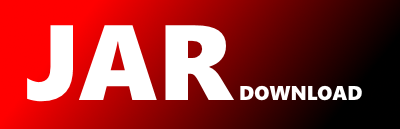
org.neo4j.gds.embeddings.hashgnn.HashGNN Maven / Gradle / Ivy
/*
* Copyright (c) "Neo4j"
* Neo4j Sweden AB [http://neo4j.com]
*
* This file is part of Neo4j.
*
* Neo4j is free software: you can redistribute it and/or modify
* it under the terms of the GNU General Public License as published by
* the Free Software Foundation, either version 3 of the License, or
* (at your option) any later version.
*
* This program is distributed in the hope that it will be useful,
* but WITHOUT ANY WARRANTY; without even the implied warranty of
* MERCHANTABILITY or FITNESS FOR A PARTICULAR PURPOSE. See the
* GNU General Public License for more details.
*
* You should have received a copy of the GNU General Public License
* along with this program. If not, see .
*/
package org.neo4j.gds.embeddings.hashgnn;
import org.apache.commons.lang3.mutable.MutableLong;
import org.neo4j.gds.Algorithm;
import org.neo4j.gds.api.Graph;
import org.neo4j.gds.api.properties.nodes.NodePropertyValues;
import org.neo4j.gds.api.schema.GraphSchema;
import org.neo4j.gds.collections.ha.HugeObjectArray;
import org.neo4j.gds.core.concurrency.Concurrency;
import org.neo4j.gds.core.utils.paged.HugeAtomicBitSet;
import org.neo4j.gds.core.utils.partition.Partition;
import org.neo4j.gds.core.utils.partition.PartitionUtils;
import org.neo4j.gds.core.utils.progress.tasks.ProgressTracker;
import org.neo4j.gds.termination.TerminationFlag;
import java.util.List;
import java.util.Optional;
import java.util.Set;
import java.util.SplittableRandom;
import java.util.function.Function;
import java.util.stream.Collectors;
import static org.neo4j.gds.utils.StringFormatting.formatWithLocale;
/**
* Based on the paper "Hashing-Accelerated Graph Neural Networks for Link Prediction"
*/
public class HashGNN extends Algorithm {
private static final long DEGREE_PARTITIONS_PER_THREAD = 4;
private final long randomSeed;
private final Graph graph;
private final SplittableRandom rng;
private final HashGNNParameters parameters;
private final Concurrency concurrency;
private final MutableLong currentTotalFeatureCount = new MutableLong();
public HashGNN(Graph graph, HashGNNParameters parameters, ProgressTracker progressTracker, TerminationFlag terminationFlag) {
super(progressTracker);
this.graph = graph;
this.parameters = parameters;
this.concurrency = parameters.concurrency();
long tempRandomSeed = this.parameters.randomSeed().orElse((new SplittableRandom().nextLong()));
this.randomSeed = new SplittableRandom(tempRandomSeed).nextLong();
this.rng = new SplittableRandom(randomSeed);
this.terminationFlag = terminationFlag;
}
@Override
public HashGNNResult compute() {
progressTracker.beginSubTask("HashGNN");
// Since degree only very approximately reflect the min hash task workload per node we decrease the partition sizes.
