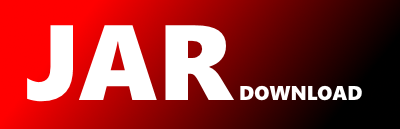
boofcv.alg.tracker.tld.TldTemplateMatching Maven / Gradle / Ivy
Go to download
Show more of this group Show more artifacts with this name
Show all versions of recognition Show documentation
Show all versions of recognition Show documentation
BoofCV is an open source Java library for real-time computer vision and robotics applications.
/*
* Copyright (c) 2011-2016, Peter Abeles. All Rights Reserved.
*
* This file is part of BoofCV (http://boofcv.org).
*
* Licensed under the Apache License, Version 2.0 (the "License");
* you may not use this file except in compliance with the License.
* You may obtain a copy of the License at
*
* http://www.apache.org/licenses/LICENSE-2.0
*
* Unless required by applicable law or agreed to in writing, software
* distributed under the License is distributed on an "AS IS" BASIS,
* WITHOUT WARRANTIES OR CONDITIONS OF ANY KIND, either express or implied.
* See the License for the specific language governing permissions and
* limitations under the License.
*/
package boofcv.alg.tracker.tld;
import boofcv.alg.descriptor.DescriptorDistance;
import boofcv.alg.interpolate.InterpolatePixelS;
import boofcv.struct.ImageRectangle;
import boofcv.struct.feature.NccFeature;
import boofcv.struct.image.ImageGray;
import java.util.ArrayList;
import java.util.List;
import java.util.Stack;
/**
* Created {@link NccFeature NCC} templates to describe the target region. Each template is composed of a 15x15
* area. The descriptor is computed by sampling evenly spaced points through out the rectangular region. Confidence
* values are computed based in the distance a point is from the closest positive and negative template.
*
* @author Peter Abeles
*/
public class TldTemplateMatching {
// set of features for positive and negative examples
private List templatePositive = new ArrayList();
private List templateNegative = new ArrayList();
// storage for the feature in the region that's being processed.
private NccFeature observed = new NccFeature(15*15);
// used when sampling the image
private InterpolatePixelS interpolate;
// storage for descriptors which can be recycled
protected Stack unused = new Stack();
public TldTemplateMatching( InterpolatePixelS interpolate ) {
this.interpolate = interpolate;
}
protected TldTemplateMatching() {
}
/**
* Discard previous results and puts it back into its initial state
*/
public void reset() {
unused.addAll(templateNegative);
unused.addAll(templatePositive);
templateNegative.clear();
templatePositive.clear();
}
/**
* Must call this function before any of the others which process descriptions
*
* @param gray Input image
*/
public void setImage( T gray ) {
interpolate.setImage(gray);
}
/**
* Creates a new descriptor for the specified region
*
* @param positive if it is a positive or negative example
*/
public void addDescriptor( boolean positive , ImageRectangle rect ) {
addDescriptor(positive, rect.x0, rect.y0, rect.x1, rect.y1);
}
public void addDescriptor( boolean positive , float x0 , float y0 , float x1 , float y1 ) {
NccFeature f = createDescriptor();
computeNccDescriptor(f,x0,y0,x1,y1);
addDescriptor(positive, f);
}
/**
* Adds a descriptor to the positive or negative list. If it is very similar to an existing one it is not
* added. Look at code for details
*
* @param positive true for positive list and false for negative list
* @param f The feature which is to be added
*/
private void addDescriptor(boolean positive, NccFeature f) {
// avoid adding the same descriptor twice or adding contradicting results
if( positive)
if( distance(f,templatePositive) < 0.05 ) {
return;
}
if( !positive) {
if( distance(f,templateNegative) < 0.05 ) {
return;
}
// a positive positive can have very bad affects on tracking, try to avoid learning a positive
// example as a negative one
if( distance(f,templatePositive) < 0.05 ) {
return;
}
}
if( positive )
templatePositive.add(f);
else
templateNegative.add(f);
}
/**
* Computes the NCC descriptor by sample points at evenly spaced distances inside the rectangle
*/
public void computeNccDescriptor( NccFeature f , float x0 , float y0 , float x1 , float y1 ) {
double mean = 0;
float widthStep = (x1-x0)/15.0f;
float heightStep = (y1-y0)/15.0f;
// compute the mean value
int index = 0;
for( int y = 0; y < 15; y++ ) {
float sampleY = y0 + y*heightStep;
for( int x = 0; x < 15; x++ ) {
mean += f.value[index++] = interpolate.get_fast(x0 + x * widthStep, sampleY);
}
}
mean /= 15*15;
// compute the variance and save the difference from the mean
double variance = 0;
index = 0;
for( int y = 0; y < 15; y++ ) {
for( int x = 0; x < 15; x++ ) {
double v = f.value[index++] -= mean;
variance += v*v;
}
}
variance /= 15*15;
f.mean = mean;
f.sigma = Math.sqrt(variance);
}
/**
* Creates a new descriptor or recycles an old one
*/
public NccFeature createDescriptor() {
NccFeature f;
if( unused.isEmpty() )
f = new NccFeature(15*15);
else
f = unused.pop();
return f;
}
/**
* Compute a value which indicates how confident the specified region is to be a member of the positive set.
* The confidence value is from 0 to 1. 1 indicates 100% confidence.
*
* Positive and negative templates are used to compute the confidence value. Only the point in each set
* which is closest to the specified region are used in the calculation.
*
* @return value from 0 to 1, where higher values are more confident
*/
public double computeConfidence( int x0 , int y0 , int x1 , int y1 ) {
computeNccDescriptor(observed,x0,y0,x1,y1);
// distance from each set of templates
if( templateNegative.size() > 0 && templatePositive.size() > 0 ) {
double distancePositive = distance(observed,templatePositive);
double distanceNegative = distance(observed,templateNegative);
return distanceNegative/(distanceNegative + distancePositive);
} else if( templatePositive.size() > 0 ) {
return 1.0-distance(observed,templatePositive);
} else {
return distance(observed,templateNegative);
}
}
/**
* see the other function with the same name
*/
public double computeConfidence( ImageRectangle r ) {
return computeConfidence(r.x0,r.y0,r.x1,r.y1);
}
/**
* Computes the best distance to 'observed' from the candidate list.
* @param observed Feature being matched
* @param candidates Set of candidate matches
* @return score from 0 to 1, where lower is closer
*/
public double distance( NccFeature observed , List candidates ) {
double maximum = -Double.MAX_VALUE;
// The feature which has the best fit will maximize the score
for( NccFeature f : candidates ) {
double score = DescriptorDistance.ncc(observed, f);
if( score > maximum )
maximum = score;
}
return 1-0.5*(maximum + 1);
}
public List getTemplatePositive() {
return templatePositive;
}
public List getTemplateNegative() {
return templateNegative;
}
}
© 2015 - 2025 Weber Informatics LLC | Privacy Policy