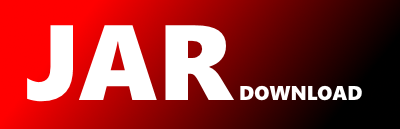
org.cicirello.search.operators.permutations.EnhancedEdgeRecombination Maven / Gradle / Ivy
Show all versions of chips-n-salsa Show documentation
/*
* Chips-n-Salsa: A library of parallel self-adaptive local search algorithms.
* Copyright (C) 2002-2022 Vincent A. Cicirello
*
* This file is part of Chips-n-Salsa (https://chips-n-salsa.cicirello.org/).
*
* Chips-n-Salsa is free software: you can redistribute it and/or modify
* it under the terms of the GNU General Public License as published by
* the Free Software Foundation, either version 3 of the License, or
* (at your option) any later version.
*
* Chips-n-Salsa is distributed in the hope that it will be useful,
* but WITHOUT ANY WARRANTY; without even the implied warranty of
* MERCHANTABILITY or FITNESS FOR A PARTICULAR PURPOSE. See the
* GNU General Public License for more details.
*
* You should have received a copy of the GNU General Public License
* along with this program. If not, see .
*/
package org.cicirello.search.operators.permutations;
import java.util.Arrays;
import org.cicirello.math.rand.RandomIndexer;
import org.cicirello.permutations.Permutation;
import org.cicirello.permutations.PermutationBinaryOperator;
import org.cicirello.search.operators.CrossoverOperator;
/**
* Implementation of the Enhanced Edge Recombination operator, a crossover operator for
* permutations. Enhanced Edge Recombination is an improvement over the original Edge Recombination
* operator. Both the original and the Enhanced Edge Recombination assumes that the permutations
* represent a cyclic sequence of edges. That is, if 3 follows 5 in the permutation, then that
* corresponds to an undirected edge between 3 and 5. Given this assumption, it is suitable for
* problems where permutations do represent a sequence of edges such as the traveling salesperson.
* However, the Chips-n-Salsa library does not limit its use to such problems, and you can use it on
* any problem with solutions represented as permutations.
*
* The original Edge Recombination operator, implemented in the {@link EdgeRecombination} class,
* is designed to create children that inherit undirected edges from the parents. A child
* permutation inherited an edge (i,j), if i and j are in adjacent positions anywhere in the child,
* and if there is at least one parent that likewise includes i and j in adjacent positions. Since
* the operator considers edges to be undirected, the order also doesn't matter. For example, if j
* immediately follows i in the child, then a parent may have been such that i immediately followed
* j. And since it considers the permutation to be a cycle, the two endpoints are considered to be
* adjacent. The documentation of the {@link EdgeRecombination} class includes an explanation of the
* inner workings of the operator, along with an example.
*
*
The Enhanced Edge Recombination operator additionally attempts to create children that inherit
* common subsequences of edges from the parents. It does so using a modified version of the edge
* map data structure introduced by Whitley et al for efficient implementation of the original Edge
* Recombination. Starkweather et al's modification involves augmenting the edge map to mark the
* edges that the parents have in common. Then, when constructing the child, during the decision of
* which element to add next to the permutation, an edge that is the start of a common subsequence
* of edges is preferred over other edges from the parents.
*
*
We leave the details to the paper that introduced the Enhanced Edge Recombination operator:
*
* T. Starkweather, S McDaniel, K Mathias, D Whitley, and C Whitley. A Comparison of Genetic
* Sequencing Operators. Proceedings of the Fourth International Conference on Genetic
* Algorithms, pages 69-76, 1991.
*
*
The worst case runtime of a call to {@link #cross cross} is O(n), where n is the length of the
* permutations.
*
* @author Vincent A. Cicirello, https://www.cicirello.org/
*/
public final class EnhancedEdgeRecombination
implements CrossoverOperator, PermutationBinaryOperator {
/** Constructs an enhanced edge recombination operator. */
public EnhancedEdgeRecombination() {}
@Override
public void cross(Permutation c1, Permutation c2) {
if (c1.length() > 1) {
c1.apply(this, c2);
}
}
@Override
public EnhancedEdgeRecombination split() {
// doesn't maintain any state, so safe to return this
return this;
}
/**
* See {@link PermutationBinaryOperator} for details of this method. This method is not intended
* for direct usage. Use the {@link #cross} method instead.
