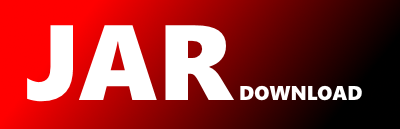
boofcv.alg.tracker.sfot.SparseFlowObjectTracker Maven / Gradle / Ivy
Go to download
Show more of this group Show more artifacts with this name
Show all versions of recognition Show documentation
Show all versions of recognition Show documentation
BoofCV is an open source Java library for real-time computer vision and robotics applications.
/*
* Copyright (c) 2011-2016, Peter Abeles. All Rights Reserved.
*
* This file is part of BoofCV (http://boofcv.org).
*
* Licensed under the Apache License, Version 2.0 (the "License");
* you may not use this file except in compliance with the License.
* You may obtain a copy of the License at
*
* http://www.apache.org/licenses/LICENSE-2.0
*
* Unless required by applicable law or agreed to in writing, software
* distributed under the License is distributed on an "AS IS" BASIS,
* WITHOUT WARRANTIES OR CONDITIONS OF ANY KIND, either express or implied.
* See the License for the specific language governing permissions and
* limitations under the License.
*/
package boofcv.alg.tracker.sfot;
import boofcv.abst.filter.derivative.ImageGradient;
import boofcv.alg.sfm.robust.DistanceScaleTranslateRotate2DSq;
import boofcv.alg.sfm.robust.GenerateScaleTranslateRotate2D;
import boofcv.alg.sfm.robust.ModelManagerScaleTranslateRotate2D;
import boofcv.alg.tracker.klt.KltTrackFault;
import boofcv.alg.tracker.klt.PyramidKltFeature;
import boofcv.alg.tracker.klt.PyramidKltTracker;
import boofcv.alg.tracker.tld.TldTracker;
import boofcv.core.image.GeneralizedImageOps;
import boofcv.factory.tracker.FactoryTrackerAlg;
import boofcv.factory.transform.pyramid.FactoryPyramid;
import boofcv.struct.RectangleRotate_F64;
import boofcv.struct.geo.AssociatedPair;
import boofcv.struct.image.ImageGray;
import boofcv.struct.pyramid.ImagePyramid;
import boofcv.struct.sfm.ScaleTranslateRotate2D;
import georegression.geometry.UtilPoint2D_F32;
import org.ddogleg.fitting.modelset.lmeds.LeastMedianOfSquares;
import org.ddogleg.struct.FastQueue;
import java.lang.reflect.Array;
/**
* Uses a pyramidal KLT tracker to track features inside the user selected region. The motion of the region
* is found robustly using {@link LeastMedianOfSquares} and a translation + rotation model. Drift is a problem
* since motion is estimated relative to the previous frame and it will eventually drift away from the original target.
* When it works well it is very smooth and can handle partially obscured objects. Can't recover after the target
* has been lost. Runs very fast.
*
* @author Peter Abeles
*/
public class SparseFlowObjectTracker
{
// for the current image
private ImagePyramid currentImage;
private Derivative[] currentDerivX;
private Derivative[] currentDerivY;
// previous image
private ImagePyramid previousImage;
private Derivative[] previousDerivX;
private Derivative[] previousDerivY;
// tracks features from frame-to-frame
private PyramidKltTracker klt;
private PyramidKltFeature track;
private FastQueue pairs = new FastQueue(AssociatedPair.class,true);
// used for estimating motion from track locations
private LeastMedianOfSquares estimateMotion;
// if true the object track has been last and can't be recovered
private boolean trackLost;
// configuration parameters
private SfotConfig config;
// class used to compute the image derivative
private ImageGradient gradient;
private Class imageType;
private Class derivType;
// maximum allowed forward-backwards error squared
private float maximumErrorFB;
// location of the target in the current frame
RectangleRotate_F64 region = new RectangleRotate_F64();
public SparseFlowObjectTracker( SfotConfig config ,
Class imageType , Class derivType ,
ImageGradient gradient ) {
this.config = config;
this.imageType = imageType;
this.derivType = derivType;
this.gradient = gradient;
maximumErrorFB = (float)(config.maximumErrorFB*config.maximumErrorFB);
klt = FactoryTrackerAlg.kltPyramid(config.trackerConfig, imageType, derivType);
ModelManagerScaleTranslateRotate2D manager = new ModelManagerScaleTranslateRotate2D();
GenerateScaleTranslateRotate2D generator = new GenerateScaleTranslateRotate2D();
DistanceScaleTranslateRotate2DSq distance = new DistanceScaleTranslateRotate2DSq();
estimateMotion = new LeastMedianOfSquares(
config.randSeed,config.robustCycles,Double.MAX_VALUE,0,manager,generator,distance);
}
public void init( Image input , RectangleRotate_F64 region ) {
if( currentImage == null ||
currentImage.getInputWidth() != input.width || currentImage.getInputHeight() != input.height) {
declarePyramid(input.width,input.height);
}
previousImage.process(input);
for( int i = 0; i < previousImage.getNumLayers(); i++ ) {
Image layer = previousImage.getLayer(i);
gradient.process(layer,previousDerivX[i],previousDerivY[i]);
}
trackLost = false;
this.region.set(region);
}
/**
* Given the input image compute the new location of the target region and store the results in output.
*
* @param input next image in the sequence.
* @param output Storage for the output.
