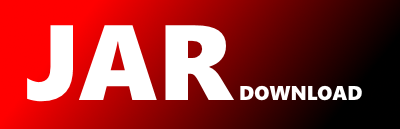
org.cicirello.search.ss.ValueBiasedStochasticSampling Maven / Gradle / Ivy
Show all versions of chips-n-salsa Show documentation
/*
* Chips-n-Salsa: A library of parallel self-adaptive local search algorithms.
* Copyright (C) 2002-2022 Vincent A. Cicirello
*
* This file is part of Chips-n-Salsa (https://chips-n-salsa.cicirello.org/).
*
* Chips-n-Salsa is free software: you can redistribute it and/or modify
* it under the terms of the GNU General Public License as published by
* the Free Software Foundation, either version 3 of the License, or
* (at your option) any later version.
*
* Chips-n-Salsa is distributed in the hope that it will be useful,
* but WITHOUT ANY WARRANTY; without even the implied warranty of
* MERCHANTABILITY or FITNESS FOR A PARTICULAR PURPOSE. See the
* GNU General Public License for more details.
*
* You should have received a copy of the GNU General Public License
* along with this program. If not, see .
*/
package org.cicirello.search.ss;
import java.util.concurrent.ThreadLocalRandom;
import org.cicirello.search.ProgressTracker;
import org.cicirello.search.SolutionCostPair;
import org.cicirello.util.Copyable;
/**
* Value Biased Stochastic Sampling (VBSS) is a form of stochastic sampling search that uses a
* constructive heuristic to bias the random decisions. In VBSS, the search generates N random
* candidate solutions to the problem, using a problem-specific heuristic to bias each random
* decision in favor of choices that are preferred by the heuristic. It evaluates each of the N
* candidate solutions with respect to the optimization problem's cost function, and returns the
* best of the N candidate solutions.
*
* Although the VBSS algorithm itself is not restricted to permutation problems, the examples
* that follow in this documentation focus on permutations for illustrative purposes.
*
*
Let's consider an illustrative example. Consider a problem whose solution is to be represented
* with a permutation of length L of the integers {0, 1, ..., (L-1)}. For the sake of this example,
* let L=4. Thus, we are searching for a permutation of the integers {0, 1, 2, 3}. We will
* iteratively build up a partial permutation containing a subset of the elements into a complete
* permutation.
*
*
We begin with an empty partial permutation: p = []. The first iteration will select the first
* element. To do so, we use a heuristic to evaluate each option within the context of the problem.
* Let S be the set of elements not yet in the permutation, which in this case is initially S = {0,
* 1, 2, 3}. Let h(p, e) be a constructive heuristic that takes as input the current partial
* permutation p, and an element e from S under consideration for addition to p, and which produces
* a real value as output that increases as the importance of adding e to p increases. That is,
* higher values of the heuristic imply that the heuristic has a higher level of confidence that the
* element e should be added next to p. For the sake of this example, consider that the heuristic
* values are as follows: h([], 0) = 5, h([], 1) = 7, h([], 2) = 1, and h([], 3) = 1. The heuristic
* seems to favor element 1 the most, and has element 0 as its second choice, and doesn't seem to
* think that elements 2 and 3 are very good choices relative to the others.
*
*
Now, VBSS also uses a bias function b. We will compute b(h(p, e)) for each element e under
* consideration for addition to partial permutation p. A common form of the bias function is b(h(p,
* e)) = h(p, e)a for some exponent a. The greater your confidence is in the decision
* making ability of the heuristic, the greater the exponent a should be. We'll use a=2 for this
* example. So given our heuristic values from above, we have the following: b(h([], 0)) = 25,
* b(h([], 1)) = 49, b(h([], 2)) = 1, and b(h([], 3)) = 1.
*
*
The element that is added to the partial permutation p is then determined randomly such that
* the probability P(e) of choosing element e is proportional to b(h(p, e)). In this example, P(0) =
* 25 / (25+49+1+1) = 25 / 76 = 0.329. P(1) = 49 / 76 = 0.645. P(2) = 1 / 76 = 0.013. And likewise
* P(3) = 0.013. So slightly less than two out of every three samples, VBSS will end up beginning
* the permutation with element 1 in this example.
