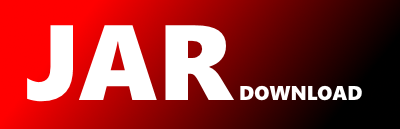
org.deeplearning4j.zoo.model.VGG19 Maven / Gradle / Ivy
/*
* ******************************************************************************
* *
* *
* * This program and the accompanying materials are made available under the
* * terms of the Apache License, Version 2.0 which is available at
* * https://www.apache.org/licenses/LICENSE-2.0.
* *
* * See the NOTICE file distributed with this work for additional
* * information regarding copyright ownership.
* * Unless required by applicable law or agreed to in writing, software
* * distributed under the License is distributed on an "AS IS" BASIS, WITHOUT
* * WARRANTIES OR CONDITIONS OF ANY KIND, either express or implied. See the
* * License for the specific language governing permissions and limitations
* * under the License.
* *
* * SPDX-License-Identifier: Apache-2.0
* *****************************************************************************
*/
package org.deeplearning4j.zoo.model;
import lombok.AllArgsConstructor;
import lombok.Builder;
import lombok.NoArgsConstructor;
import org.deeplearning4j.common.resources.DL4JResources;
import org.deeplearning4j.nn.api.Model;
import org.deeplearning4j.nn.api.OptimizationAlgorithm;
import org.deeplearning4j.nn.conf.*;
import org.deeplearning4j.nn.conf.inputs.InputType;
import org.deeplearning4j.nn.conf.layers.ConvolutionLayer;
import org.deeplearning4j.nn.conf.layers.DenseLayer;
import org.deeplearning4j.nn.conf.layers.OutputLayer;
import org.deeplearning4j.nn.conf.layers.SubsamplingLayer;
import org.deeplearning4j.nn.graph.ComputationGraph;
import org.deeplearning4j.nn.multilayer.MultiLayerNetwork;
import org.deeplearning4j.zoo.ModelMetaData;
import org.deeplearning4j.zoo.PretrainedType;
import org.deeplearning4j.zoo.ZooModel;
import org.deeplearning4j.zoo.ZooType;
import org.nd4j.linalg.activations.Activation;
import org.nd4j.linalg.learning.config.IUpdater;
import org.nd4j.linalg.learning.config.Nesterovs;
import org.nd4j.linalg.lossfunctions.LossFunctions;
@AllArgsConstructor
@Builder
public class VGG19 extends ZooModel {
@Builder.Default private long seed = 1234;
@Builder.Default private int[] inputShape = new int[] {3, 224, 224};
@Builder.Default private int numClasses = 0;
@Builder.Default private IUpdater updater = new Nesterovs();
@Builder.Default private CacheMode cacheMode = CacheMode.NONE;
@Builder.Default private WorkspaceMode workspaceMode = WorkspaceMode.ENABLED;
@Builder.Default private ConvolutionLayer.AlgoMode cudnnAlgoMode = ConvolutionLayer.AlgoMode.NO_WORKSPACE;
private VGG19() {}
@Override
public String pretrainedUrl(PretrainedType pretrainedType) {
if (pretrainedType == PretrainedType.IMAGENET)
return DL4JResources.getURLString("models/vgg19_dl4j_inference.zip");
else
return null;
}
@Override
public long pretrainedChecksum(PretrainedType pretrainedType) {
if (pretrainedType == PretrainedType.IMAGENET)
return 2782932419L;
else
return 0L;
}
@Override
public Class extends Model> modelType() {
return ComputationGraph.class;
}
public ComputationGraphConfiguration conf() {
ComputationGraphConfiguration conf =
new NeuralNetConfiguration.Builder().seed(seed)
.optimizationAlgo(OptimizationAlgorithm.STOCHASTIC_GRADIENT_DESCENT)
.updater(updater)
.activation(Activation.RELU)
.cacheMode(cacheMode)
.trainingWorkspaceMode(workspaceMode)
.inferenceWorkspaceMode(workspaceMode)
.graphBuilder()
.addInputs("in")
// block 1
.layer(0, new ConvolutionLayer.Builder().kernelSize(3, 3).stride(1, 1)
.padding(1, 1).nIn(inputShape[0]).nOut(64)
.cudnnAlgoMode(cudnnAlgoMode).build(), "in")