int decreasedConcurrency = Math.toIntExact(Math.min(concurrency.value() * DEGREE_PARTITIONS_PER_THREAD, graph.nodeCount()));
var degreePartition = PartitionUtils.degreePartition(
graph,
new Concurrency(decreasedConcurrency),
Function.identity(),
Optional.of(1)
);
var rangePartition = PartitionUtils.rangePartition(
concurrency,
graph.nodeCount(),
Function.identity(),
Optional.of(1)
);
Graph graphCopy = graph.concurrentCopy();
GraphSchema schema = graph.schema();
List graphs = parameters.heterogeneous()
? schema.relationshipSchema().availableTypes()
.stream()
.map(rt -> graph.relationshipTypeFilteredGraph(Set.of(rt)))
.collect(Collectors.toList())
: List.of(graphCopy);
var embeddingsB = constructInputEmbeddings(rangePartition);
int embeddingDimension = (int) embeddingsB.get(0).size();
double avgInputActiveFeatures = currentTotalFeatureCount.doubleValue() / graph.nodeCount();
progressTracker.logInfo(formatWithLocale(
"Density (number of active features) of binary input features is %.4f.",
avgInputActiveFeatures
));
var embeddingsA = HugeObjectArray.newArray(HugeAtomicBitSet.class, graph.nodeCount());
embeddingsA.setAll(unused -> HugeAtomicBitSet.create(embeddingDimension));
double avgDegree = graph.relationshipCount() / (double) graph.nodeCount();
double upperBoundNeighborExpectedBits = embeddingDimension == 0
? 1
: embeddingDimension * (1 - Math.pow(
1 - (1.0 / embeddingDimension),
avgDegree
));
progressTracker.beginSubTask("Propagate embeddings");
for (int iteration = 0; iteration < parameters.iterations(); iteration++) {
terminationFlag.assertRunning();
var currentEmbeddings = iteration % 2 == 0 ? embeddingsA : embeddingsB;
var previousEmbeddings = iteration % 2 == 0 ? embeddingsB : embeddingsA;
for (long i = 0; i < currentEmbeddings.size(); i++) {
currentEmbeddings.get(i).clear();
}
double scaledNeighborInfluence = graph.relationshipCount() == 0 ? 1.0 : (currentTotalFeatureCount.doubleValue() / graph.nodeCount()) * parameters.neighborInfluence() / upperBoundNeighborExpectedBits;
currentTotalFeatureCount.setValue(0);
var hashes = HashTask.compute(
embeddingDimension,
scaledNeighborInfluence,
graphs.size(),
concurrency,
parameters.embeddingDensity(),
randomSeed + parameters.embeddingDensity() * iteration,
terminationFlag,
progressTracker
);
MinHashTask.compute(
degreePartition,
graphs,
concurrency,
parameters.embeddingDensity(),
embeddingDimension,
currentEmbeddings,
previousEmbeddings,
hashes,
progressTracker,
terminationFlag,
currentTotalFeatureCount
);
double avgActiveFeatures = currentTotalFeatureCount.doubleValue() / graph.nodeCount();
progressTracker.logInfo(formatWithLocale(
"After iteration %d average node embedding density (number of active features) is %.4f.",
iteration,
avgActiveFeatures
));
}
progressTracker.endSubTask("Propagate embeddings");
var binaryOutputVectors = (parameters.iterations() - 1) % 2 == 0 ? embeddingsA : embeddingsB;
NodePropertyValues outputVectors = parameters.outputDimension().map(it -> {
var denseVectors = DensifyTask.compute(
graph,
rangePartition,
concurrency,
it,
rng,
binaryOutputVectors,
progressTracker,
terminationFlag
);
return (NodePropertyValues) EmbeddingsToNodePropertyValues.fromDense(denseVectors);
}).orElseGet(() -> EmbeddingsToNodePropertyValues.fromBinary(binaryOutputVectors, embeddingDimension));
progressTracker.endSubTask("HashGNN");
return new HashGNNResult(outputVectors);
}
private HugeObjectArray constructInputEmbeddings(List partition) {
// User input parsing proves that if FeatureProperties is empty
// then GenerateFeatures is not
if (parameters.featureProperties().isEmpty()) {
return GenerateFeaturesTask.compute(
parameters.generateFeatures().get(),
graph,
partition,
concurrency,
randomSeed,
progressTracker,
terminationFlag,
currentTotalFeatureCount
);
}
return parameters.binarizeFeatures().map(it ->
BinarizeTask.compute(
graph,
partition,
concurrency,
parameters.featureProperties(),
it,
rng,
progressTracker,
terminationFlag,
currentTotalFeatureCount
)
).orElseGet(() ->
RawFeaturesTask.compute(
concurrency,
parameters.featureProperties(),
progressTracker,
graph,
partition,
terminationFlag,
currentTotalFeatureCount
)
);
}
static final class MinAndArgmin {
public int min;
public int argMin;
MinAndArgmin() {
this.min = -1;
this.argMin = Integer.MAX_VALUE;
}
}
}
© 2015 - 2025 Weber Informatics LLC | Privacy Policy