*
*
For the sake of the example, let's assume that element 1 was chosen above. We now have partial
* permutation p = [1], and the set of elements not yet added to p is S = {0, 2, 3}. We need to
* compute h([1], e) for each of the elements e from S, and then compute b(h([1], e)). For most
* problems, the heuristic values would have changed. For example, if the problem was the traveling
* salesperson, then the heuristic might be in terms of the distance from the last city already in
* the partial permutation (favoring nearby cities). As you move from city to city, which cities are
* nearest will change. This is why one of the inputs to the heuristic must be the current partial
* permutation. So let's assume for the example that we recompute the heuristic and get the
* following values: h([1], 0) = 1, h([1], 2) = 4, and h([1], 3) = 3. When we compute the biases, we
* get: b(h([1], 0)) = 1, b(h([1], 2)) = 16, and b(h([1], 3)) = 9. The selection probabilities are
* thus: P(0) = 1 / (1+16+9) = 1 / 26 = 0.038; P(2) = 16 / 26 = 0.615; and P(3) = 9 / 26 = 0.346.
* Although there is much higher probability of selecting element 2, there is also a reasonably high
* chance of VBSS selecting element 3 in this case. Let's consider that it did choose element 3.
* Thus, p is now p = [1, 3] and S = {0, 2}.
*
*
One final decision is needed in this example. Let h([1, 3], 0) = 5, and h([1, 3], 2) = 6,
* which means b(h([1, 3], 0)) = 25, and b(h([1, 3], 2)) = 36. The selection probabilities are then:
* P(0) = 25 / (25+36) = 25 / 61 = 0.41, and P(2) = 36 / 61 = 0.59. Let's say that element 2 was
* chosen, so that now we have p = [0, 3, 2]. Since only one element remains, it is thus added as
* well to get p = [0, 3, 2, 1]. This permutation is then evaluated with the optimization problem's
* cost function, and the entire process repeated N times ultimately returning the best (lowest
* cost) of the N randomly sampled solutions.
*
*
To use this implementation of VBSS, you will need to implement a constructive heuristic for
* your problem using the {@link ConstructiveHeuristic} interface. The ValueBiasedStochasticSampling
* class also provides a variety of constructors enabling defining the bias function in different
* ways. The most basic uses the approach of the above example, allowing specifying the exponent,
* and the default is simply an exponent of 1. The most general allows you to specify any arbitrary
* bias function using the {@link BiasFunction} interface.
*
*
Assuming that the length of the permutation is L, and that the runtime of the constructive
* heuristic is O(f(L)), the runtime to construct one permutation using VBSS is O(L2
* f(L)). If the cost, f(L), to heuristically evaluate one permutation element is simply, O(1),
* constant time, then the cost to heuristically construct one permutation with VBSS is simply
* O(L2).
*
*
See the following two publications for the original description of the VBSS algorithm:
*
*
* - Vincent A. Cicirello. "Boosting Stochastic Problem Solvers Through Online Self-Analysis of
* Performance." PhD thesis, Ph.D. in Robotics, The Robotics Institute, School of Computer
* Science, Carnegie Mellon University, Pittsburgh, PA, July 2003.
*
- Vincent A. Cicirello and Stephen F. Smith. "Enhancing Stochastic Search Performance by Value-Biased Randomization of
* Heuristics." Journal of Heuristics, 11(1):5-34, January 2005.
*
*
* @param The type of object under optimization.
* @author Vincent A. Cicirello, https://www.cicirello.org/
*/
public final class ValueBiasedStochasticSampling>
extends AbstractStochasticSampler {
private final BiasFunction bias;
private final ConstructiveHeuristic heuristic;
/**
* Constructs a ValueBiasedStochasticSampling search object. A ProgressTracker is created for you.
* The bias function simply returns the heuristic value (random decisions are simply proportional
* to the element's heuristic value).
*
* @param heuristic The constructive heuristic.
* @throws NullPointerException if heuristic is null
*/
public ValueBiasedStochasticSampling(ConstructiveHeuristic heuristic) {
this(heuristic, null, new ProgressTracker());
}
/**
* Constructs a ValueBiasedStochasticSampling search object. The bias function simply returns the
* heuristic value (random decisions are simply proportional to the element's heuristic value).
*
* @param heuristic The constructive heuristic.
* @param tracker A ProgressTracker
* @throws NullPointerException if heuristic or tracker is null
*/
public ValueBiasedStochasticSampling(
ConstructiveHeuristic heuristic, ProgressTracker tracker) {
this(heuristic, null, tracker);
}
/**
* Constructs a ValueBiasedStochasticSampling search object. A ProgressTracker is created for you.
*
* @param heuristic The constructive heuristic.
* @param exponent The bias function is defined as: bias(value) = pow(value, exponent).
* @throws NullPointerException if heuristic is null
*/
public ValueBiasedStochasticSampling(ConstructiveHeuristic heuristic, double exponent) {
this(heuristic, exponent, new ProgressTracker());
}
/**
* Constructs a ValueBiasedStochasticSampling search object.
