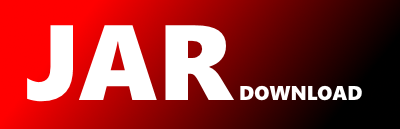
deepboof.impl.forward.standard.SpatialBatchNorm_F64 Maven / Gradle / Ivy
Go to download
Show more of this group Show more artifacts with this name
Show all versions of main Show documentation
Show all versions of main Show documentation
Trainer Agnostic Deep Learning
/*
* Copyright (c) 2016, Peter Abeles. All Rights Reserved.
*
* This file is part of DeepBoof
*
* Licensed under the Apache License, Version 2.0 (the "License");
* you may not use this file except in compliance with the License.
* You may obtain a copy of the License at
*
* http://www.apache.org/licenses/LICENSE-2.0
*
* Unless required by applicable law or agreed to in writing, software
* distributed under the License is distributed on an "AS IS" BASIS,
* WITHOUT WARRANTIES OR CONDITIONS OF ANY KIND, either express or implied.
* See the License for the specific language governing permissions and
* limitations under the License.
*/
package deepboof.impl.forward.standard;
import deepboof.forward.SpatialBatchNorm;
import deepboof.tensors.Tensor_F64;
/**
* Implementation of {@link SpatialBatchNorm} for {@link Tensor_F64}
*
* @author Peter Abeles
*/
public class SpatialBatchNorm_F64 extends FunctionBatchNorm_F64 implements SpatialBatchNorm {
public SpatialBatchNorm_F64(boolean requiresGammaBeta) {
super(requiresGammaBeta);
}
@Override
public void _initialize() {
if( shapeInput.length != 3 )
throw new IllegalArgumentException("Expected 3 DOF in a spatial shape (C,W,H)");
this.shapeOutput = shapeInput.clone();
int paramShape[] = new int[2];
paramShape[0] = shapeInput[0]; // number of channels
paramShape[1] = requiresGammaBeta ? 4 : 2; // number of variables
this.shapeParameters.add(paramShape);
this.params.reshape(paramShape);
}
@Override
public void _forward(Tensor_F64 input, Tensor_F64 output) {
int C = input.length(1);
int W = input.length(2);
int H = input.length(3);
int D = W*H;
int indexIn = input.startIndex;
int indexOut = output.startIndex;
if( hasGammaBeta() ) {
for (int batch = 0; batch < miniBatchSize; batch++) {
int indexP = params.startIndex;
for( int channel = 0; channel < C; channel++ ) {
double mean = params.d[indexP++];
double inv_stdev_eps = params.d[indexP++];
double gamma = params.d[indexP++];
double beta = params.d[indexP++];
int end = indexIn + D;
while (indexIn < end) {
output.d[indexOut++] = (input.d[indexIn++] - mean)*(gamma * inv_stdev_eps) + beta;
}
}
}
} else {
for (int batch = 0; batch < miniBatchSize; batch++) {
int indexP = params.startIndex;
for (int channel = 0; channel < C; channel++) {
double mean = params.d[indexP++];
double inv_stdev_eps = params.d[indexP++];
int end = indexIn + D;
while (indexIn < end) {
output.d[indexOut++] = (input.d[indexIn++] - mean) * inv_stdev_eps;
}
}
}
}
}
}
© 2015 - 2025 Weber Informatics LLC | Privacy Policy