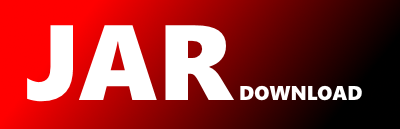
org.neo4j.gds.leiden.RefinementPhase Maven / Gradle / Ivy
Go to download
Show more of this group Show more artifacts with this name
Show all versions of algo Show documentation
Show all versions of algo Show documentation
Neo4j Graph Data Science :: Algorithms
The newest version!
/*
* Copyright (c) "Neo4j"
* Neo4j Sweden AB [http://neo4j.com]
*
* This file is part of Neo4j.
*
* Neo4j is free software: you can redistribute it and/or modify
* it under the terms of the GNU General Public License as published by
* the Free Software Foundation, either version 3 of the License, or
* (at your option) any later version.
*
* This program is distributed in the hope that it will be useful,
* but WITHOUT ANY WARRANTY; without even the implied warranty of
* MERCHANTABILITY or FITNESS FOR A PARTICULAR PURPOSE. See the
* GNU General Public License for more details.
*
* You should have received a copy of the GNU General Public License
* along with this program. If not, see .
*/
package org.neo4j.gds.leiden;
import com.carrotsearch.hppc.BitSet;
import org.apache.commons.lang3.mutable.MutableLong;
import org.neo4j.gds.api.Graph;
import org.neo4j.gds.core.concurrency.Concurrency;
import org.neo4j.gds.core.concurrency.RunWithConcurrency;
import org.neo4j.gds.mem.MemoryEstimation;
import org.neo4j.gds.mem.MemoryEstimations;
import org.neo4j.gds.collections.ha.HugeDoubleArray;
import org.neo4j.gds.collections.ha.HugeLongArray;
import org.neo4j.gds.core.utils.partition.PartitionUtils;
import org.neo4j.gds.core.utils.progress.tasks.ProgressTracker;
import org.neo4j.gds.mem.Estimate;
import java.util.List;
import java.util.Optional;
import java.util.Random;
import java.util.concurrent.ExecutorService;
final class RefinementPhase {
private final Graph workingGraph;
private final HugeLongArray originalCommunities;
private final HugeDoubleArray nodeVolumes;
private final HugeDoubleArray communityVolumes;
private final HugeDoubleArray communityVolumesAfterMerge;
private final double gamma;
private final double theta; // randomness
private final HugeDoubleArray relationshipsBetweenCommunities;
private final HugeLongArray encounteredCommunities;
private final HugeDoubleArray encounteredCommunitiesWeights;
private final long seed;
private long communityCounter = 0L;
private final Concurrency concurrency;
private final ExecutorService executorService;
private final HugeDoubleArray nextCommunityProbabilities;
private final ProgressTracker progressTracker;
static RefinementPhase create(
Graph workingGraph,
HugeLongArray originalCommunities,
HugeDoubleArray nodeVolumes,
HugeDoubleArray communityVolumes,
double gamma,
double theta,
long seed,
Concurrency concurrency,
ExecutorService executorService,
ProgressTracker progressTracker
) {
var encounteredCommunities = HugeLongArray.newArray(workingGraph.nodeCount());
var encounteredCommunitiesWeights = HugeDoubleArray.newArray(workingGraph.nodeCount());
encounteredCommunitiesWeights.setAll(c -> -1L);
HugeDoubleArray nextCommunityProbabilities = HugeDoubleArray.newArray(workingGraph.nodeCount());
return new RefinementPhase(
workingGraph,
originalCommunities,
nodeVolumes,
communityVolumes,
encounteredCommunities,
encounteredCommunitiesWeights,
nextCommunityProbabilities,
gamma,
theta,
seed,
concurrency,
executorService,
progressTracker
);
}
private RefinementPhase(
Graph workingGraph,
HugeLongArray originalCommunities,
HugeDoubleArray nodeVolumes,
HugeDoubleArray communityVolumes,
HugeLongArray encounteredCommunities,
HugeDoubleArray encounteredCommunitiesWeights,
HugeDoubleArray nextCommunityProbabilities,
double gamma,
double theta,
long seed,
Concurrency concurrency,
ExecutorService executorService,
ProgressTracker progressTracker
) {
this.workingGraph = workingGraph;
this.originalCommunities = originalCommunities;
this.nodeVolumes = nodeVolumes;
