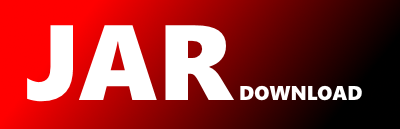
org.deeplearning4j.zoo.model.ResNet50 Maven / Gradle / Ivy
package org.deeplearning4j.zoo.model;
import lombok.AllArgsConstructor;
import lombok.Builder;
import lombok.NoArgsConstructor;
import org.deeplearning4j.nn.api.Model;
import org.deeplearning4j.nn.api.OptimizationAlgorithm;
import org.deeplearning4j.nn.conf.*;
import org.deeplearning4j.nn.conf.distribution.NormalDistribution;
import org.deeplearning4j.nn.conf.distribution.TruncatedNormalDistribution;
import org.deeplearning4j.nn.conf.graph.ElementWiseVertex;
import org.deeplearning4j.nn.conf.inputs.InputType;
import org.deeplearning4j.nn.conf.layers.*;
import org.deeplearning4j.nn.graph.ComputationGraph;
import org.deeplearning4j.nn.weights.WeightInit;
import org.deeplearning4j.zoo.ModelMetaData;
import org.deeplearning4j.zoo.PretrainedType;
import org.deeplearning4j.zoo.ZooModel;
import org.deeplearning4j.zoo.ZooType;
import org.nd4j.linalg.activations.Activation;
import org.nd4j.linalg.learning.config.IUpdater;
import org.nd4j.linalg.learning.config.RmsProp;
import org.nd4j.linalg.lossfunctions.LossFunctions;
/**
* Residual networks for deep learning.
*
* Paperp: https://arxiv.org/abs/1512.03385
* ImageNet weights for this model are available and have been converted from https://keras.io/applications/.
*
* @author Justin Long (crockpotveggies)
*/
@AllArgsConstructor
@Builder
public class ResNet50 extends ZooModel {
@Builder.Default private long seed = 1234;
@Builder.Default private int[] inputShape = new int[] {3, 224, 224};
@Builder.Default private int numClasses = 0;
@Builder.Default private WeightInit weightInit = WeightInit.DISTRIBUTION;
@Builder.Default private IUpdater updater = new RmsProp(0.1, 0.96, 0.001);
@Builder.Default private CacheMode cacheMode = CacheMode.NONE;
@Builder.Default private WorkspaceMode workspaceMode = WorkspaceMode.ENABLED;
@Builder.Default private ConvolutionLayer.AlgoMode cudnnAlgoMode = ConvolutionLayer.AlgoMode.PREFER_FASTEST;
private ResNet50() {}
@Override
public String pretrainedUrl(PretrainedType pretrainedType) {
if (pretrainedType == PretrainedType.IMAGENET)
return "http://blob.deeplearning4j.org/models/resnet50_dl4j_inference.v3.zip";
else
return null;
}
@Override
public long pretrainedChecksum(PretrainedType pretrainedType) {
if (pretrainedType == PretrainedType.IMAGENET)
return 3914447815L;
else
return 0L;
}
@Override
public Class extends Model> modelType() {
return ComputationGraph.class;
}
@Override
public ComputationGraph init() {
ComputationGraphConfiguration.GraphBuilder graph = graphBuilder();
ComputationGraphConfiguration conf = graph.build();
ComputationGraph model = new ComputationGraph(conf);
model.init();
return model;
}
private void identityBlock(ComputationGraphConfiguration.GraphBuilder graph, int[] kernelSize, int[] filters,
String stage, String block, String input) {
String convName = "res" + stage + block + "_branch";
String batchName = "bn" + stage + block + "_branch";
String activationName = "act" + stage + block + "_branch";
String shortcutName = "short" + stage + block + "_branch";
graph.addLayer(convName + "2a",