* @return true if tracking is successful
*/
public boolean update( Image input , RectangleRotate_F64 output ) {
if( trackLost )
return false;
trackFeatures(input, region);
// See if there are enough points remaining. use of config.numberOfSamples is some what arbitrary
if( pairs.size() < config.numberOfSamples ) {
System.out.println("Lack of sample pairs");
trackLost = true;
return false;
}
// find the motion using tracked features
if( !estimateMotion.process(pairs.toList()) ) {
System.out.println("estimate motion failed");
trackLost = true;
return false;
}
if( estimateMotion.getFitQuality() > config.robustMaxError ) {
System.out.println("exceeded Max estimation error");
trackLost = true;
return false;
}
// update the target's location using the found motion
ScaleTranslateRotate2D model = estimateMotion.getModelParameters();
region.width *= model.scale;
region.height *= model.scale;
double c = Math.cos(model.theta);
double s = Math.sin(model.theta);
double x = region.cx;
double y = region.cy;
region.cx = (x*c - y*s)*model.scale + model.transX;
region.cy = (x*s + y*c)*model.scale + model.transY;
region.theta += model.theta;
output.set(region);
// make the current image into the previous image
swapImages();
return true;
}
/**
* Tracks features from the previous image into the current image. Tracks are created inside the specified
* region in a grid pattern.
*/
private void trackFeatures(Image input, RectangleRotate_F64 region) {
pairs.reset();
currentImage.process(input);
for( int i = 0; i < currentImage.getNumLayers(); i++ ) {
Image layer = currentImage.getLayer(i);
gradient.process(layer,currentDerivX[i],currentDerivY[i]);
}
// convert to float to avoid excessive conversions from double to float
float cx = (float)region.cx;
float cy = (float)region.cy;
float height = (float)(region.height);
float width = (float)(region.width);
float c = (float)Math.cos(region.theta);
float s = (float)Math.sin(region.theta);
float p = 1.0f/(config.numberOfSamples-1);
for( int i = 0; i < config.numberOfSamples; i++ ) {
float y = (p*i-0.5f)*height;
for( int j = 0; j < config.numberOfSamples; j++ ) {
float x = (p*j - 0.5f)*width;
float xx = cx + x*c - y*s;
float yy = cy + x*s + y*c;
// track in the forward direction
track.x = xx;
track.y = yy;
klt.setImage(previousImage,previousDerivX,previousDerivY);
if( !klt.setDescription(track) ) {
continue;
}
klt.setImage(currentImage,currentDerivX,currentDerivY);
KltTrackFault fault = klt.track(track);
if( fault != KltTrackFault.SUCCESS ) {
continue;
}
float xc = track.x;
float yc = track.y;
// validate by tracking backwards
if( !klt.setDescription(track) ) {
continue;
}
klt.setImage(previousImage,previousDerivX,previousDerivY);
fault = klt.track(track);
if( fault != KltTrackFault.SUCCESS ) {
continue;
}
float error = UtilPoint2D_F32.distanceSq(track.x, track.y, xx, yy);
if( error > maximumErrorFB ) {
continue;
}
// create a list of the observations
AssociatedPair a = pairs.grow();
a.p1.x = xx;
a.p1.y = yy;
a.p2.x = xc;
a.p2.y = yc;
}
}
}
/**
* Declares internal data structures
*/
private void declarePyramid( int imageWidth , int imageHeight ) {
int minSize = (config.trackerFeatureRadius*2+1)*5;
int scales[] = TldTracker.selectPyramidScale(imageWidth, imageHeight, minSize);
currentImage = FactoryPyramid.discreteGaussian(scales,-1,1,false,imageType);
currentImage.initialize(imageWidth, imageHeight);
previousImage = FactoryPyramid.discreteGaussian(scales, -1, 1, false,imageType);
previousImage.initialize(imageWidth, imageHeight);
int numPyramidLayers = currentImage.getNumLayers();
previousDerivX = (Derivative[]) Array.newInstance(derivType, numPyramidLayers);
previousDerivY = (Derivative[])Array.newInstance(derivType,numPyramidLayers);
currentDerivX = (Derivative[])Array.newInstance(derivType,numPyramidLayers);
currentDerivY = (Derivative[])Array.newInstance(derivType,numPyramidLayers);
for( int i = 0; i < numPyramidLayers; i++ ) {
int w = currentImage.getWidth(i);
int h = currentImage.getHeight(i);
previousDerivX[i] = GeneralizedImageOps.createSingleBand(derivType, w, h);
previousDerivY[i] = GeneralizedImageOps.createSingleBand(derivType, w, h);
currentDerivX[i] = GeneralizedImageOps.createSingleBand(derivType, w, h);
currentDerivY[i] = GeneralizedImageOps.createSingleBand(derivType, w, h);
}
track = new PyramidKltFeature(numPyramidLayers,config.trackerFeatureRadius);
}
/**
* Swaps the current and previous so that image derivative doesn't need to be recomputed or compied.
*/
private void swapImages() {
ImagePyramid tempP;
tempP = currentImage;
currentImage = previousImage;
previousImage = tempP;
Derivative[] tempD;
tempD = previousDerivX;
previousDerivX = currentDerivX;
currentDerivX = tempD;
tempD = previousDerivY;
previousDerivY = currentDerivY;
currentDerivY = tempD;
}
public boolean isTrackLost() {
return trackLost;
}
public SfotConfig getConfig() {
return config;
}
}
© 2015 - 2025 Weber Informatics LLC | Privacy Policy