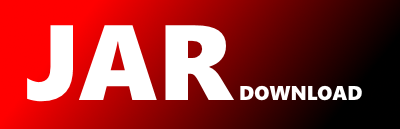
org.cicirello.search.problems.IntegerCostOptimizationProblem Maven / Gradle / Ivy
Show all versions of chips-n-salsa Show documentation
/*
* Chips-n-Salsa: A library of parallel self-adaptive local search algorithms.
* Copyright (C) 2002-2022 Vincent A. Cicirello
*
* This file is part of Chips-n-Salsa (https://chips-n-salsa.cicirello.org/).
*
* Chips-n-Salsa is free software: you can redistribute it and/or modify
* it under the terms of the GNU General Public License as published by
* the Free Software Foundation, either version 3 of the License, or
* (at your option) any later version.
*
* Chips-n-Salsa is distributed in the hope that it will be useful,
* but WITHOUT ANY WARRANTY; without even the implied warranty of
* MERCHANTABILITY or FITNESS FOR A PARTICULAR PURPOSE. See the
* GNU General Public License for more details.
*
* You should have received a copy of the GNU General Public License
* along with this program. If not, see .
*/
package org.cicirello.search.problems;
import org.cicirello.search.SolutionCostPair;
import org.cicirello.util.Copyable;
/**
* The IntegerCostOptimizationProblem interface provides search algorithms with a way to interact
* with an instance of an optimization problem without the need to know the specifics of the problem
* (e.g., traveling salesperson, bin packing, etc). It specifically concerns problems whose cost
* function is always integer valued, such as most combinatorial optimization problems.
*
* Classes that implement this interface should implement the {@link #value(Copyable) value(T)}
* method such that it returns the actual optimization objective value, and should implement the
* {@link #cost(Copyable) cost(T)} method such that lower values are better. For a minimization
* problem, these two methods can be implemented the same, while for a maximization problem, the
* {@link #cost(Copyable) cost(T)} method represents a transformation from maximization to
* minimization. This enables search algorithms to be implemented without the need to know if the
* problem is inherently minimization or maximization. That is, a search algorithm can treat every
* problem as minimization using the {@link #cost(Copyable) cost(T)} method. Upon completion,
* results can then be reported in terms of the actual optimization objective function, via the
* {@link #value(Copyable) value(T)} method.
*
*
Implementers of this interface should implement the {@link #minCost minCost} method to return
* a lower bound on the minimum cost across all possible solutions to the problem instance.
* Implementations should be fast (preferably constant time), and need not be tight. The purpose of
* this method is to enable a search algorithm to know if further search is futile (e.g., if it
* actually finds a solution whose cost is equal to the bound on the minimum theoretical cost). For
* a problem with non-negative costs, a very simple implementation might simply return 0. The
* default implementation returns Integer.MIN_VALUE.
*
* @param The type of object used to represent candidate solutions to the problem.
* @author Vincent A. Cicirello, https://www.cicirello.org/
*/
public interface IntegerCostOptimizationProblem> extends Problem {
/**
* Computes the cost of a candidate solution to the problem instance. The lower the cost, the more
* optimal the candidate solution.
*
* @param candidate The candidate solution to evaluate.
* @return The cost of the candidate solution. Lower cost means better solution.
*/
int cost(T candidate);
/**
* A lower bound on the minimum theoretical cost across all possible solutions to the problem
* instance, where lower cost implies better solution. The default implementation returns
* Integer.MIN_VALUE.
*
* @return A lower bound on the minimum theoretical cost of the problem instance.
*/
default int minCost() {
return Integer.MIN_VALUE;
}
/**
* Checks if a given cost value is equal to the minimum theoretical cost across all possible
* solutions to the problem instance, where lower cost implies better solution.
*
* @param cost The cost to check.
* @return true if cost is equal to the minimum theoretical cost,
*/
default boolean isMinCost(int cost) {
return cost == minCost();
}
/**
* Computes the value of the candidate solution within the usual constraints and interpretation of
* the problem.
*
* @param candidate The candidate solution to evaluate.
* @return The actual optimization value of the candidate solution.
*/
int value(T candidate);
/**
* {@inheritDoc}
*
* The default implementation delegates work to the {@link #cost} method, which is the desired
* behavior in most (probably all) cases. You will not likely need to override this default
* behavior.
*/
@Override
default SolutionCostPair getSolutionCostPair(T candidate) {
int c = cost(candidate);
return new SolutionCostPair(candidate, c, isMinCost(c));
}
/**
* {@inheritDoc}
*
* The default implementation delegates work to the {@link #cost} method. You should not need
* to override this default behavior.
*/
@Override
default double costAsDouble(T candidate) {
return cost(candidate);
}
}