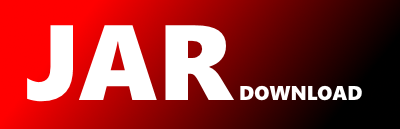
org.cicirello.search.sa.ParameterFreeLinearCooling Maven / Gradle / Ivy
Show all versions of chips-n-salsa Show documentation
/*
* Chips-n-Salsa: A library of parallel self-adaptive local search algorithms.
* Copyright (C) 2002-2021 Vincent A. Cicirello
*
* This file is part of Chips-n-Salsa (https://chips-n-salsa.cicirello.org/).
*
* Chips-n-Salsa is free software: you can redistribute it and/or modify
* it under the terms of the GNU General Public License as published by
* the Free Software Foundation, either version 3 of the License, or
* (at your option) any later version.
*
* Chips-n-Salsa is distributed in the hope that it will be useful,
* but WITHOUT ANY WARRANTY; without even the implied warranty of
* MERCHANTABILITY or FITNESS FOR A PARTICULAR PURPOSE. See the
* GNU General Public License for more details.
*
* You should have received a copy of the GNU General Public License
* along with this program. If not, see .
*/
package org.cicirello.search.sa;
import java.util.concurrent.ThreadLocalRandom;
/**
* This class implements a parameter-free version of the linear cooling schedule for simulated
* annealing. In this parameter-free version of the linear cooling schedule, the initial
* temperature, the value of Δt, and the step size are all computed by the
* ParameterFreeLinearCooling object based on an estimate of the cost difference between random
* neighbors, and the run length specified upon calling the {@link #init} method.
*
* In linear cooling, the k-th temperature, tk, is determined as follows:
* tk = t0 - k * Δt, where t0 is the initial temperature and
* Δt is the difference between two consecutive temperature values. The new temperature is
* usually computed incrementally from the previous with: tk = tk-1 -
* Δt. In some applications, the temperature update occurs with each simulated annealing
* evaluation, while in others it is updated periodically, such as every s steps (i.e., iterations)
* of simulated annealing.
*
*
The {@link #accept accept} method of this class use the classic, and most common, Boltzmann
* distribution for determining whether to accept a neighbor. With the Boltzmann distribution,
* simulated annealing accepts a neighbor with higher cost than the current state with probability
* e(c-n)/t where c is the cost of the current state, n > c is the cost of the random
* neighbor, and t is the current temperature. Note that if n ≤ c, then simulated annealing
* always accepts the neighbor.
*
*
A classic approach to setting the initial temperature t0 is to randomly sample the
* space of solutions to compute an estimate of ΔC, the average difference in cost between
* random neighbors, and to then set t0 = -ΔC / ln(P), where P < 1 is an initial
* target acceptance probability near 1. To see why, plug -ΔC / ln(P) into the Boltzmann
* distribution for t, and assume the cost c of the current state and the neighbor cost n exhibits
* the average difference, then you'd derive the following acceptance probability
* e(c-n)/t = e(c-n)/(-ΔC / ln(P)) = e-ΔC/(-ΔC /
* ln(P)) = eln(P) = P.
*
*
We use the following variation of this approach to determine an initial temperature. We
* initially accept all neighbors until we have seen 10 transitions between states with different
* cost values. We then use those 10 transitions to compute ΔC, by averaging the absolute
* value of the difference in costs across the 10 pairs of neighboring solutions, and set
* t0 = -ΔC / ln(0.95).
*
*
We then set Δt and steps (number of transitions between temperature changes) based on
* the run length specified in the maxEvals parameter of {@link #init} such that the temperature t
* declines to 0.001 by the end of the run. Specifically, we set Δt = (t0 - 0.001)
* / ceiling(k / steps), where k is the number of remaining iterations (maxEvals reduced by the
* number of iterations necessary to obtain the 10 samples used to compute t0) and where
* steps is set to the lowest power of 2 such that the Δt we compute is Δt ≥
* 10-6. The rationale for setting steps to a power of 2 is for efficiency in computing
* Δt and steps (start steps at 1 and double until Δt is in target range, very few
* iterations needed and usually terminates after first).
*
* @author Vincent A. Cicirello, https://www.cicirello.org/
*/
public final class ParameterFreeLinearCooling implements AnnealingSchedule {
private double t;
private double deltaT;
private int steps;
private int stepCounter;
private static final int ESTIMATION_SAMPLE_SIZE = 10;
private static final double LOG_INITIAL_ACCEPTANCE_PROBABILITY = Math.log(0.95);
private double costSum;
private int maxEvals;
private int numEstSamples;
/**
* Constructs a linear cooling schedule that uses first few samples to estimate cost difference
* between random neighbors, and then uses that estimate to set the initial temperature,
* temperature delta, and step size.
*/
public ParameterFreeLinearCooling() {
// deliberately empty
}
@Override
public void init(int maxEvals) {
this.maxEvals = maxEvals;
costSum = 0.0;
stepCounter = 0;
numEstSamples = 0;
t = 0;
steps = 0;
deltaT = 0;
}
@Override
public boolean accept(double neighborCost, double currentCost) {
if (numEstSamples < ESTIMATION_SAMPLE_SIZE) {
estimationStep(neighborCost, currentCost);
return true;
} else {
boolean doAccept =
neighborCost <= currentCost
|| ThreadLocalRandom.current().nextDouble()
< Math.exp((currentCost - neighborCost) / t);
stepCounter++;
if (stepCounter == steps && t > 0.001) {
stepCounter = 0;
t -= deltaT;
if (t < 0.001) t = 0.001;
}
return doAccept;
}
}
@Override
public ParameterFreeLinearCooling split() {
return new ParameterFreeLinearCooling();
}
private void estimationStep(double neighborCost, double currentCost) {
stepCounter++;
if (neighborCost != currentCost) {
numEstSamples++;
costSum = costSum - Math.abs(neighborCost - currentCost);
if (numEstSamples == ESTIMATION_SAMPLE_SIZE) {
// Set temperature using first few samples to estimate cost difference
// of random neighbors, and set temperature to cause expected acceptance
// probability of random neighbors to be near 1.0.
t = costSum / (ESTIMATION_SAMPLE_SIZE * LOG_INITIAL_ACCEPTANCE_PROBABILITY);
// sanity check, highly unlikely to occur, but make sure t not too low
if (t < 0.002) t = 0.002;
int i = 0;
int j = 0;
double drop = t - 0.001;
int remaining = maxEvals - stepCounter - 1;
// Sets deltaT and steps:
// Sets deltaT such that temperature cools to 0.001 by end of run.
// At t = 0.001, the acceptance probability should be sufficiently close
// to 0 for worsening moves that simulated annealing has converged to a hill climb.
// Sets steps relative to deltaT such that deltaT >= 1e-6.
do {
// This loop should rarely execute more than once.
int k = (remaining & j) == 0 ? remaining >> i : (remaining >> i) + 1;
deltaT = drop / k;
i++;
j = (j << 1) | 1;
} while (deltaT < 1e-6);
steps = 1 << (i - 1);
stepCounter = 0;
}
}
}
/*
* package-private for unit testing
*/
double getTemperature() {
return t;
}
/*
* package-private for unit testing
*/
double getDeltaT() {
return deltaT;
}
/*
* package-private for unit testing
*/
int getSteps() {
return steps;
}
}