compliance with the License.
* You may obtain a copy of the License at
*
* http://www.apache.org/licenses/LICENSE-2.0
*
* Unless required by applicable law or agreed to in writing, software
* distributed under the License is distributed on an "AS IS" BASIS,
* WITHOUT WARRANTIES OR CONDITIONS OF ANY KIND, either express or implied.
* See the License for the specific language governing permissions and
* limitations under the License.
*/
package boofcv.alg.transform.pyramid;
import boofcv.abst.filter.convolve.GenericConvolveDown;
import boofcv.core.image.border.BorderType;
import boofcv.factory.filter.convolve.FactoryConvolveDown;
import boofcv.struct.convolve.Kernel1D;
import boofcv.struct.image.ImageSingleBand;
import boofcv.struct.pyramid.PyramidDiscrete;
/**
*
* Convolves a re-normalizable blur kernel across the image before down sampling. This is useful for creating
* a Gaussian pyramid as well as other standard pyramids.
*
*
*
* NOTE: This pyramid cannot be configured such that blur will be applied to the input image. It can be done by
* the user before the image is passed in.
*
*
*
* NOTE: This blur magnitude is constant for each level in the pyramid. In general it is desirable to
* have it dependent on each level's scale factor.
*
*
* @author Peter Abeles
*/
@SuppressWarnings({"unchecked"})
public class PyramidDiscreteSampleBlur extends PyramidDiscrete {
// stores the results from the first convolution
private T temp;
GenericConvolveDown horizontal;
GenericConvolveDown vertical;
// amount of blur applied to each layer
double sigmas[];
/**
*
* @param kernel A blur kernel
* @param sigma The effective amount of Gaussian blur the kernel applies
* @param imageType Type of image processed
* @param saveOriginalReference If a reference to the full resolution image should be saved instead of copied.
* Set to false if you don't know what you are doing.
* @param scaleFactors Scale factor for each layer in the pyramid relative to the input layer
*/
public PyramidDiscreteSampleBlur(Kernel1D kernel, double sigma, Class imageType,
boolean saveOriginalReference, int... scaleFactors)
{
super(imageType,saveOriginalReference,scaleFactors);
horizontal = FactoryConvolveDown.convolve(kernel,imageType,imageType,
BorderType.NORMALIZED,true,1);
vertical = FactoryConvolveDown.convolve(kernel,imageType,imageType,
BorderType.NORMALIZED,false,1);
sigmas = new double[ scaleFactors.length ];
sigmas[0] = 0;
for( int i = 1; i < sigmas.length; i++ ) {
// blur in previous layer
double prev = sigmas[i-1];
// the effective amount of blur applied to previous layer while being down sampled
double applied = sigma*scaleFactors[i-1];
// The amount of blur which has been applied to this layer
sigmas[i] = Math.sqrt(prev*prev + applied*applied);
}
}
@Override
public void process(T input) {
super.initialize(input.width,input.height);
if( temp == null ) {
// declare it to be the latest image that it might need to be, resize below
temp = (T)input._createNew(1,1);
}
if (scale[0] == 1) {
if (isSaveOriginalReference()) {
setFirstLayer(input);
} else {
getLayer(0).setTo(input);
}
} else {
int skip = scale[0];
horizontal.setSkip(skip);
vertical.setSkip(skip);
temp.reshape(input.width/skip,input.height);
horizontal.process(input,temp);
vertical.process(temp,getLayer(0));
}
for (int index = 1; index < getNumLayers(); index++) {
int skip = scale[index]/scale[index-1];
T prev = getLayer(index-1);
temp.reshape(prev.width/skip,prev.height);
horizontal.setSkip(skip);
vertical.setSkip(skip);
horizontal.process(prev,temp);
vertical.process(temp,getLayer(index));
}
}
/**
* There is no offset since a symmetric kernel is applied starting at pixel (0,0)
*
* @param layer Layer in the pyramid
* @return offset
*/
@Override
public double getSampleOffset(int layer) {
return 0;
}
@Override
public double getSigma(int layer) {
return sigmas[layer];
}
}
© 2015 - 2024 Weber Informatics LLC | Privacy Policy