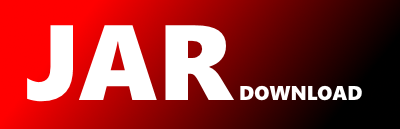
org.deeplearning4j.util.MathUtils Maven / Gradle / Ivy
package org.deeplearning4j.util;
import java.util.ArrayList;
import java.util.List;
import java.util.Random;
import java.util.Set;
import org.apache.commons.math3.linear.CholeskyDecomposition;
import org.apache.commons.math3.linear.NonSquareMatrixException;
import org.apache.commons.math3.linear.RealMatrix;
import org.apache.commons.math3.random.RandomGenerator;
import org.apache.commons.math3.stat.regression.OLSMultipleLinearRegression;
import org.deeplearning4j.berkeley.Counter;
/**
* This is a math utils class.
*
* @author Adam Gibson
*
*/
public class MathUtils {
/** The natural logarithm of 2. */
public static double log2 = Math.log(2);
/**
* Normalize a value
* (val - min) / (max - min)
* @param val value to normalize
* @param max max value
* @param min min value
* @return the normalized value
*/
public static double normalize(double val,double min,double max) {
if(max < min)
throw new IllegalArgumentException("Max must be greather than min");
return (val - min) / (max - min);
}
/**
* Clamps the value to a discrete value
* @param value the value to clamp
* @param min min for the probability distribution
* @param max max for the probability distribution
* @return the discrete value
*/
public static int clamp(int value, int min, int max) {
if (value < min) value = min;
if (value > max) value = max;
return value;
}
/**
* Discretize the given value
* @param value the value to discretize
* @param min the min of the distribution
* @param max the max of the distribution
* @param binCount the number of bins
* @return the discretized value
*/
public static int discretize(double value, double min, double max, int binCount) {
int discreteValue = (int) (binCount * normalize(value, min, max));
return clamp(discreteValue, 0, binCount - 1);
}
/**
* See: http://stackoverflow.com/questions/466204/rounding-off-to-nearest-power-of-2
* @param v the number to getFromOrigin the next power of 2 for
* @return the next power of 2 for the passed in value
*/
public static long nextPowOf2(long v)
{
v--;
v |= v >> 1;
v |= v >> 2;
v |= v >> 4;
v |= v >> 8;
v |= v >> 16;
v++;
return v;
}
/**
* Generates a binomial distributed number using
* the given rng
* @param rng
* @param n
* @param p
* @return
*/
public static int binomial(RandomGenerator rng,int n, double p) {
if ((p < 0) || (p > 1)) {
return 0;
}
int c = 0;
for (int i = 0; i < n; i++) {
if (rng.nextDouble() < p) {
c++;
}
}
return c;
}
/**
* Generate a uniform random number from the given rng
* @param rng the rng to use
* @param min the min num
* @param max the max num
* @return a number uniformly distributed between min and max
*/
public static double uniform(Random rng,double min, double max) {
return rng.nextDouble() * (max - min) + min;
}
/**
* Returns the correlation coefficient of two double vectors.
*
* @param residuals residuals
* @param targetAttribute target attribute vector
*
* @return the correlation coefficient or r
*/
public static double correlation(double[] residuals,double targetAttribute[]) {
double[] predictedValues = new double[residuals.length];
for(int i=0;i counter = new Counter();
Counter counter2 = new Counter();
for(int i = 0; i < strings[0].length(); i++)
counter.incrementCount(String.valueOf(strings[0].charAt(i)), 1.0);
for(int i = 0; i < strings[1].length(); i++)
counter2.incrementCount(String.valueOf(strings[1].charAt(i)), 1.0);
Set v1 = counter.keySet();
Set v2 = counter2.keySet();
Set both = SetUtils.intersection(v1, v2);
double sclar = 0, norm1 = 0, norm2 = 0;
for (String k : both)
sclar += counter.getCount(k) * counter2.getCount(k);
for (String k : v1)
norm1 += counter.getCount(k) * counter.getCount(k);
for (String k : v2)
norm2 += counter2.getCount(k) * counter2.getCount(k);
return sclar / Math.sqrt(norm1 * norm2);
}
/**
* Returns the vector length (sqrt(sum(x_i))
* @param vector the vector to return the vector length for
* @return the vector length of the passed in array
*/
public static double vectorLength(double[] vector) {
double ret=0;
if(vector==null)
return ret;
else {
for(int i=0;i 0 ? Math.log10(totalDocs/numTimesWordAppearedInADocument) : 0;
}
/**
* Term frequency: 1+ log10(count)
