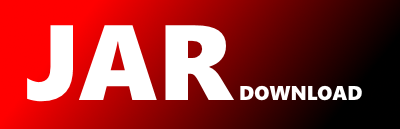
deepboof.visualization.ConfusionCounts Maven / Gradle / Ivy
Go to download
Show more of this group Show more artifacts with this name
Show all versions of visualization Show documentation
Show all versions of visualization Show documentation
Trainer Agnostic Deep Learning
/*
* Copyright (c) 2016, Peter Abeles. All Rights Reserved.
*
* This file is part of DeepBoof
*
* Licensed under the Apache License, Version 2.0 (the "License");
* you may not use this file except in compliance with the License.
* You may obtain a copy of the License at
*
* http://www.apache.org/licenses/LICENSE-2.0
*
* Unless required by applicable law or agreed to in writing, software
* distributed under the License is distributed on an "AS IS" BASIS,
* WITHOUT WARRANTIES OR CONDITIONS OF ANY KIND, either express or implied.
* See the License for the specific language governing permissions and
* limitations under the License.
*/
package deepboof.visualization;
import java.util.List;
/**
* Stores a confusion matrix of results in an N by N integer row-major matrix and provides functions for computing
* different statistical properties. To compute different statistical properties first invoke {@link #precompute()}.
*
* @author Peter Abeles
*/
public class ConfusionCounts {
/**
* N by N matrix of counts. Each element represents the number of times category 'row' was labeled
* 'col'. Thus, the diagonals are the totals that each category has been correctly labeled.
*/
public int counts[];
// precompute the sums along rows and columns
public int sumRows[];
public int sumCols[];
/**
* Optional labels for each category. Can be null.
*/
public List labels;
/**
* Number of categories
*/
public int N;
public ConfusionCounts(List labels) {
this(labels.size());
this.labels = labels;
}
public ConfusionCounts(int N) {
this.N = N;
counts = new int[this.N * this.N];
sumRows = new int[this.N];
sumCols = new int[this.N];
}
public void precompute() {
for (int row = 0; row < N; row++) {
int sum = 0;
for (int col = 0; col < N; col++) {
sum += get(row,col);
}
sumRows[row] = sum;
}
for (int col = 0; col < N; col++) {
int sum = 0;
for (int row = 0; row < N; row++) {
sum += get(row,col);
}
sumCols[col] = sum;
}
}
public void increment( int actual , int predicted ) {
counts[actual*N+predicted]++;
}
public void set( int row , int col , int num ) {
counts[row*N+col] = num;
}
/**
* Returns the total number of times category 'row' was labeled 'col'.
* @param row Index of category
* @param col Index of category
* @return counts
*/
public int get( int row , int col ) {
return counts[row*labels.size()+col];
}
/**
* Returns the fraction of time it correctly labeled this category
*/
public double precision( int which ) {
return get(which,which)/(double)sumRows[which];
}
public double recall( int which ) {
return get(which,which)/(double)sumCols[which];
}
}
© 2015 - 2024 Weber Informatics LLC | Privacy Policy