*
* @param raw1 The raw representation of the first permutation.
* @param raw2 The raw representation of the second permutation.
*/
@Override
public void apply(int[] raw1, int[] raw2) {
EnhancedEdgeMap map = new EnhancedEdgeMap(raw1, raw2);
build(raw1, new EnhancedEdgeMap(map));
build(raw2, map);
}
private void build(int[] raw, EnhancedEdgeMap map) {
// 0th element is as in parent, so start iteration at 1.
for (int i = 1; i < raw.length; i++) {
// 1. record that we used raw[i-1]
map.used(raw[i - 1]);
// 2. pick an adjacent element of raw[i-1] and add to raw[i]
raw[i] = map.pick(raw[i - 1]);
}
}
static final class EnhancedEdgeMap {
final int[][] adj;
final int[] count;
final boolean[] done;
/*
* Assumes length is greater than 1
*/
EnhancedEdgeMap(int[] raw1, int[] raw2) {
adj = new int[raw1.length][4];
count = new int[raw1.length];
done = new boolean[raw1.length];
boolean[][] in = new boolean[raw1.length][raw1.length];
adj[raw1[0]][0] = raw1[raw1.length - 1];
in[raw1[0]][raw1[raw1.length - 1]] = true;
for (int i = 1; i < raw1.length; i++) {
adj[raw1[i]][0] = raw1[i - 1];
in[raw1[i]][raw1[i - 1]] = true;
}
if (raw1.length <= 2) {
Arrays.fill(count, 1);
} else {
Arrays.fill(count, 2);
adj[raw1[raw1.length - 1]][1] = raw1[0];
in[raw1[raw1.length - 1]][raw1[0]] = true;
for (int i = 1; i < raw1.length; i++) {
adj[raw1[i - 1]][1] = raw1[i];
in[raw1[i - 1]][raw1[i]] = true;
}
if (!in[raw2[0]][raw2[raw2.length - 1]]) {
adj[raw2[0]][count[raw2[0]]] = raw2[raw2.length - 1];
in[raw2[0]][raw2[raw2.length - 1]] = true;
count[raw2[0]]++;
} else {
negate(raw2[0], raw2[raw2.length - 1]);
}
if (!in[raw2[raw2.length - 1]][raw2[0]]) {
adj[raw2[raw2.length - 1]][count[raw2[raw2.length - 1]]] = raw2[0];
in[raw2[raw2.length - 1]][raw2[0]] = true;
count[raw2[raw2.length - 1]]++;
} else {
negate(raw2[raw2.length - 1], raw2[0]);
}
for (int i = 1; i < raw2.length; i++) {
if (!in[raw2[i]][raw2[i - 1]]) {
adj[raw2[i]][count[raw2[i]]] = raw2[i - 1];
in[raw2[i]][raw2[i - 1]] = true;
count[raw2[i]]++;
} else {
negate(raw2[i], raw2[i - 1]);
}
if (!in[raw2[i - 1]][raw2[i]]) {
adj[raw2[i - 1]][count[raw2[i - 1]]] = raw2[i];
in[raw2[i - 1]][raw2[i]] = true;
count[raw2[i - 1]]++;
} else {
negate(raw2[i - 1], raw2[i]);
}
}
// Mild modification from how described by Starkweather, et al.
// Case with only 2 adjacent is when element is in the interior of
// common subsequence, and both are negative. Flip sign to positive
// to simplify logic elsewhere.
for (int i = 0; i < count.length; i++) {
if (count[i] == 2) {
// Original version was a simple negation, but they assumed elements began at 1.
// We begin at 0, and -0 obviously equals 0.
