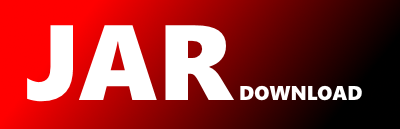
boofcv.alg.tracker.tld.TldRegionTracker Maven / Gradle / Ivy
Go to download
Show more of this group Show more artifacts with this name
Show all versions of recognition Show documentation
Show all versions of recognition Show documentation
BoofCV is an open source Java library for real-time computer vision and robotics applications.
/*
* Copyright (c) 2011-2016, Peter Abeles. All Rights Reserved.
*
* This file is part of BoofCV (http://boofcv.org).
*
* Licensed under the Apache License, Version 2.0 (the "License");
* you may not use this file except in compliance with the License.
* You may obtain a copy of the License at
*
* http://www.apache.org/licenses/LICENSE-2.0
*
* Unless required by applicable law or agreed to in writing, software
* distributed under the License is distributed on an "AS IS" BASIS,
* WITHOUT WARRANTIES OR CONDITIONS OF ANY KIND, either express or implied.
* See the License for the specific language governing permissions and
* limitations under the License.
*/
package boofcv.alg.tracker.tld;
import boofcv.abst.filter.derivative.ImageGradient;
import boofcv.alg.tracker.klt.KltTrackFault;
import boofcv.alg.tracker.klt.PyramidKltFeature;
import boofcv.alg.tracker.klt.PyramidKltTracker;
import boofcv.core.image.GeneralizedImageOps;
import boofcv.factory.transform.pyramid.FactoryPyramid;
import boofcv.struct.geo.AssociatedPair;
import boofcv.struct.image.ImageGray;
import boofcv.struct.pyramid.ImagePyramid;
import boofcv.struct.pyramid.PyramidDiscrete;
import georegression.geometry.UtilPoint2D_F32;
import georegression.struct.shapes.Rectangle2D_F64;
import org.ddogleg.sorting.QuickSelect;
import org.ddogleg.struct.FastQueue;
import java.lang.reflect.Array;
/**
* Tracks features inside target's rectangle using pyramidal KLT and updates the rectangle using found motion.
* A scale and translation model model is used. A major departure from the paper is that KLT forward-backward (FB)
* error and robust model fitting is used to prune tracks and estimate motion.
* In the paper FB and NCC error is used to prune tracks and a (in my opinion) hack is used by computing
* median error values. The way the motion is computed is more
* mathematically sound this way. NCC would provide a good sanity check, but is probably not needed.
*
* @author Peter Abeles
*/
public class TldRegionTracker< Image extends ImageGray, Derivative extends ImageGray> {
// maximum allowed median forwards-backwards error in pixels squared
private double maxErrorFB;
// for the current image
private ImagePyramid currentImage;
private Derivative[] currentDerivX;
private Derivative[] currentDerivY;
// previous image
private ImagePyramid previousImage;
private Derivative[] previousDerivX;
private Derivative[] previousDerivY;
// Derivative image type
private Class derivType;
// computes the gradient in each layer
private ImageGradient gradient;
// number of layers in the input image pyramid
private int numPyramidLayers;
// tracks features from frame-to-frame
private PyramidKltTracker tracker;
// Storage for feature tracks
private Track[] tracks;
// List showing how each active feature moved
private FastQueue pairs = new FastQueue(AssociatedPair.class,true);
// storage for computing error statistics
private double[] errorsFB;
// size of grid that features are spawned in
private int gridWidth;
// size of features being tracked
private int featureRadius;
// tracking rectangle adjusted for the image's view rectangle
private Rectangle2D_F64 spawnRect = new Rectangle2D_F64();
/**
* Configures tracker
*
* @param gridWidth Number of tracks spawned along a side in the grid. Try 10
* @param featureRadius Radius of KLT features being tracked. Try 5
* @param maxErrorFB Maximum allowed forwards-backwards error
* @param gradient Computes image gradient used by KLT tracker
* @param tracker Feature tracker
* @param imageType Type of input image
* @param derivType Type of derivative image
*/
public TldRegionTracker(int gridWidth, int featureRadius, double maxErrorFB,
ImageGradient gradient, PyramidKltTracker tracker,
Class imageType, Class derivType) {
this.gridWidth = gridWidth;
this.featureRadius = featureRadius;
this.maxErrorFB = maxErrorFB;
this.tracker = tracker;
this.gradient = gradient;
this.derivType = derivType;
tracks = new Track[ gridWidth*gridWidth ];
errorsFB = new double[ gridWidth*gridWidth ];
}
/**
* Call for the first image being tracked
*
* @param image Most recent video image.