*
* @param heuristic The constructive heuristic.
* @param exponent The bias function is defined as: bias(value) = pow(value, exponent).
* @param tracker A ProgressTracker
* @throws NullPointerException if heuristic or tracker is null
*/
public ValueBiasedStochasticSampling(
ConstructiveHeuristic heuristic, double exponent, ProgressTracker tracker) {
this(heuristic, value -> Math.pow(value, exponent), tracker);
}
/**
* Constructs a ValueBiasedStochasticSampling search object. A ProgressTracker is created for you.
*
* @param heuristic The constructive heuristic.
* @param bias The bias function. If null, then the default bias is used.
* @throws NullPointerException if heuristic is null
*/
public ValueBiasedStochasticSampling(ConstructiveHeuristic heuristic, BiasFunction bias) {
this(heuristic, bias, new ProgressTracker());
}
/**
* Constructs a ValueBiasedStochasticSampling search object.
*
* @param heuristic The constructive heuristic.
* @param bias The bias function. If null, then the default bias is used.
* @param tracker A ProgressTracker
* @throws NullPointerException if heuristic or tracker is null
*/
public ValueBiasedStochasticSampling(
ConstructiveHeuristic heuristic, BiasFunction bias, ProgressTracker tracker) {
super(heuristic.getProblem(), tracker);
this.bias = bias;
this.heuristic = heuristic;
}
/*
* private for use by split method
*/
private ValueBiasedStochasticSampling(ValueBiasedStochasticSampling other) {
super(other);
bias = other.bias;
heuristic = other.heuristic;
}
@Override
public ValueBiasedStochasticSampling split() {
return new ValueBiasedStochasticSampling(this);
}
/**
* Creates an exponential bias function of the form: exp(scale * value). If you want to use
* exponential bias with VBSS, carefully set the scale parameter based on the scale of the cost
* function you are optimizing. There is no good general purpose default that can be provided here
* since the scale of cost function values can vary greatly from one problem to another. As you
* consider how to set the scale parameter, consider that if not set well, the bias functions can
* easily exceed the range of doubles for some cost functions.
*
* The intended usage of this method is to provide a convenient way of constructing exponential
* bias functions that can be passed to one of the constructors of the class that take a
* BiasFunction as parameter.
*
* @param scale A parameter to scale the heuristic values.
* @return A BiasFunction object representing the function: exp(scale * value)
*/
public static BiasFunction createExponentialBias(double scale) {
return value -> Math.exp(scale * value);
}
/**
* Implement this interface to implement the bias function used by VBSS. Specifically, when making
* a randomized decision among possible permutation elements to add to the permutation, VBSS
* choose randomly but biased by a function of the heuristic value. If value is the heuristic
* evaluation of permutation element e, then e will be added to the permutation with a probability
* proportional to bias(value). How you implement this depends upon how much confidence you have
* in the specific heuristic you are randomizing.
*
* @author Vincent A. Cicirello, https://www.cicirello.org/
*/
@FunctionalInterface
public static interface BiasFunction {
/**
* This method is the bias function.
*
* @param value The heuristic value of one of the elements under consideration for addition to
* the permutation.
* @return the bias function applied to that value
*/
double bias(double value);
}
/*
* package-private: used internally, but want to access from test class for unit testing
*/
void adjustForBias(double[] values, int k) {
double total = 0.0;
if (bias != null) {
for (int i = 0; i < k; i++) {
values[i] = bias.bias(values[i]);
total += values[i];
}
} else {
for (int i = 0; i < k; i++) total += values[i];
}
values[0] /= total;
for (int i = 1; i < k; i++) {
values[i] = values[i - 1] + values[i] / total;
}
values[k - 1] = 1.0;
}
@Override
SolutionCostPair sample() {
IncrementalEvaluation incEval = heuristic.createIncrementalEvaluation();
int n = heuristic.completeLength();
Partial p = heuristic.createPartial(n);
double[] b = new double[n];
ThreadLocalRandom r = ThreadLocalRandom.current();
while (!p.isComplete()) {
int k = p.numExtensions();
if (k == 1) {
if (incEval != null) {
incEval.extend(p, p.getExtension(0));
}
p.extend(0);
} else {
for (int i = 0; i < k; i++) {
b[i] = heuristic.h(p, p.getExtension(i), incEval);
}
adjustForBias(b, k);
int which = select(b, k, r.nextDouble());
if (incEval != null) {
incEval.extend(p, p.getExtension(which));
}
p.extend(which);
}
}
T complete = p.toComplete();
return evaluateAndPackageSolution(complete);
}
}