new ConvolutionLayer.Builder(new int[] {1, 1}).nOut(filters[0]).cudnnAlgoMode(cudnnAlgoMode)
.build(),
input)
.addLayer(batchName + "2a", new BatchNormalization(), convName + "2a")
.addLayer(activationName + "2a",
new ActivationLayer.Builder().activation(Activation.RELU).build(),
batchName + "2a")
.addLayer(convName + "2b", new ConvolutionLayer.Builder(kernelSize).nOut(filters[1])
.cudnnAlgoMode(cudnnAlgoMode).convolutionMode(ConvolutionMode.Same).build(),
activationName + "2a")
.addLayer(batchName + "2b", new BatchNormalization(), convName + "2b")
.addLayer(activationName + "2b",
new ActivationLayer.Builder().activation(Activation.RELU).build(),
batchName + "2b")
.addLayer(convName + "2c",
new ConvolutionLayer.Builder(new int[] {1, 1}).nOut(filters[2])
.cudnnAlgoMode(cudnnAlgoMode).build(),
activationName + "2b")
.addLayer(batchName + "2c", new BatchNormalization(), convName + "2c")
.addVertex(shortcutName, new ElementWiseVertex(ElementWiseVertex.Op.Add), batchName + "2c",
input)
.addLayer(convName, new ActivationLayer.Builder().activation(Activation.RELU).build(),
shortcutName);
}
private void convBlock(ComputationGraphConfiguration.GraphBuilder graph, int[] kernelSize, int[] filters,
String stage, String block, String input) {
convBlock(graph, kernelSize, filters, stage, block, new int[] {2, 2}, input);
}
private void convBlock(ComputationGraphConfiguration.GraphBuilder graph, int[] kernelSize, int[] filters,
String stage, String block, int[] stride, String input) {
String convName = "res" + stage + block + "_branch";
String batchName = "bn" + stage + block + "_branch";
String activationName = "act" + stage + block + "_branch";
String shortcutName = "short" + stage + block + "_branch";
graph.addLayer(convName + "2a", new ConvolutionLayer.Builder(new int[] {1, 1}, stride).nOut(filters[0]).build(),
input)
.addLayer(batchName + "2a", new BatchNormalization(), convName + "2a")
.addLayer(activationName + "2a",
new ActivationLayer.Builder().activation(Activation.RELU).build(),
batchName + "2a")
.addLayer(convName + "2b",
new ConvolutionLayer.Builder(kernelSize).nOut(filters[1])
.convolutionMode(ConvolutionMode.Same).build(),
activationName + "2a")
.addLayer(batchName + "2b", new BatchNormalization(), convName + "2b")
.addLayer(activationName + "2b",
new ActivationLayer.Builder().activation(Activation.RELU).build(),
batchName + "2b")
.addLayer(convName + "2c",
new ConvolutionLayer.Builder(new int[] {1, 1}).nOut(filters[2]).build(),
activationName + "2b")
.addLayer(batchName + "2c", new BatchNormalization(), convName + "2c")
// shortcut
.addLayer(convName + "1",
new ConvolutionLayer.Builder(new int[] {1, 1}, stride).nOut(filters[2]).build(),
input)
.addLayer(batchName + "1", new BatchNormalization(), convName + "1")
.addVertex(shortcutName, new ElementWiseVertex(ElementWiseVertex.Op.Add), batchName + "2c",
batchName + "1")
.addLayer(convName, new ActivationLayer.Builder().activation(Activation.RELU).build(),
shortcutName);
}
public ComputationGraphConfiguration.GraphBuilder graphBuilder() {
ComputationGraphConfiguration.GraphBuilder graph = new NeuralNetConfiguration.Builder().seed(seed)
.activation(Activation.IDENTITY)
