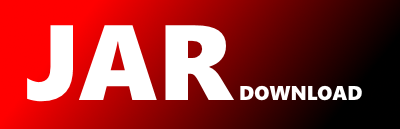
org.ddogleg.optimization.impl.DoglegStepF Maven / Gradle / Ivy
Go to download
DDogleg Numerics is a high performance Java library for non-linear optimization, robust model fitting, polynomial root finding, sorting, and more.
/*
* Copyright (c) 2012-2017, Peter Abeles. All Rights Reserved.
*
* This file is part of DDogleg (http://ddogleg.org).
*
* Licensed under the Apache License, Version 2.0 (the "License");
* you may not use this file except in compliance with the License.
* You may obtain a copy of the License at
*
* http://www.apache.org/licenses/LICENSE-2.0
*
* Unless required by applicable law or agreed to in writing, software
* distributed under the License is distributed on an "AS IS" BASIS,
* WITHOUT WARRANTIES OR CONDITIONS OF ANY KIND, either express or implied.
* See the License for the specific language governing permissions and
* limitations under the License.
*/
package org.ddogleg.optimization.impl;
import org.ejml.data.DMatrixRMaj;
import org.ejml.dense.row.CommonOps_DDRM;
import org.ejml.dense.row.NormOps_DDRM;
import org.ejml.dense.row.SpecializedOps_DDRM;
import org.ejml.dense.row.factory.LinearSolverFactory_DDRM;
import org.ejml.dense.row.mult.VectorVectorMult_DDRM;
import org.ejml.interfaces.linsol.LinearSolverDense;
/**
* @author Peter Abeles
*/
public class DoglegStepF implements TrustRegionStep {
// linear solver for least squares problem, needs to handle singular matrices
LinearSolverDense pinv;
// gradient J'*f
private DMatrixRMaj gradient;
// negative of the residuals
private DMatrixRMaj residualsNeg = new DMatrixRMaj(1,1);
// predicted reduction. Is computed efficiently depending on the case
private double predicted;
// if the step is at the region's border
private boolean maxStep;
// step and distance of Cauchy point
protected DMatrixRMaj stepCauchy = new DMatrixRMaj(1,1);
private double distanceCauchy;
private double alpha;
// step computed using Gauss-Newton
protected DMatrixRMaj stepGN = new DMatrixRMaj(1,1);
// distance of the Gauss-Newton step
private double distanceGN;
double gnorm;
// Jacobian times the gradient
DMatrixRMaj Jg = new DMatrixRMaj(1,1);
/**
* Configure internal algorithms
*
* @param pinv Linear solver for least-squares problem. Needs to handle
*/
public DoglegStepF(LinearSolverDense pinv) {
this.pinv = pinv;
}
/**
* Default solver
*/
public DoglegStepF() {
this(LinearSolverFactory_DDRM.leastSquaresQrPivot(true, false));
}
@Override
public void init(int numParam, int numFunctions) {
stepCauchy.reshape(numParam,1);
stepGN.reshape(numParam,1);
residualsNeg.reshape(numFunctions,1);
Jg.reshape(numFunctions, 1);
}
@Override
public void setInputs(DMatrixRMaj x, DMatrixRMaj residuals,
DMatrixRMaj J, DMatrixRMaj gradient, double fx) {
this.gradient = gradient;
if( !pinv.setA(J) )
throw new RuntimeException("Solver failed");
// compute Gauss Newton step
CommonOps_DDRM.scale(-1,residuals,residualsNeg);
pinv.solve(residualsNeg,stepGN);
distanceGN = NormOps_DDRM.normF(stepGN);
// Compute Cauchy step
CommonOps_DDRM.mult(J,gradient, Jg);
alpha = SpecializedOps_DDRM.elementSumSq(gradient)/SpecializedOps_DDRM.elementSumSq(Jg);
gnorm = NormOps_DDRM.normF(gradient);
distanceCauchy = alpha*gnorm;
}
@Override
public void computeStep(double regionRadius, DMatrixRMaj step) {
// of the Gauss-Newton solution is inside the trust region use that
if( distanceGN <= regionRadius ) {
step.set(stepGN);
maxStep = distanceGN == regionRadius;
predicted = -0.5* VectorVectorMult_DDRM.innerProd(stepGN, gradient);
} else if( distanceCauchy >= regionRadius ) {
// if the trust region comes before the Cauchy point then perform the cauchy step
cauchyStep(regionRadius, step);
} else {
combinedStep(regionRadius, step);
maxStep = true;
}
}
/**
* Computes the Cauchy step and the predicted reduction
*/
protected void cauchyStep(double regionRadius, DMatrixRMaj step) {
double dist;
if( distanceCauchy >= regionRadius ) {
maxStep = true;
dist = regionRadius;
} else {
maxStep = false;
dist = distanceCauchy;
}
CommonOps_DDRM.scale(-dist/gnorm, gradient, step);
predicted = regionRadius*(2.0*alpha*gnorm - regionRadius)/(2.0*alpha);
}
/**
* Computes a linear interpolation between the Cauchy and Gauss-Newton steps
*/
protected void combinedStep(double regionRadius, DMatrixRMaj step) {
// find the Cauchy point
CommonOps_DDRM.scale(-distanceCauchy/gnorm, gradient, stepCauchy);
// compute the combined step
double beta = DoglegStepFtF.combinedStep(stepCauchy,stepGN,regionRadius,step);
// predicted reduction
double predictedGN = -0.5* VectorVectorMult_DDRM.innerProd(stepGN, gradient);
predicted = 0.5*alpha*(1-beta)*(1-beta)*gnorm*gnorm + beta*(2-beta)*predictedGN;
}
@Override
public double predictedReduction() {
return predicted;
}
@Override
public boolean isMaxStep() {
return maxStep;
}
}
© 2015 - 2025 Weber Informatics LLC | Privacy Policy