* @param count the count of a word or character in a given string or document
* @return 1+ log(10) count
*/
public static double tf(int count) {
return count > 0 ? 1 + Math.log10(count) : 0;
}
/**
* Return td * idf
* @param td the term frequency (assumed calculated)
* @param idf inverse document frequency (assumed calculated)
* @return td * idf
*/
public static double tfidf(double td,double idf) {
return td * idf;
}
private static int charForLetter(char c) {
char[] chars={'a','b','c','d','e','f','g','h','i','j','k','l','m','n','o','p','q','r','s','t','u','v','w','x','y','z'};
for(int i=0;i mergeCoords(List x,List y) {
if(x.size()!=y.size())
throw new IllegalArgumentException("Sample sizes must be the same for each data applyTransformToDestination.");
List ret = new ArrayList();
for(int i=0;i vector) {
/* split coordinate system */
List coords=coordSplit(vector);
/* x vals */
double[] x=coords.get(0);
/* y vals */
double[] y=coords.get(1);
double meanX=sum(x)/x.length;
double meanY=sum(y)/y.length;
double sumOfMeanDifferences=sumOfMeanDifferences(x,y);
double xDifferenceOfMean=sumOfMeanDifferencesOnePoint(x);
double w_1=sumOfMeanDifferences/xDifferenceOfMean;
double w_0=meanY - (w_1) * meanX;
//double w_1=(n*sumOfProducts(x,y) - sum(x) * sum(y))/(n*sumOfSquares(x) - Math.pow(sum(x),2));
// double w_0=(sum(y) - (w_1 * sum(x)))/n;
double[] ret = new double[vector.size()];
ret[0]=w_0;
ret[1]=w_1;
return ret;
}//end weightsFor
/**
* This will return the squared loss of the given
* points
* @param x the x coordinates to use
* @param y the y coordinates to use
* @param w_0 the first weight
*
* @param w_1 the second weight
* @return the squared loss of the given points
*/
public static double squaredLoss(double[] x,double[] y,double w_0,double w_1) {
double sum=0;
for(int j=0;j coords=coordSplit(vector);
/* x vals */
double[] x=coords.get(0);
/* y vals */
double[] y=coords.get(1);
double meanX=sum(x)/x.length;
double meanY=sum(y)/y.length;
double sumOfMeanDifferences=sumOfMeanDifferences(x,y);
double xDifferenceOfMean=sumOfMeanDifferencesOnePoint(x);
double w_1=sumOfMeanDifferences/xDifferenceOfMean;
double w_0=meanY - (w_1) * meanX;
double[] ret = new double[vector.length];
ret[0]=w_0;
ret[1]=w_1;
return ret;
}//end weightsFor
public static double errorFor(double actual,double prediction) {
return actual-prediction;
}
/**
* Used for calculating top part of simple regression for
* beta 1
* @param vector the x coordinates
* @param vector2 the y coordinates
* @return the sum of mean differences for the input vectors
*/
public static double sumOfMeanDifferences(double[] vector,double[] vector2) {
double mean=sum(vector)/vector.length;
double mean2=sum(vector2)/vector2.length;
double ret=0;
for(int i=0;i coordSplit(double[] vector) {
if(vector==null) return null;
List ret = new ArrayList();
/* x coordinates */
double[] xVals = new double[vector.length/2];
/* y coordinates */
double[] yVals = new double[vector.length/2];
/* current points */
int xTracker=0;
int yTracker=0;
for(int i=0;i> partitionVariable(List arr,int chunk) {
int count=0;
List> ret = new ArrayList>();
while(count < arr.size()) {
List sublist=arr.subList(count,count+chunk);
count+=chunk;
ret.add(sublist);
}
//All data sets must be same size
for(List lists : ret) {
if(lists.size() < chunk)
ret.remove(lists);
}
return ret;
}//end partitionVariable
/**
* This returns the coordinate split in a list of coordinates
* such that the values for ret[0] are the x values
* and ret[1] are the y values
* @param vector the vector to split with x and y values
* Note that the list will be more stable due to the size operator.
* The array version will have extraneous values if not monitored
* properly.
* @return a coordinate split for the given vector of values.
* if null, is passed in null is returned
*/
public static List coordSplit(List vector) {
if(vector==null) return null;
List ret = new ArrayList();
/* x coordinates */
double[] xVals = new double[vector.size()/2];
/* y coordinates */
double[] yVals = new double[vector.size()/2];
/* current points */
int xTracker=0;
int yTracker=0;
for(int i=0;i ret )
ret = d;
return ret;
}
/**
* Normalizes the doubles in the array using the given value.