this.communityVolumesAfterMerge = nodeVolumes.copyOf(nodeVolumes.size());
this.communityVolumes = communityVolumes;
this.encounteredCommunities = encounteredCommunities;
this.encounteredCommunitiesWeights = encounteredCommunitiesWeights;
this.nextCommunityProbabilities = nextCommunityProbabilities;
this.gamma = gamma;
this.theta = theta;
this.seed = seed;
encounteredCommunitiesWeights.setAll(c -> -1L);
this.relationshipsBetweenCommunities = HugeDoubleArray.newArray(workingGraph.nodeCount());
this.concurrency = concurrency;
this.executorService = executorService;
this.progressTracker = progressTracker;
}
static MemoryEstimation memoryEstimation() {
return MemoryEstimations.builder(RefinementPhase.class)
.perNode("encountered communities", HugeLongArray::memoryEstimation)
.perNode("encountered community weights", HugeDoubleArray::memoryEstimation)
.perNode("next community probabilities", HugeDoubleArray::memoryEstimation)
.perNode("merged community volumes", HugeDoubleArray::memoryEstimation)
.perNode("relationships between communities", HugeDoubleArray::memoryEstimation)
.perNode("refined communities", HugeLongArray::memoryEstimation)
.perNode("merge tracking bitset", Estimate::sizeOfBitset)
.build();
}
RefinementPhaseResult run() {
var refinedCommunities = HugeLongArray.newArray(workingGraph.nodeCount());
refinedCommunities.setAll(nodeId -> nodeId); //singleton partition
computeRelationshipsBetweenCommunities();
BitSet singleton = new BitSet(workingGraph.nodeCount());
singleton.set(0, workingGraph.nodeCount());
var random = new Random(seed);
MutableLong maximumCommunityId = new MutableLong(-1);
workingGraph.forEachNode(nodeId -> {
boolean isSingleton = singleton.get(nodeId);
if (isSingleton && isWellConnected(nodeId)) {
mergeNodeSubset(nodeId, refinedCommunities, singleton, random);
}
var refinedId = refinedCommunities.get(nodeId);
if (maximumCommunityId.longValue() < refinedId) {
maximumCommunityId.setValue(refinedId);
}
progressTracker.logProgress();
return true;
});
// We don't use the `communityCount` from the RefinementPhase => set it to `-1` in case we try to read it by mistake.
return new RefinementPhaseResult(
refinedCommunities,
communityVolumesAfterMerge,
maximumCommunityId.longValue()
);
}
private void computeRelationshipsBetweenCommunities() {
List tasks = PartitionUtils.degreePartition(
workingGraph,
concurrency,
degreePartition -> new RefinementBetweenRelationshipCounter(
workingGraph.concurrentCopy(),
relationshipsBetweenCommunities,
originalCommunities,
degreePartition
),
Optional.empty()
);
RunWithConcurrency.builder().
concurrency(concurrency)
.tasks(tasks)
.executor(executorService).
run();
}
private void mergeNodeSubset(
long nodeId,
HugeLongArray refinedCommunities,
BitSet singleton,
Random random
) {
// Reset the community counter
communityCounter = 0;
computeCommunityInformation(nodeId, refinedCommunities);
var currentNodeCommunityId = refinedCommunities.get(nodeId);
var currentNodeVolume = nodeVolumes.get(nodeId);
long i = 0;
double probabilitiesSum = 0d;
if (communityCounter == 0)
return;
double bestGain = 0d;
long bestCommunityId = 0;
double totalSumOfRelationships = 0.0;
for (long c = 0; c < communityCounter; c++) {
var candidateCommunityId = encounteredCommunities.get(c);
var communityRelationshipsCount = encounteredCommunitiesWeights.get(candidateCommunityId);
totalSumOfRelationships += communityRelationshipsCount;
encounteredCommunitiesWeights.set(candidateCommunityId, -communityRelationshipsCount);
var modularityGain =
communityRelationshipsCount - currentNodeVolume * communityVolumesAfterMerge.get(candidateCommunityId) * gamma;
if (modularityGain > bestGain) {
bestGain = modularityGain;
bestCommunityId = candidateCommunityId;
}
double nextCommunityProbability = 0d;
if (modularityGain >= 0) {
nextCommunityProbability = Math.exp(modularityGain / theta);
}
nextCommunityProbabilities.set(i++, nextCommunityProbability);