.optimizationAlgo(OptimizationAlgorithm.STOCHASTIC_GRADIENT_DESCENT)
.updater(updater)
.weightInit(weightInit)
.dist(new TruncatedNormalDistribution(0.0, 0.5))
.l1(1e-7)
.l2(5e-5)
.miniBatch(true)
.cacheMode(cacheMode)
.trainingWorkspaceMode(workspaceMode)
.inferenceWorkspaceMode(workspaceMode)
.cudnnAlgoMode(cudnnAlgoMode)
.convolutionMode(ConvolutionMode.Truncate)
.graphBuilder();
graph.addInputs("input").setInputTypes(InputType.convolutional(inputShape[2], inputShape[1], inputShape[0]))
// stem
.addLayer("stem-zero", new ZeroPaddingLayer.Builder(3, 3).build(), "input")
.addLayer("stem-cnn1",
new ConvolutionLayer.Builder(new int[] {7, 7}, new int[] {2, 2}).nOut(64)
.build(),
"stem-zero")
.addLayer("stem-batch1", new BatchNormalization(), "stem-cnn1")
.addLayer("stem-act1", new ActivationLayer.Builder().activation(Activation.RELU).build(),
"stem-batch1")
.addLayer("stem-maxpool1", new SubsamplingLayer.Builder(SubsamplingLayer.PoolingType.MAX,
new int[] {3, 3}, new int[] {2, 2}).build(), "stem-act1");
convBlock(graph, new int[] {3, 3}, new int[] {64, 64, 256}, "2", "a", new int[] {2, 2}, "stem-maxpool1");
identityBlock(graph, new int[] {3, 3}, new int[] {64, 64, 256}, "2", "b", "res2a_branch");
identityBlock(graph, new int[] {3, 3}, new int[] {64, 64, 256}, "2", "c", "res2b_branch");
convBlock(graph, new int[] {3, 3}, new int[] {128, 128, 512}, "3", "a", "res2c_branch");
identityBlock(graph, new int[] {3, 3}, new int[] {128, 128, 512}, "3", "b", "res3a_branch");
identityBlock(graph, new int[] {3, 3}, new int[] {128, 128, 512}, "3", "c", "res3b_branch");
identityBlock(graph, new int[] {3, 3}, new int[] {128, 128, 512}, "3", "d", "res3c_branch");
convBlock(graph, new int[] {3, 3}, new int[] {256, 256, 1024}, "4", "a", "res3d_branch");
identityBlock(graph, new int[] {3, 3}, new int[] {256, 256, 1024}, "4", "b", "res4a_branch");
identityBlock(graph, new int[] {3, 3}, new int[] {256, 256, 1024}, "4", "c", "res4b_branch");
identityBlock(graph, new int[] {3, 3}, new int[] {256, 256, 1024}, "4", "d", "res4c_branch");
identityBlock(graph, new int[] {3, 3}, new int[] {256, 256, 1024}, "4", "e", "res4d_branch");
identityBlock(graph, new int[] {3, 3}, new int[] {256, 256, 1024}, "4", "f", "res4e_branch");
convBlock(graph, new int[] {3, 3}, new int[] {512, 512, 2048}, "5", "a", "res4f_branch");
identityBlock(graph, new int[] {3, 3}, new int[] {512, 512, 2048}, "5", "b", "res5a_branch");
identityBlock(graph, new int[] {3, 3}, new int[] {512, 512, 2048}, "5", "c", "res5b_branch");
graph.addLayer("avgpool",
new SubsamplingLayer.Builder(SubsamplingLayer.PoolingType.MAX, new int[] {3, 3}).build(),
"res5c_branch")
// TODO add flatten/reshape layer here
.addLayer("output",
new OutputLayer.Builder(LossFunctions.LossFunction.NEGATIVELOGLIKELIHOOD)
.nOut(numClasses).activation(Activation.SOFTMAX).build(),
"avgpool")
.setOutputs("output").backprop(true).pretrain(false);
return graph;
}
@Override
public ModelMetaData metaData() {
return new ModelMetaData(new int[][] {inputShape}, 1, ZooType.CNN);
}
@Override
public void setInputShape(int[][] inputShape) {
this.inputShape = inputShape[0];
}
}
© 2015 - 2024 Weber Informatics LLC | Privacy Policy