*
* @param doubles the array of double
* @param sum the value by which the doubles are to be normalized
* @exception IllegalArgumentException if sum is zero or NaN
*/
public static void normalize(double[] doubles, double sum) {
if (Double.isNaN(sum)) {
throw new IllegalArgumentException("Can't normalize array. Sum is NaN.");
}
if (sum == 0) {
// Maybe this should just be a return.
throw new IllegalArgumentException("Can't normalize array. Sum is zero.");
}
for (int i = 0; i < doubles.length; i++) {
doubles[i] /= sum;
}
}//end normalize
/**
* Converts an array containing the natural logarithms of
* probabilities stored in a vector back into probabilities.
* The probabilities are assumed to sum to one.
*
* @param a an array holding the natural logarithms of the probabilities
* @return the converted array
*/
public static double[] logs2probs(double[] a) {
double max = a[maxIndex(a)];
double sum = 0.0;
double[] result = new double[a.length];
for(int i = 0; i < a.length; i++) {
result[i] = Math.exp(a[i] - max);
sum += result[i];
}
normalize(result, sum);
return result;
}//end logs2probs
/**
* This returns the entropy for a given vector of probabilities.
* @param probabilities the probabilities to getFromOrigin the entropy for
* @return the entropy of the given probabilities.
*/
public static double information(double[] probabilities) {
double total = 0.0;
for (double d : probabilities) {
total += (-1.0 * log2(d) * d);
}
return total;
}//end information
/**
*
*
* Returns index of maximum element in a given
* array of doubles. First maximum is returned.
*
* @param doubles the array of doubles
* @return the index of the maximum element
*/
public static /*@pure@*/ int maxIndex(double[] doubles) {
double maximum = 0;
int maxIndex = 0;
for (int i = 0; i < doubles.length; i++) {
if ((i == 0) || (doubles[i] > maximum)) {
maxIndex = i;
maximum = doubles[i];
}
}
return maxIndex;
}//end maxIndex
/**
* This will return the factorial of the given number n.
* @param n the number to getFromOrigin the factorial for
* @return the factorial for this number
*/
public static double factorial(double n) {
if(n==1 || n==0) return 1;
for(double i=n;i>0;i--,n*=(i > 0 ? i : 1)) {}
return n;
}//end factorial
/** The small deviation allowed in double comparisons. */
public static double SMALL = 1e-6;
/**
* Returns the log-odds for a given probability.
*
* @param prob the probability
*
* @return the log-odds after the probability has been mapped to
* [Utils.SMALL, 1-Utils.SMALL]
*/
public static /*@pure@*/ double probToLogOdds(double prob) {
if (gr(prob, 1) || (sm(prob, 0))) {
throw new IllegalArgumentException("probToLogOdds: probability must " +
"be in [0,1] "+prob);
}
double p = SMALL + (1.0 - 2 * SMALL) * prob;
return Math.log(p / (1 - p));
}
/**
* Rounds a double to the next nearest integer value. The JDK version
* of it doesn't work properly.
*
* @param value the double value
* @return the resulting integer value
*/
public static /*@pure@*/ int round(double value) {
int roundedValue = value > 0
? (int)(value + 0.5)
: -(int)(Math.abs(value) + 0.5);
return roundedValue;
}//end round
/**
* This returns the permutation of n choose r.
* @param n the n to choose
* @param r the number of elements to choose
* @return the permutation of these numbers
*/
public static double permutation(double n,double r) {
double nFac=MathUtils.factorial(n);
double nMinusRFac=MathUtils.factorial((n-r));
return nFac/nMinusRFac;
}//end permutation
/**
* This returns the combination of n choose r
* @param n the number of elements overall
* @param r the number of elements to choose
* @return the amount of possible combinations for this applyTransformToDestination of elements
*/
public static double combination(double n,double r) {
double nFac=MathUtils.factorial(n);
double rFac=MathUtils.factorial(r);
double nMinusRFac=MathUtils.factorial((n-r));
return nFac/(rFac * nMinusRFac);
}//end combination
/**
* sqrt(a^2 + b^2) without under/overflow.
