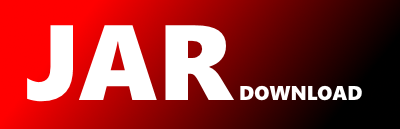
org.neo4j.gds.similarity.knn.metrics.Pearson Maven / Gradle / Ivy
Go to download
Show more of this group Show more artifacts with this name
Show all versions of algo Show documentation
Show all versions of algo Show documentation
Neo4j Graph Data Science :: Algorithms
The newest version!
/*
* Copyright (c) "Neo4j"
* Neo4j Sweden AB [http://neo4j.com]
*
* This file is part of Neo4j.
*
* Neo4j is free software: you can redistribute it and/or modify
* it under the terms of the GNU General Public License as published by
* the Free Software Foundation, either version 3 of the License, or
* (at your option) any later version.
*
* This program is distributed in the hope that it will be useful,
* but WITHOUT ANY WARRANTY; without even the implied warranty of
* MERCHANTABILITY or FITNESS FOR A PARTICULAR PURPOSE. See the
* GNU General Public License for more details.
*
* You should have received a copy of the GNU General Public License
* along with this program. If not, see .
*/
package org.neo4j.gds.similarity.knn.metrics;
/**
* Here we compute Pearson correlation coefficient and turn that into a metric.
*
* We use the formula from https://en.wikipedia.org/wiki/Pearson_correlation_coefficient#For_a_sample for the Pearson
* computation.
*
* If input arrays are of different length we ignore the longer tail.
*
* In the end we turn Pearson's r into a metric moving it to the range 0..1
*/
public final class Pearson {
private Pearson() {}
public static double floatMetric(float[] a, float[] b) {
int n = Math.min(a.length, b.length);
// compute sample means
double sumA = 0d;
double sumB = 0d;
for (int i = 0; i < n; i++) {
sumA += a[i];
sumB += b[i];
}
double meanA = sumA / n;
double meanB = sumB / n;
// compute sums
double sumOfProductOfADeltaBDelta = 0d;
double sumOfADeltaSquared = 0d;
double sumOfBDeltaSquared = 0d;
for (int i = 0; i < n; i++) {
double aDelta = a[i] - meanA;
double bDelta = b[i] - meanB;
sumOfProductOfADeltaBDelta += aDelta * bDelta;
sumOfADeltaSquared += aDelta * aDelta;
sumOfBDeltaSquared += bDelta * bDelta;
}
// final formula
double r = sumOfProductOfADeltaBDelta/ (Math.sqrt(sumOfADeltaSquared * sumOfBDeltaSquared));
// now turn it into a metric; Pearson's r is in the range -1..1 and we want to land it in 0..1
return (r+1)/ 2;
}
public static double doubleMetric(double[] a, double[] b) {
int n = Math.min(a.length, b.length);
// compute sample means
double sumA = 0d;
double sumB = 0d;
for (int i = 0; i < n; i++) {
sumA += a[i];
sumB += b[i];
}
double meanA = sumA / n;
double meanB = sumB / n;
// compute sums
double sumOfProductOfADeltaBDelta = 0d;
double sumOfADeltaSquared = 0d;
double sumOfBDeltaSquared = 0d;
for (int i = 0; i < n; i++) {
double aDelta = a[i] - meanA;
double bDelta = b[i] - meanB;
sumOfProductOfADeltaBDelta += aDelta * bDelta;
sumOfADeltaSquared += aDelta * aDelta;
sumOfBDeltaSquared += bDelta * bDelta;
}
// final formula
double r = sumOfProductOfADeltaBDelta/ (Math.sqrt(sumOfADeltaSquared * sumOfBDeltaSquared));
// now turn it into a metric; Pearson's r is in the range -1..1 and we want to land it in 0..1
return (r+1)/ 2;
}
}
© 2015 - 2025 Weber Informatics LLC | Privacy Policy