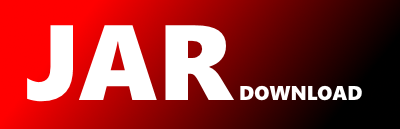
org.neo4j.gds.embeddings.graphsage.BatchSampler Maven / Gradle / Ivy
Go to download
Show more of this group Show more artifacts with this name
Show all versions of algo Show documentation
Show all versions of algo Show documentation
Neo4j Graph Data Science :: Algorithms
The newest version!
/*
* Copyright (c) "Neo4j"
* Neo4j Sweden AB [http://neo4j.com]
*
* This file is part of Neo4j.
*
* Neo4j is free software: you can redistribute it and/or modify
* it under the terms of the GNU General Public License as published by
* the Free Software Foundation, either version 3 of the License, or
* (at your option) any later version.
*
* This program is distributed in the hope that it will be useful,
* but WITHOUT ANY WARRANTY; without even the implied warranty of
* MERCHANTABILITY or FITNESS FOR A PARTICULAR PURPOSE. See the
* GNU General Public License for more details.
*
* You should have received a copy of the GNU General Public License
* along with this program. If not, see .
*/
package org.neo4j.gds.embeddings.graphsage;
import com.carrotsearch.hppc.LongHashSet;
import org.apache.commons.lang3.mutable.MutableLong;
import org.neo4j.gds.api.Graph;
import org.neo4j.gds.api.IdMap;
import org.neo4j.gds.api.properties.relationships.ImmutableRelationshipCursor;
import org.neo4j.gds.core.utils.partition.Partition;
import org.neo4j.gds.core.utils.partition.PartitionUtils;
import org.neo4j.gds.core.utils.progress.tasks.ProgressTracker;
import org.neo4j.gds.ml.core.samplers.WeightedUniformSampler;
import org.neo4j.gds.termination.TerminationFlag;
import java.util.Arrays;
import java.util.List;
import java.util.SplittableRandom;
import java.util.stream.LongStream;
final class BatchSampler {
public static final double DEGREE_SMOOTHING_FACTOR = 0.75;
private final Graph graph;
private final ProgressTracker progressTracker;
private final TerminationFlag terminationFlag;
BatchSampler(Graph graph, ProgressTracker progressTracker, TerminationFlag terminationFlag) {
this.graph = graph;
this.progressTracker = progressTracker;
this.terminationFlag = terminationFlag;
}
List extendedBatches(int batchSize, int searchDepth, long randomSeed) {
return PartitionUtils.rangePartitionWithBatchSize(
graph.nodeCount(),
batchSize,
batch -> {
terminationFlag.assertRunning();
var localSeed = Math.toIntExact(Math.floorDiv(batch.startNode(), graph.nodeCount())) + randomSeed;
long[] extendedBatch = sampleNeighborAndNegativeNodePerBatchNode(batch, searchDepth, localSeed);
progressTracker.logProgress();
return extendedBatch;
}
);
}
/**
* For each node in the batch we sample one neighbor node and one negative node from the graph.
*/
long[] sampleNeighborAndNegativeNodePerBatchNode(Partition batch, int searchDepth, long randomSeed) {
var neighbours = neighborBatch(batch, randomSeed, searchDepth);
LongStream negativeSamples = negativeBatch(Math.toIntExact(batch.nodeCount()), neighbours, randomSeed);
return LongStream.concat(
batch.stream(),
LongStream.concat(
Arrays.stream(neighbours),
// batch.nodeCount is <= config.batchsize (which is an int)
negativeSamples
)
).toArray();
}
long[] neighborBatch(Partition batch, long batchLocalSeed, int searchDepth) {
int iBatchSize = Math.toIntExact(batch.nodeCount());
var neighbors = new long[iBatchSize];
var localRandom = new SplittableRandom(batchLocalSeed);
// sample a neighbor for each batchNode
var batchOffset = batch.startNode();
for (int idx = 0; idx < iBatchSize; idx++) {
var nodeId = batchOffset + idx;
// randomWalk with at most maxSearchDepth steps and only save last node
int actualSearchDepth = localRandom.nextInt(searchDepth) + 1;
var currentNode = new MutableLong(nodeId);
while (actualSearchDepth > 0) {
int degree = graph.degree(currentNode.longValue());
if (degree != 0) {
var sampledIdx = localRandom.nextInt(degree);
var nextNode = graph.nthTarget(currentNode.longValue(), sampledIdx);
assert nextNode != IdMap.NOT_FOUND : "The offset '" + sampledIdx + "' is bound by the degree but no target could be found for nodeId " + currentNode.longValue();
currentNode.setValue(nextNode);
} else {
// terminate
actualSearchDepth = 0;
}
actualSearchDepth--;
}
neighbors[idx] = currentNode.longValue();
}
return neighbors;
}
// get a negative sample per node in batch
LongStream negativeBatch(int batchSize, long[] batchNeighbors, long batchLocalRandomSeed) {
long nodeCount = graph.nodeCount();
var sampler = new WeightedUniformSampler(batchLocalRandomSeed);
// avoid sampling the sampled neighbor as a negative example
var neighborsSet = new LongHashSet(batchNeighbors.length);
neighborsSet.addAll(batchNeighbors);
// each node should be possible to sample
// therefore we need fictive rels to all nodes
// Math.log to avoid always sampling the high degree nodes
var degreeWeightedNodes = LongStream.range(0, nodeCount)
.mapToObj(nodeId -> ImmutableRelationshipCursor.of(0, nodeId, Math.pow(graph.degree(nodeId),
DEGREE_SMOOTHING_FACTOR
)));
return sampler.sample(degreeWeightedNodes, nodeCount, batchSize, sample -> !neighborsSet.contains(sample));
}
}
© 2015 - 2025 Weber Informatics LLC | Privacy Policy