*/
public static double hypotenuse(double a, double b) {
double r;
if (Math.abs(a) > Math.abs(b)) {
r = b/a;
r = Math.abs(a)*Math.sqrt(1+r*r);
} else if (b != 0) {
r = a/b;
r = Math.abs(b)*Math.sqrt(1+r*r);
} else {
r = 0.0;
}
return r;
}//end hypotenuse
/**
* Rounds a double to the next nearest integer value in a probabilistic
* fashion (e.g. 0.8 has a 20% chance of being rounded down to 0 and a
* 80% chance of being rounded up to 1). In the limit, the average of
* the rounded numbers generated by this procedure should converge to
* the original double.
*
* @param value the double value
* @param rand the random number generator
* @return the resulting integer value
*/
public static int probRound(double value, Random rand) {
if (value >= 0) {
double lower = Math.floor(value);
double prob = value - lower;
if (rand.nextDouble() < prob) {
return (int)lower + 1;
} else {
return (int)lower;
}
} else {
double lower = Math.floor(Math.abs(value));
double prob = Math.abs(value) - lower;
if (rand.nextDouble() < prob) {
return -((int)lower + 1);
} else {
return -(int)lower;
}
}
}//end probRound
/**
* Rounds a double to the given number of decimal places.
*
* @param value the double value
* @param afterDecimalPoint the number of digits after the decimal point
* @return the double rounded to the given precision
*/
public static /*@pure@*/ double roundDouble(double value,int afterDecimalPoint) {
double mask = Math.pow(10.0, (double)afterDecimalPoint);
return (double)(Math.round(value * mask)) / mask;
}//end roundDouble
/**
* Rounds a double to the given number of decimal places.
*
* @param value the double value
* @param afterDecimalPoint the number of digits after the decimal point
* @return the double rounded to the given precision
*/
public static /*@pure@*/ float roundFloat(float value,int afterDecimalPoint) {
float mask = (float) Math.pow(10, (float) afterDecimalPoint);
return (float)(Math.round(value * mask)) / mask;
}//end roundDouble
/**
* This will return the bernoulli trial for the given event.
* A bernoulli trial is a mechanism for detecting the probability
* of a given event occurring k times in n independent trials
* @param n the number of trials
* @param k the number of times the target event occurs
* @param successProb the probability of the event happening
* @return the probability of the given event occurring k times.
*/
public static double bernoullis(double n,double k,double successProb) {
double combo = MathUtils.combination(n, k);
double p = successProb;
double q= 1 - successProb;
return combo * Math.pow(p,k) * Math.pow(q,n-k);
}//end bernoullis
/**
* Tests if a is smaller than b.
*
* @param a a double
* @param b a double
*/
public static /*@pure@*/ boolean sm(double a,double b) {
return (b-a > SMALL);
}
/**
* Tests if a is greater than b.
*
* @param a a double
* @param b a double
*/
public static /*@pure@*/ boolean gr(double a,double b) {
return (a-b > SMALL);
}
/**
* This will take a given string and separator and convert it to an equivalent
* double array.
* @param data the data to separate
* @param separator the separator to use
* @return the new double array based on the given data
*/
public static double[] fromString(String data,String separator) {
String[] split=data.split(separator);
double[] ret = new double[split.length];
for(int i=0;i 0){
rem = num % 10;
num = num / 10;
if(rem != 0 && rem != 1){
System.out.println("This is not a binary number.");
System.out.println("Please try once again.");
return -1;
}
}
int i= Integer.parseInt(binary,2);
return i;
}//end toDecimal
/**
* This will translate a vector in to an equivalent integer
* @param vector the vector to translate
* @return a z value such that the value is the interleaved lsd to msd for each
* double in the vector
*/
public static int distanceFinderZValue(double[] vector) {
StringBuffer binaryBuffer =new StringBuffer();
List binaryReps = new ArrayList(vector.length);
for(int i=0;i end)
throw new IllegalArgumentException("Begin must not be less than end");
return (int)begin + (int)(Math.random() * ((end - begin) + 1));
}
/**
* Generates a random integer between the specified numbers
* @param begin the begin of the interval
* @param end the end of the interval
* @return an int between begin and end
*/
public static int randomNumberBetween(double begin,double end,RandomGenerator rng) {
if(begin > end)
throw new IllegalArgumentException("Begin must not be less than end");
return (int)begin + (int)(rng.nextDouble() * ((end - begin) + 1));
}
public static float randomFloatBetween(float begin,float end) {
float rand = (float) Math.random();
return begin + (rand * ((end - begin)));
}
public static double randomDoubleBetween(double begin,double end) {
return begin + (Math.random() * ((end - begin)));
}
}
© 2015 - 2025 Weber Informatics LLC | Privacy Policy