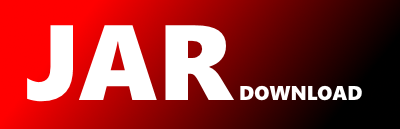
georegression.fitting.curves.FitEllipseWeightedAlgebraic_F64 Maven / Gradle / Ivy
/*
* Copyright (C) 2021, Peter Abeles. All Rights Reserved.
*
* This file is part of Geometric Regression Library (GeoRegression).
*
* Licensed under the Apache License, Version 2.0 (the "License");
* you may not use this file except in compliance with the License.
* You may obtain a copy of the License at
*
* http://www.apache.org/licenses/LICENSE-2.0
*
* Unless required by applicable law or agreed to in writing, software
* distributed under the License is distributed on an "AS IS" BASIS,
* WITHOUT WARRANTIES OR CONDITIONS OF ANY KIND, either express or implied.
* See the License for the specific language governing permissions and
* limitations under the License.
*/
package georegression.fitting.curves;
import georegression.struct.curve.EllipseQuadratic_F64;
import georegression.struct.point.Point2D_F64;
import org.ejml.data.DMatrixRMaj;
import org.ejml.dense.row.CommonOps_DDRM;
import org.ejml.dense.row.factory.DecompositionFactory_DDRM;
import org.ejml.dense.row.factory.LinearSolverFactory_DDRM;
import org.ejml.interfaces.decomposition.EigenDecomposition;
import org.ejml.interfaces.linsol.LinearSolverDense;
import org.jetbrains.annotations.Nullable;
import java.util.List;
/**
*
* Fits an ellipse to a set of points in "closed form" by minimizing algebraic least-squares error. The method used is
* described in [1] and is a repartitioning of the solution describe in [2], with the aim of improving numerical
* stability. The found ellipse is described using 6 coefficients, as is shown below.
* {@code F(x,y) = a*x^2 + 2*b*x*y + c*y^2 + 2*d*x + 2*e*y + f = 0 and b^2 - 4*ac < 0}
*
*
*
* One peculiarity of this algorithm is that it's less stable when perfect data is provided. This instability became
* evident when constructing unit tests and some of them failed. Tests on the original Matlab code also failed.
*
*
*
* - [1] Radim Halir and Jan Flusser, "Numerically Stable Direct Least Squares Fitting Of Ellipses" 1998
* - [2] Fitzgibbon, A. W., Pilu, M and Fischer, R. B.: "Direct least squares fitting of ellipses"
* Technical Report DAIRP-794, Department of Artificial Intelligence, The University of Edinburgh, January 1996
*
*
* @author Peter Abeles
*/
public class FitEllipseWeightedAlgebraic_F64 {
// qudratic part of design matrix
private DMatrixRMaj D1 = new DMatrixRMaj(3,1);
// linear part of design matrix
private DMatrixRMaj D2 = new DMatrixRMaj(3,1);
// quadratic part of scatter matrix
private DMatrixRMaj S1 = new DMatrixRMaj(3,3);
// combined part of scatter matrix
private DMatrixRMaj S2 = new DMatrixRMaj(3,3);
//linear part of scatter matrix
private DMatrixRMaj S3 = new DMatrixRMaj(3,3);
// Reduced scatter matrix
private DMatrixRMaj M = new DMatrixRMaj(3,3);
// storage for intermediate steps
private DMatrixRMaj T = new DMatrixRMaj(3,3);
private DMatrixRMaj Ta1 = new DMatrixRMaj(3,1);
private DMatrixRMaj S2_tran = new DMatrixRMaj(3,3);
private LinearSolverDense solver = LinearSolverFactory_DDRM.linear(3);
private EigenDecomposition eigen = DecompositionFactory_DDRM.eig(3,true,false);
private EllipseQuadratic_F64 ellipse = new EllipseQuadratic_F64();
/**
* Fits the ellipse to the line
*
* @param points Set of points that are to be fit
* @param weights Weight or importance of each point. Each weight must be a positive number
* @return true if successful or false if it failed
*/
public boolean process( List points , double weights[] ) {
if( points.size() > weights.length ) {
throw new IllegalArgumentException("Weights must be as long as the number of points. "+
points.size()+" vs "+weights.length);
}
int N = points.size();
// Construct the design matrices. linear and quadratic
D1.reshape(N,3); D2.reshape(N,3);
int index = 0;
for( int i = 0; i < N; i++ ) {
Point2D_F64 p = points.get(i);
double w = weights[i];
// fill in each row one at a time
D1.data[index] = w*p.x*p.x;
D2.data[index++] = w*p.x;
D1.data[index] = w*p.x*p.y;
D2.data[index++] = w*p.y;
D1.data[index] = w*p.y*p.y;
D2.data[index++] = w;
}
// Compute scatter matrix
CommonOps_DDRM.multTransA(D1, D1, S1); // S1 = D1'*D1
CommonOps_DDRM.multTransA(D1, D2, S2); // S2 = D1'*D2
CommonOps_DDRM.multTransA(D2, D2, S3); // S3 = D2'*D2
// for getting a2 from a1
// T = -inv(S3)*S2'
if( !solver.setA(S3) )
return false;
CommonOps_DDRM.transpose(S2,S2_tran);
CommonOps_DDRM.changeSign(S2_tran);
solver.solve(S2_tran, T);
// Compute reduced scatter matrix
// M = S1 + S2*T
CommonOps_DDRM.mult(S2, T, M);
CommonOps_DDRM.add(M,S1,M);
// Premultiply by inv(C1). inverse of constraint matrix
for( int col = 0; col < 3; col++ ) {
double m0 = M.unsafe_get(0, col);
double m1 = M.unsafe_get(1, col);
double m2 = M.unsafe_get(2, col);
M.unsafe_set(0,col, m2 / 2);
M.unsafe_set(1,col, -m1);
M.unsafe_set(2,col, m0 / 2);
}
if( !eigen.decompose(M) )
return false;
DMatrixRMaj a1 = selectBestEigenVector();
if( a1 == null )
return false;
// ellipse coefficients
CommonOps_DDRM.mult(T,a1,Ta1);
ellipse.A = a1.data[0];
ellipse.B = a1.data[1]/2;
ellipse.C = a1.data[2];
ellipse.D = Ta1.data[0]/2;
ellipse.E = Ta1.data[1]/2;
ellipse.F = Ta1.data[2];
return true;
}
private @Nullable DMatrixRMaj selectBestEigenVector() {
int bestIndex = -1;
double bestCond = Double.MAX_VALUE;
for( int i = 0; i < eigen.getNumberOfEigenvalues(); i++ ) {
DMatrixRMaj v = eigen.getEigenVector(i);
if( v == null ) // TODO WTF?!?!
continue;
// evaluate a'*C*a = 1
double cond = 4*v.get(0)*v.get(2) - v.get(1)*v.get(1);
double condError = (cond - 1)*(cond - 1);
if( cond > 0 && condError < bestCond ) {
bestCond = condError;
bestIndex = i;
}
}
if( bestIndex == -1 )
return null;
return eigen.getEigenVector(bestIndex);
}
public EllipseQuadratic_F64 getEllipse() {
return ellipse;
}
}
© 2015 - 2025 Weber Informatics LLC | Privacy Policy