.layer(1, new ConvolutionLayer.Builder().kernelSize(3, 3).stride(1, 1)
.padding(1, 1).nOut(64).cudnnAlgoMode(cudnnAlgoMode).build(), "0")
.layer(2, new SubsamplingLayer.Builder()
.poolingType(SubsamplingLayer.PoolingType.MAX).kernelSize(2, 2)
.stride(2, 2).build(), "1")
// block 2
.layer(3, new ConvolutionLayer.Builder().kernelSize(3, 3).stride(1, 1)
.padding(1, 1).nOut(128).cudnnAlgoMode(cudnnAlgoMode).build(), "2")
.layer(4, new ConvolutionLayer.Builder().kernelSize(3, 3).stride(1, 1)
.padding(1, 1).nOut(128).cudnnAlgoMode(cudnnAlgoMode).build(), "3")
.layer(5, new SubsamplingLayer.Builder()
.poolingType(SubsamplingLayer.PoolingType.MAX).kernelSize(2, 2)
.stride(2, 2).build(), "4")
// block 3
.layer(6, new ConvolutionLayer.Builder().kernelSize(3, 3).stride(1, 1)
.padding(1, 1).nOut(256).cudnnAlgoMode(cudnnAlgoMode).build(), "5")
.layer(7, new ConvolutionLayer.Builder().kernelSize(3, 3).stride(1, 1)
.padding(1, 1).nOut(256).cudnnAlgoMode(cudnnAlgoMode).build(), "6")
.layer(8, new ConvolutionLayer.Builder().kernelSize(3, 3).stride(1, 1)
.padding(1, 1).nOut(256).cudnnAlgoMode(cudnnAlgoMode).build(), "7")
.layer(9, new ConvolutionLayer.Builder().kernelSize(3, 3).stride(1, 1)
.padding(1, 1).nOut(256).cudnnAlgoMode(cudnnAlgoMode).build(), "8")
.layer(10, new SubsamplingLayer.Builder()
.poolingType(SubsamplingLayer.PoolingType.MAX).kernelSize(2, 2)
.stride(2, 2).build(), "9")
// block 4
.layer(11, new ConvolutionLayer.Builder().kernelSize(3, 3).stride(1, 1)
.padding(1, 1).nOut(512).cudnnAlgoMode(cudnnAlgoMode).build(), "10")
.layer(12, new ConvolutionLayer.Builder().kernelSize(3, 3).stride(1, 1)
.padding(1, 1).nOut(512).cudnnAlgoMode(cudnnAlgoMode).build(), "11")
.layer(13, new ConvolutionLayer.Builder().kernelSize(3, 3).stride(1, 1)
.padding(1, 1).nOut(512).cudnnAlgoMode(cudnnAlgoMode).build(), "12")
.layer(14, new ConvolutionLayer.Builder().kernelSize(3, 3).stride(1, 1)
.padding(1, 1).nOut(512).cudnnAlgoMode(cudnnAlgoMode).build(), "13")
.layer(15, new SubsamplingLayer.Builder()
.poolingType(SubsamplingLayer.PoolingType.MAX).kernelSize(2, 2)
.stride(2, 2).build(), "14")
// block 5
.layer(16, new ConvolutionLayer.Builder().kernelSize(3, 3).stride(1, 1)
.padding(1, 1).nOut(512).cudnnAlgoMode(cudnnAlgoMode).build(), "15")
.layer(17, new ConvolutionLayer.Builder().kernelSize(3, 3).stride(1, 1)
.padding(1, 1).nOut(512).cudnnAlgoMode(cudnnAlgoMode).build(), "16")
.layer(18, new ConvolutionLayer.Builder().kernelSize(3, 3).stride(1, 1)
.padding(1, 1).nOut(512).cudnnAlgoMode(cudnnAlgoMode).build(), "17")
.layer(19, new ConvolutionLayer.Builder().kernelSize(3, 3).stride(1, 1)
.padding(1, 1).nOut(512).cudnnAlgoMode(cudnnAlgoMode).build(), "18")
.layer(20, new SubsamplingLayer.Builder()
.poolingType(SubsamplingLayer.PoolingType.MAX).kernelSize(2, 2)
.stride(2, 2).build(), "19")
.layer(21, new DenseLayer.Builder().nOut(4096).build(), "20")
.layer(22, new OutputLayer.Builder(
LossFunctions.LossFunction.NEGATIVELOGLIKELIHOOD).name("output")
.nOut(numClasses).activation(Activation.SOFTMAX) // radial basis function required
.build(), "21")
.setOutputs("22")
.setInputTypes(InputType.convolutionalFlat(inputShape[2], inputShape[1], inputShape[0]))
.build();
return conf;
}
@Override
public ComputationGraph init() {
ComputationGraph network = new ComputationGraph(conf());
network.init();
return network;
}
@Override
public ModelMetaData metaData() {
return new ModelMetaData(new int[][] {inputShape}, 1, ZooType.CNN);
}
@Override
public void setInputShape(int[][] inputShape) {
this.inputShape = inputShape[0];
}
}
© 2015 - 2024 Weber Informatics LLC | Privacy Policy