// Instead, positives directly correspond to elements, and
// our modified negation is -(v+1). This way 0 negated is -1.
adj[i][0] = -(adj[i][0] + 1);
adj[i][1] = -(adj[i][1] + 1);
}
}
}
}
EnhancedEdgeMap(EnhancedEdgeMap other) {
count = other.count.clone();
// deliberately not cloning done... this copy constructor
// only used on the initial EnhancedEdgeMap, so nothing done
done = new boolean[count.length];
adj = new int[other.adj.length][];
for (int i = 0; i < adj.length; i++) {
adj[i] = other.adj[i].clone();
}
}
final int pick(int from) {
if (count[from] == 1) {
return negateIfNecessary(adj[from][0]);
}
if (count[from] > 0) {
if (adj[from][0] < 0) {
// Original version was a simple negation, but they assumed elements began at 1.
// We begin at 0, and -0 obviously equals 0.
// Instead, positives directly correspond to elements, and
// our modified negation is -(v+1). This way 0 negated is -1.
return -(adj[from][0] + 1);
}
int[] minIndexes = new int[4];
int num = 1;
for (int i = 1; i < count[from]; i++) {
if (adj[from][i] < 0) {
// Original version was a simple negation, but they assumed elements began at 1.
// We begin at 0, and -0 obviously equals 0.
// Instead, positives directly correspond to elements, and
// our modified negation is -(v+1). This way 0 negated is -1.
return -(adj[from][i] + 1);
}
if (count[adj[from][i]] < count[adj[from][minIndexes[0]]]) {
minIndexes[0] = i;
num = 1;
} else if (count[adj[from][i]] == count[adj[from][minIndexes[0]]]) {
minIndexes[num] = i;
num++;
}
}
if (num > 1) {
// The num can be at most 3, so nextBiasedInt's lack of rejection sampling
// should introduce an extremely negligible bias away from uniformity.
return adj[from][minIndexes[RandomIndexer.nextBiasedInt(num)]];
}
return adj[from][minIndexes[0]];
}
// IS IT POSSIBLE TO GET HERE?
// IS IT POSSIBLE FOR NONE AVAILABLE?
// IF NOT, THEN ABOVE IF STATEMENT NOT NEEDED AND CAN JUST DO THE BLOCK.
// ALSO WOULDN'T NEED THE DONE ARRAY AT ALL.
// NOTE: Test cases include unit tests of this specific method that include
// an extra call after the permutation is complete to artificially create a
// scenario that ends up here. Try to confirm if a real scenario exists.
return anyRemaining();
}
final void used(int element) {
for (int i = 0; i < count[element]; i++) {
remove(negateIfNecessary(adj[element][i]), element);
}
done[element] = true;
}
final void remove(int list, int element) {
int i = 0;
// guaranteed to be in list
while (negateIfNecessary(adj[list][i]) != element) {
i++;
}
count[list]--;
adj[list][i] = adj[list][count[list]];
}
final int anyRemaining() {
int[] minIndexes = new int[adj.length];
int num = 0;
for (int i = 0; i < done.length; i++) {
if (!done[i]) {
if (num == 0) {
minIndexes[0] = i;
num = 1;
} else if (count[i] == count[minIndexes[0]]) {
minIndexes[num] = i;
num++;
} else if (count[i] < count[minIndexes[0]]) {
minIndexes[0] = i;
num = 1;
}
}
}
if (num > 1) {
// The num should be very small, so nextBiasedInt's lack of rejection sampling
// should introduce an extremely negligible bias away from uniformity. In fact, this
// case is believed extremely statistically rare.
return minIndexes[RandomIndexer.nextBiasedInt(num)];
}
if (num == 1) {
return minIndexes[0];
}
return -1;
}
private void negate(int u, int v) {
int i = 0;
// guaranteed to be in list so no bounds check needed
while (adj[u][i] != v) {
i++;
}
// Original version was a simple negation, but they assumed elements began at 1.
// We begin at 0, and -0 obviously equals 0.
// Instead, positives directly correspond to elements, and
// our modified negation is -(v+1). This way 0 negated is -1.
adj[u][i] = -(v + 1);
}
private int negateIfNecessary(int e) {
// Original version was a simple negation, but they assumed elements began at 1.
// We begin at 0, and -0 obviously equals 0.
// Instead, positives directly correspond to elements, and
// our modified negation is -(v+1). This way 0 negated is -1.
return e >= 0 ? e : -(e + 1);
}
}
}