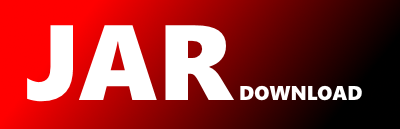
deepboof.DFunction Maven / Gradle / Ivy
Go to download
Show more of this group Show more artifacts with this name
Show all versions of main Show documentation
Show all versions of main Show documentation
Trainer Agnostic Deep Learning
/*
* Copyright (c) 2016, Peter Abeles. All Rights Reserved.
*
* This file is part of DeepBoof
*
* Licensed under the Apache License, Version 2.0 (the "License");
* you may not use this file except in compliance with the License.
* You may obtain a copy of the License at
*
* http://www.apache.org/licenses/LICENSE-2.0
*
* Unless required by applicable law or agreed to in writing, software
* distributed under the License is distributed on an "AS IS" BASIS,
* WITHOUT WARRANTIES OR CONDITIONS OF ANY KIND, either express or implied.
* See the License for the specific language governing permissions and
* limitations under the License.
*/
package deepboof;
import java.util.List;
/**
* {@link Function Functions} which also implement the backwards step and compute the gradient for all inputs.
* Functions have two modes for operation, learning and evaluating. When in learning mode they are free
* to modify their internal state during the forward step, otherwise, while in evaluation mode, they are not
* allowed to modify their state. By default, all functions start in evaluation mode.
*
* @author Peter Abeles
*/
public interface DFunction> extends Function {
/**
* Puts the function into learning mode.
*/
void learning();
/**
* Puts the function into evaluation mode.
*/
void evaluating();
/**
* Computes the derivatives of all the inputs and parameters to this function. The {@link #forward} function
* must be called first before calling this one and the same inputs and parameters must be passed in.
*
* @param input The same input tensor which was passed in during the forward pass.
* @param dout Derivative of output, computed from next layer.
* @param gradientInput gradient of input {@link Tensor}
* @param gradientParameters Gradients of all parameter {@link Tensor Tensors}. Same order as parameters
* in {@link #forward}
*/
void backwards(T input, T dout , T gradientInput , List gradientParameters );
/**
* Is the function in the learning state?
*
* @return true if in learning state or false if it's in the evaluation state
*/
boolean isLearning();
}
© 2015 - 2025 Weber Informatics LLC | Privacy Policy