probabilitiesSum += nextCommunityProbability;
}
long nextCommunityId = currentNodeCommunityId;
if (Double.isInfinite(probabilitiesSum) || probabilitiesSum <= 0) {
if (bestGain > 0) {
nextCommunityId = bestCommunityId;
}
} else {
nextCommunityId = selectRandomCommunity(
nextCommunityProbabilities,
probabilitiesSum,
random,
nextCommunityId
);
}
if (nextCommunityId != currentNodeCommunityId) {
addToCommunity(
nodeId,
refinedCommunities,
singleton,
currentNodeCommunityId,
totalSumOfRelationships,
nextCommunityId
);
}
}
private long selectRandomCommunity(
HugeDoubleArray nextCommunityProbabilities,
double probabilitiesSum,
Random random,
long defaultCommunity
) {
var x = probabilitiesSum * random.nextDouble();
assert x >= 0;
long nextCommunityId = defaultCommunity;
long j = 0;
double curr = 0d;
for (long c = 0; c < communityCounter; c++) {
var candidateCommunityId = encounteredCommunities.get(c);
var candidateCommunityProbability = nextCommunityProbabilities.get(j);
curr += candidateCommunityProbability;
if (x <= curr) {
nextCommunityId = candidateCommunityId;
break;
}
j++;
}
return nextCommunityId;
}
private void addToCommunity(
long nodeId,
HugeLongArray refinedCommunities,
BitSet singleton,
long currentNodeCommunityId,
double totalSumOfRelationships,
long nextCommunityId
) {
refinedCommunities.set(nodeId, nextCommunityId);
if (singleton.get(nextCommunityId)) {
singleton.flip(nextCommunityId);
}
var nodeVolume = nodeVolumes.get(nodeId);
communityVolumesAfterMerge.addTo(nextCommunityId, nodeVolume);
communityVolumesAfterMerge.addTo(currentNodeCommunityId, -nodeVolume);
final long updatedCommunityId = nextCommunityId;
double externalEdgesWithNewCommunity = Math.abs(encounteredCommunitiesWeights.get(updatedCommunityId));
relationshipsBetweenCommunities.addTo(
updatedCommunityId,
totalSumOfRelationships - externalEdgesWithNewCommunity
);
}
private void computeCommunityInformation(
long nodeId,
HugeLongArray refinedCommunities
) {
long originalCommunityId = originalCommunities.get(nodeId);
workingGraph.forEachRelationship(nodeId, 1.0, (s, t, relationshipWeight) -> {
long tOriginalCommunity = originalCommunities.get(t);
if (tOriginalCommunity == originalCommunityId) { //they are in the same original partition
long tCommunity = refinedCommunities.get(t);
boolean candidateCommunityIsWellConnected = isWellConnected(tCommunity);
if (candidateCommunityIsWellConnected) {
if (encounteredCommunitiesWeights.get(tCommunity) < 0) {
encounteredCommunities.set(communityCounter, tCommunity);
communityCounter++;
encounteredCommunitiesWeights.set(tCommunity, relationshipWeight);
} else {
encounteredCommunitiesWeights.addTo(tCommunity, relationshipWeight);
}
}
}
return true;
});
}
private boolean isWellConnected(
long nodeOrCommunityId
) {
long originalCommunityId = originalCommunities.get(nodeOrCommunityId);
double originalCommunityVolume = communityVolumes.get(originalCommunityId);
double updatedCommunityVolume = communityVolumesAfterMerge.get(nodeOrCommunityId);
double rightSide = gamma * updatedCommunityVolume * (originalCommunityVolume - updatedCommunityVolume);
return relationshipsBetweenCommunities.get(nodeOrCommunityId) >= rightSide;
}
static class RefinementPhaseResult {
private final HugeLongArray communities;
private final HugeDoubleArray communityVolumes;
private final long maximumRefinementCommunityId;
RefinementPhaseResult(
HugeLongArray communities,
HugeDoubleArray communityVolumes,
long maximumRefinedCommunityId
) {
this.communities = communities;
this.communityVolumes = communityVolumes;
this.maximumRefinementCommunityId = maximumRefinedCommunityId;
}
HugeLongArray communities() {
return communities;
}
HugeDoubleArray communityVolumes() {
return communityVolumes;
}
long maximumRefinedCommunityId() {return maximumRefinementCommunityId;}
}
}
© 2015 - 2025 Weber Informatics LLC | Privacy Policy