*/
public void initialize(PyramidDiscrete image ) {
if( previousDerivX == null || previousDerivX.length != image.getNumLayers()
|| previousImage.getInputWidth() != image.getInputWidth() || previousImage.getInputHeight() != image.getInputHeight() ) {
declareDataStructures(image);
}
for( int i = 0; i < image.getNumLayers(); i++ ) {
gradient.process(image.getLayer(i), previousDerivX[i], previousDerivY[i]);
}
previousImage.setTo(image);
}
/**
* Declares internal data structures based on the input image pyramid
*/
protected void declareDataStructures(PyramidDiscrete image) {
numPyramidLayers = image.getNumLayers();
previousDerivX = (Derivative[])Array.newInstance(derivType,image.getNumLayers());
previousDerivY = (Derivative[])Array.newInstance(derivType,image.getNumLayers());
currentDerivX = (Derivative[])Array.newInstance(derivType,image.getNumLayers());
currentDerivY = (Derivative[])Array.newInstance(derivType,image.getNumLayers());
for( int i = 0; i < image.getNumLayers(); i++ ) {
int w = image.getWidth(i);
int h = image.getHeight(i);
previousDerivX[i] = GeneralizedImageOps.createSingleBand(derivType, w, h);
previousDerivY[i] = GeneralizedImageOps.createSingleBand(derivType, w, h);
currentDerivX[i] = GeneralizedImageOps.createSingleBand(derivType, w, h);
currentDerivY[i] = GeneralizedImageOps.createSingleBand(derivType, w, h);
}
previousImage = FactoryPyramid.discreteGaussian(image.getScales(), -1, 1, false,image.getImageType());
previousImage.initialize(image.getInputWidth(), image.getInputHeight());
for( int i = 0; i < tracks.length; i++ ) {
Track t = new Track();
t.klt = new PyramidKltFeature(numPyramidLayers,featureRadius);
tracks[i] = t;
}
}
/**
* Creates several tracks inside the target rectangle and compuets their motion
*
* @param image Most recent video image.
* @param targetRectangle Location of target in previous frame. Not modified.
* @return true if tracking was successful or false if not
*/
public boolean process( ImagePyramid image , Rectangle2D_F64 targetRectangle ) {
boolean success = true;
updateCurrent(image);
// create feature tracks
spawnGrid(targetRectangle);
// track features while computing forward/backward error and NCC error
if( !trackFeature() )
success = false;
// makes the current image into a previous image
setCurrentToPrevious();
return success;
}
/**
* Computes the gradient and changes the reference to the current pyramid
*/
protected void updateCurrent(ImagePyramid image) {
this.currentImage = image;
for( int i = 0; i < image.getNumLayers(); i++ ) {
gradient.process(image.getLayer(i), currentDerivX[i], currentDerivY[i]);
}
}
private void setCurrentToPrevious() {
previousImage.setTo(currentImage);
// swap gradient images
Derivative[] tmp = previousDerivX;
previousDerivX = currentDerivX;
currentDerivX = tmp;
tmp = previousDerivY;
previousDerivY = currentDerivY;
currentDerivY = tmp;
}
/**
* Tracks KLT features in forward/reverse direction and the tracking error metrics
*/
protected boolean trackFeature() {
pairs.reset();
// total number of tracks which contribute to FB error
int numTracksFB = 0;
// tracks which are not dropped
int numTracksRemaining = 0;
for( int i = 0; i < tracks.length; i++ ) {
Track t = tracks[i];
if( !t.active )
continue;
float prevX = t.klt.x;
float prevY = t.klt.y;
// track in forwards direction
tracker.setImage(currentImage,currentDerivX,currentDerivY);
KltTrackFault result = tracker.track(t.klt);
if( result != KltTrackFault.SUCCESS ) {
t.active = false;
continue;
}
float currX = t.klt.x;
float currY = t.klt.y;
// track in reverse direction
tracker.setDescription(t.klt);
tracker.setImage(previousImage, previousDerivX, previousDerivY);
result = tracker.track(t.klt);
if( result != KltTrackFault.SUCCESS ) {
t.active = false;
continue;
}
// compute forward-backwards error
double errorForwardBackwards = UtilPoint2D_F32.distanceSq(prevX,prevY,t.klt.x,t.klt.y);
// put into lists for computing the median error
errorsFB[numTracksFB++] = errorForwardBackwards;
// discard if error is too large
if( errorForwardBackwards > maxErrorFB ) {
t.active = false;
continue;
}
// create data structure used for group motion estimation
AssociatedPair p = pairs.grow();
p.p1.set( prevX, prevY );
p.p2.set( currX, currY );
numTracksRemaining++;
}
// if the forward-backwards error is too large, give up
double medianFB = QuickSelect.select(errorsFB,numTracksFB/2,numTracksFB);
// System.out.println("Median tracking error FB: "+medianFB);
if( medianFB > maxErrorFB || numTracksRemaining < 4 )
return false;
return true;
}
/**
* Spawn KLT tracks at evenly spaced points inside a grid
*/
protected void spawnGrid(Rectangle2D_F64 prevRect ) {
// Shrink the rectangle to ensure that all features are entirely contained inside
spawnRect.p0.x = prevRect.p0.x + featureRadius;
spawnRect.p0.y = prevRect.p0.y + featureRadius;
spawnRect.p1.x = prevRect.p1.x - featureRadius;
spawnRect.p1.y = prevRect.p1.y - featureRadius;
double spawnWidth = spawnRect.getWidth();
double spawnHeight = spawnRect.getHeight();
// try spawning features at evenly spaced points inside the grid
tracker.setImage(previousImage,previousDerivX,previousDerivY);
for( int i = 0; i < gridWidth; i++ ) {
float y = (float)(spawnRect.p0.y + i*spawnHeight/(gridWidth-1));
for( int j = 0; j < gridWidth; j++ ) {
float x = (float)(spawnRect.p0.x + j*spawnWidth/(gridWidth-1));
Track t = tracks[i*gridWidth+j];
t.klt.x = x;
t.klt.y = y;
if( tracker.setDescription(t.klt) ) {
t.active = true;
} else {
t.active = false;
}
}
}
}
public FastQueue getPairs() {
return pairs;
}
public Track[] getTracks() {
return tracks;
}
public static class Track {
// KLT track
PyramidKltFeature klt;
boolean active;
}
}
© 2015 - 2025 Weber Informatics LLC | Privacy Policy